AI Integration: Transforming Research Across Disciplines
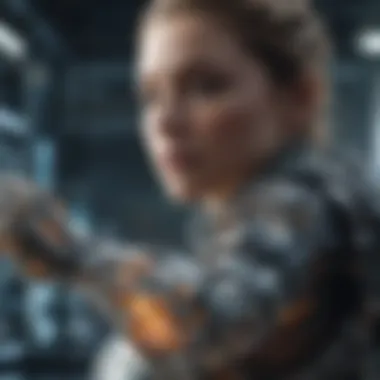
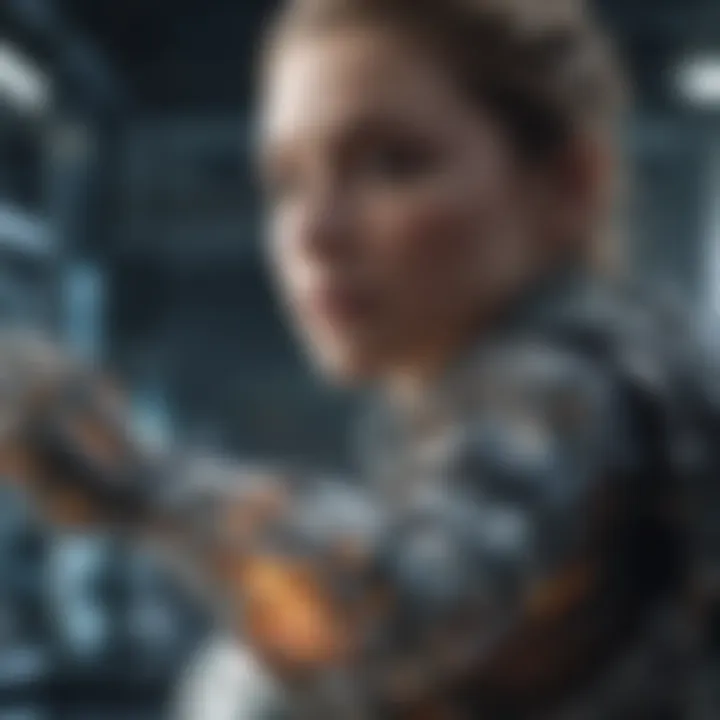
Intro
The increasing integration of artificial intelligence (AI) into scientific research is reshaping disciplines previously thought to be human domain. From biology to physics, the collaboration between AI technologies and traditional methodologies is not just incremental; it’s redefining the ways research is conducted and the very frameworks of inquiry. This transformation invites a closer look at the profound implications AI holds for scientific process, output, and the future direction of research.
As diverse fields begin to adapt and embrace AI solutions, the potential benefits in terms of efficiency and accuracy become evident. The present landscape of scientific research gives rise to questions—not solely about improved outcomes but also about the ethical ramifications and the implications for future studies.
Research Background
Overview of the Scientific Problem Addressed
Traditionally, scientific research has faced challenges that impede progress. Constraints such as time, computational limitations, and human error often result in slower advancements and less accurate findings. These barriers are sometimes marked by subjective interpretation and manually intensive processes, which limit reproducibility and scalability of results. Enter AI, with its capability for processing vast amounts of data at unprecedented speeds and accuracy.
Historical Context and Previous Studies
The seeds of AI in scientific research have been sown over decades. Early pioneers like Alan Turing paved the way for computational thinking, while more recent developments in machine learning and neural networks have broadened the landscape of possibilities. Studies have showcased AI's utility in areas such as genetics, where it has effectively predicted disease susceptibility; in chemistry, where it's accelerated drug discovery; and in earth sciences, where predictive algorithms model climate changes.
To understand the impact of AI on research today, one must reflect on these underpinnings. As the saying goes, "you've got to know where you've been to understand where you're going."
Findings and Discussion
Key Results of the Research
The integration of AI techniques into various scientific disciplines has returned tangible results. In biology, AI methods have improved the accuracy of genomic sequencing by refining the way data is analyzed, enabling researchers to uncover links between genes and diseases more swiftly.
In chemistry, AI has propelled innovation by enabling predictive modeling to identify potential drug candidates, effectively revolutionizing the pharmaceutical landscape. This technology not only accelerates the discovery process but also reduces resource consumption.
In physics, AI aids in interpreting complex data from particle colliders, providing insights that were, until now, painstakingly difficult to derive.
Interpretation of the Findings
These findings illuminate a clear trend: AI's role is not just auxiliary; it’s transformative. By minimizing human error, enhancing analysis, and facilitating collaboration across disciplines, AI is actively shaping the future of scientific inquiry. This technology empowers researchers to focus on creative problem-solving rather than being bogged down by data, paving the way for novel hypotheses and exploration methods.
"AI integration into scientific fields enables an unprecedented level of collaboration and efficiency, opening doors to innovation previously considered unattainable."
As we navigate this evolving landscape, it’s crucial to remain cognizant of ethical considerations and the societal implications these advancements carry. Researchers must ensure that AI serves as an augmentative tool, preserving integrity while embracing potential.
The ongoing dialogue about AI’s merit in research will only grow louder, underscoring the need for vigilance and responsibility in embracing these technologies into traditional frameworks.
The potential for AI in science is like a double-edged sword. It holds the promise of making us smarter, but at its core, it forces us to confront our own limitations and ethics in an increasingly complex world.
Prolusion to AI in Science
Artificial Intelligence (AI) has become a game-changer, especially in the scientific realm. It’s like tossing a coin into a wishing well; you hope for something valuable to come out, and with AI, the returns are immense. By integrating AI into various disciplines, researchers are finding new ways to tackle complex problems that once seemed insurmountable. This section unpacks the importance of AI in science, highlighting the specific elements, benefits, and considerations of this fascinating integration.
Defining AI Integration
When we talk about AI integration, we’re referring to the process of embedding artificial intelligence technologies into scientific research. This goes beyond just employing AI for data analysis; it's about weaving AI into the fabric of research methodologies. AI can assist in everything from predictive modeling to automating repetitive tasks, thus allowing researchers to focus on what they do best—innovating and experimenting.
It's not merely the technology that's transforming science, but how it augments human intellect and capabilities. For instance, in biology, machine learning algorithms sift through massive genomic databases, identifying patterns that a human might miss. This raises a vital question: how far can science go when supported by AI? Well, only time will tell, but the signs are promising.
Historical Context of AI in Research
To fully appreciate the role of AI in modern science, we should glance back at its historical roots. The journey of AI began in the mid-20th century, but its application in scientific research started gaining momentum in the 1980s. Back then, the computational power we take for granted today was still in its infancy. Nevertheless, pioneers were already exploring how to exploit algorithmic approaches to problem-solving in fields like chemistry and physics.
Fast forward to the early 2000s, a noted turning point emerged with the advent of big data. This era marked the need for advanced tools to analyze the volume and complexity of data generated across various scientific domains. Fast forward again to the 2010s and beyond, as deep learning and neural networks gained a foothold, pushing the boundaries of what AI can accomplish in research.
Thus, we find ourselves at a crossroads where historical context meets cutting-edge innovation. Understanding the past helps scientists and researchers to better navigate the ethical and technical challenges that arise today.
"The integration of AI in science not only accelerates the pace of discovery but also transforms the nature of scientific inquiry itself."
In summary, the integration of AI into scientific research is not just a trend; it's a profound shift. This section illustrates how AI facilitates a new paradigm of scientific inquiry, enabling researchers to unearth insights at an unprecedented scale and depth.
The Role of AI in Biology
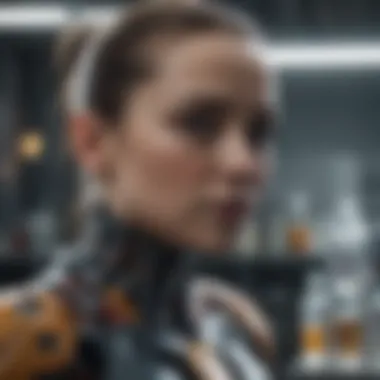
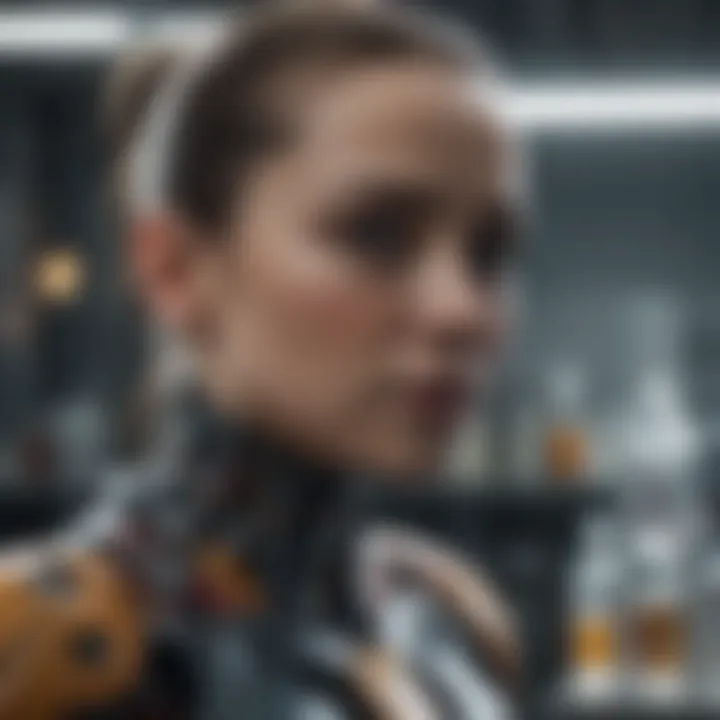
The integration of AI in biology has emerged as a revolutionary force, enhancing our capabilities to analyze complex biological systems and processes. This section will emphasize the profound impact AI technologies have on various biological disciplines, spotlighting the tools, methods, and the resultant benefits of adopting AI-driven approaches. As biological research continues to burgeon, so does the necessity for efficient, accurate data analysis and interpretation.
AI’s role transcends mere data processing; it reshapes the very fabric of scientific inquiry by unearthing patterns that might elude traditional analytical techniques. Some specific elements to consider are:
- Enhanced data processing speed, allowing for greater volumes of data to be scrutinized effectively.
- Improved accuracy in predictive modeling, potentially leading to more robust conclusions.
- Streamlined workflows that facilitate collaboration across diverse teams.
The ability of AI systems to learn from data and refine their algorithms means that they adapt over time, increasing their utility in biological research. As we delve into the subsections, we will explore Genomic Data Analysis, AI's role in Drug Discovery, and the application of Machine Learning Models in Ecology.
Genomic Data Analysis
Genomic data analysis stands as one of the most salient examples of how AI is changing biology. The sheer volume of genetic data generated through modern sequencing technologies can be staggering. Traditional methods of analysis struggle to keep pace, presenting a clear need for AI solutions.
One of the notable advantages lies in the ability of AI algorithms to identify significant genetic variants linked to diseases. For instance, utilizing deep learning techniques, researchers can predict the functional impact of genetic mutations, enhancing personalized medicine approaches.
Furthermore, AI-driven tools can automate the extraction of key insights from genomic data, reducing the margin for human error and ensuring a more streamlined analytical process. Studies show that when researchers applied AI in genomic analysis, they could achieve outcomes in a fraction of the time compared to conventional methods, enabling faster turnaround in medical diagnostics and treatments.
AI in Drug Discovery
The pharmaceutical industry has long been hampered by lengthy and expensive drug discovery processes. Integrating AI into this stage of research has the potential to turn the tides. AI can vastly improve target identification, predictive modeling of drug interactions, and optimize the design of clinical trials.
One exciting development is the use of generative models, which assist scientists in designing novel compounds that may have therapeutic benefits. They leverage existing molecular databases to discover new molecules with desired properties. This approach not only shortens the time required to develop new drugs but also increases the likelihood of success by focusing on the most promising candidates early in the research process.
AI can discover potential drug candidates that humans may overlook, further paving the way for breakthroughs in treatment options.
Machine Learning Models in Ecology
In the realm of ecology, machine learning has opened doors to understanding complex environmental patterns that govern ecosystems. By analyzing vast datasets from various sources, including satellite imagery and sensor data, AI models can forecast ecological changes, habitat loss, and even species migration patterns.
For instance, researchers employing machine learning algorithms can assess the impact of climate change on different species, helping conservationists prioritize efforts. The ability of these models to adapt and learn from new data allows for ongoing improvement in the analysis of ecological trends. This not only aids in proactive conservation strategies but also fosters a comprehensive understanding of ecological dynamics.
In summary, the application of AI in biology is transforming how researchers conduct experiments, analyze data, and draw conclusions. As technology evolves, so will the methods driving scientific discovery across biological disciplines.
AI Applications in Chemistry
Artificial intelligence is reshaping the field of chemistry in ways previously thought to be beyond reach. As chemists grapple with vast amounts of data produced by experiments, AI opens new doors to analyze, predict, and innovate. At its core, AI in chemistry leverages algorithms and computational power to tackle issues like reaction prediction, materials design, and chemical synthesis. The integration of AI not only enhances the efficacy of chemists but also paves the way for cutting-edge discoveries that could alter entire industries.
Predictive Modeling for Chemical Reactions
Predictive modeling stands as a cornerstone of AI applications in chemistry. It involves training machine learning algorithms on existing chemical data to predict the outcomes of chemical reactions. The known structure-properties relationship can be quite complex, but AI has a knack for finding hidden correlations that human researchers might overlook.
Consider this: traditional methods of predicting chemical reactions often involve lengthy trial-and-error approaches, consuming valuable time and resources. AI, however, accelerates this process. With tools like DeepMind’s AlphaFold, significant strides have been made in protein folding problems, underscoring the potential of predictive models not just in chemical reactions but biochemistry all the same.
"AI can analyze reaction pathways, optimize conditions, and even propose completely new reactions. This level of sophistication is revolutionizing how we approach chemistry."
Some notable benefits of employing AI in predictive modeling include:
- Efficiency: Reduces the time it takes to identify viable reaction pathways.
- Cost-effectiveness: Minimizes the need for extensive laboratory testing, leading to lower research expenses.
- Innovation: Aids in the discovery of novel compounds, thus expanding the chemical toolkit at researchers' disposal.
AI in Material Science
Material science is another significant frontier where AI applications are making waves. The development of new materials typically requires extensive research and experimentation. With AI, researchers can simulate various properties and behaviors of materials before actual experiments are conducted.
Machine learning can analyze databases of known materials to identify promising candidates with desired properties, which has profound implications in industries like electronics, energy, and nanotechnology. For instance, the use of AI in battery material development has led to the discovery of new battery chemistries that could significantly enhance energy storage capacities.
- Rapid Material Screening: AI sifts through thousands of materials swiftly, identifying those with optimal characteristics for specific applications.
- Tailored Material Design: With predictive models, it becomes possible to design materials with customized properties, meeting the demands of advanced technologies.
AI’s ability to innovate in material science exemplifies how technology can redefine processes and outcome prediction in chemistry. By facilitating a new level of accuracy and insight, it enables scientists to push boundaries that once seemed insurmountable.
Integrating AI in Physics Research
Integrating artificial intelligence in physics research is not just another trend; it represents a paradigm shift that can enhance how researchers approach complex problems and data. The intricacies of physics demand precision and depth, and AI offers tools that can analyze patterns, predict outcomes, and simulate scenarios much faster than traditional methods. This integration allows physicists to unravel underlying phenomena that were previously challenging to identify, ultimately pushing the frontiers of knowledge.
One significant aspect is the capacity for data analysis. In a field where experimental data can reach staggering volumes, AI can streamline the processing and interpretation of results—saving time and improving accuracy. For example, machine learning algorithms can sift through terabytes of data generated by particle accelerators far quicker than human effort can muster. This efficiency becomes increasingly crucial as experiments yield more data than any one researcher can adequately review, making AI an invaluable partner in the quest for understanding.
Data-Driven Physics Simulations
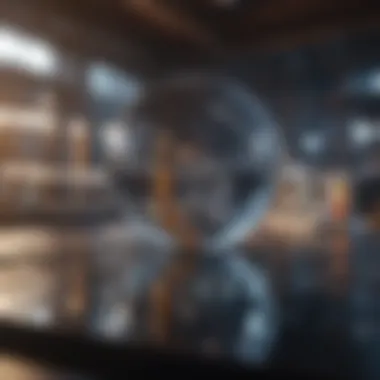
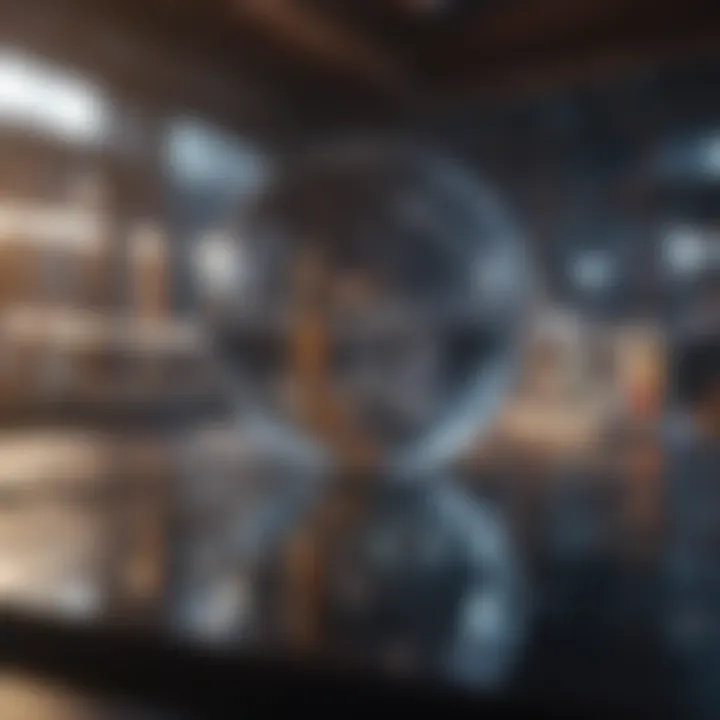
Physics is replete with intricate models to simulate natural phenomena, from climate change to quantum mechanics. The challenge has typically been the computational burden required to run these simulations. Here, AI changes the game. Techniques like neural networks can predict outcomes based on existing data, which reduces time-consuming calculations.
For instance, researchers have successfully used AI to create models of complex systems, applying them in areas such as fluid dynamics or quantum field theory. They train AI on historical data and leverage it to make predictions, facilitating innovative experiments that test theoretical predictions against real-world phenomena more efficiently. This process helps not only in managing the workload but also provides insights that steer researchers to new hypotheses and experimental designs.
In a recent study, scientists utilized AI to optimize simulations in astrophysics, enhancing their ability to predict cosmic events. Such discoveries would remain hidden in raw data if it weren't for the sharp eye of AI.
AI for Particle Physics Analysis
Particle physics, a field often overwhelmed by massive amounts of data from experiments like those conducted at CERN's Large Hadron Collider, stands to benefit immensely from AI integration. Each second, experiments generate approximately 100 petabytes of data—numerous processes yield events that could hint at new physics phenomena. In this sea of data, AI emerges as the beacon that can guide physicists through the chaos.
Applying AI in particle physics involves clustering algorithms that can identify events of interest, such as rare particle decay processes with precision. These algorithms can differentiate between signal and noise, which is crucial in determining whether an event is significant or merely a statistical fluke.
Furthermore, deep learning techniques allow researchers to sift through complex event signatures and classify particles with a high degree of fidelity. These capabilities not only bolster the search for new particles or interactions but also enhance cross-disciplinary collaboration as AI techniques and insights can be shared across various physics domains.
"The application of AI in particle physics analysis embodies a blend of scientific inquiry and computational prowess, making the previously insurmountable now achievable."
Through the synergistic blend of AI with physics, the potential for groundbreaking discoveries only begins to unfold. As the field continues to advance, integrating AI in these complex studies promises not just to expedite research but to generate novel insights that challenge our understanding of the universe.
AI’s Influence on Earth Sciences
The intersection of artificial intelligence and earth sciences carries significant weight in contemporary research paradigms. AI's influence permeates climate science, geology, and environmental monitoring, serving as a vital tool to delve deeper into complex datasets that traditional methods may overlook. As the world grapples with pressing environmental challenges, integrating AI into earth sciences promises enhanced predictive capabilities and insights.
Climate Modeling and AI
With climate change at the forefront of global discussions, the application of AI in climate modeling presents a clarion call for more accurate forecasting. Historically, climate models relied heavily on assumptions, requiring extensive time and resources for validation. Enter AI: this technology enables researchers to harness vast amounts of data collected from various sources, like satellite imagery, ground-level sensors, and historical weather patterns.
AI techniques facilitate both short- and long-term predictions, addressing uncertainty in climate projections. For instance, machine learning algorithms can identify intricate patterns in climate data, allowing scientists to model potential outcomes of various greenhouse gas emission scenarios.
Key benefits of AI in climate modeling include:
- Enhanced Accuracy: AI improves the precision of models by recognizing subtle correlations and anomalies often missed by human analysts.
- Increased Speed: Complex simulations that typically take weeks can be processed in hours, empowering timely decision-making.
- Adaptability: As new data streams flood in, AI models can be quickly refined, ensuring they remain current and relevant.
"By applying AI, we can sift through terabytes of climate data in a fraction of the time, leading to more rapid solutions to pressing environmental issues."
Remote Sensing Technologies
Remote sensing sits prominently within the toolkit of earth sciences, and AI is enhancing this field to unprecedented levels. Remote sensing technologies use satellites and aerial vehicles to collect data about the Earth’s surface. Integrating AI into this mix transforms the raw data into actionable insights.
For instance, AI algorithms can automatically analyze satellite imagery to monitor deforestation rates, assess crop health, or track urban development. The automation of this analysis reduces the manual labor traditionally involved and increases the frequency of observations, leading to better-informed environmental policies.
Some critical advantages of employing AI in remote sensing include:
- Real-Time Data Analysis: AI processes data streams live, providing immediate feedback for stakeholders to make timely actions.
- Scalability: AI systems can facilitate analysis over large geographical areas without the additional manpower, making studies more extensive and extensive.
- Precision: Using advanced pattern recognition, AI can distinguish subtle changes in land use or ecosystem health that might escape conventional visual inspections.
In summary, AI's impact on earth sciences is reshaping our understanding of complex environmental systems. From refining climate models to enhancing remote sensing capabilities, the integration of AI presents enormous potential to forge effective responses to the planet’s challenges.
Ethical Considerations in AI Integration
Integrating Artificial Intelligence into scientific research is a profound step that offers numerous benefits and challenges. Understanding the ethical dimensions of this integration is crucial, as it lays the foundation for responsible use and helps safeguard the integrity of scientific inquiry. In this section, we will explore the salient aspects of ethics in AI integration, focusing on data privacy, security, and bias in algorithms, while also discussing how these elements impact the broader landscape of research.
Data Privacy and Security
When AI technologies process vast amounts of research data, concerns about data privacy become paramount. Researchers often deal with sensitive information, particularly in fields like genomics where personal genetic data must be handled with care. It raises the question: how can institutions safeguard this sensitive data when deploying AI?
To address data privacy and security, several guidelines and protocols have emerged. There’s a growing emphasis on implementing robust encryption methods, as well as access controls to ensure that only authorized personnel can view or manipulate sensitive data. Moreover, it’s beneficial to adopt a framework that not only complies with legal standards like GDPR but also promotes ethical principles in data stewardship.
- Establish clear data governance policies.
- Implement secure data storage solutions.
- Ensure transparency in data use and allow individuals to control their data.
Ultimately, prioritizing data privacy will encourage public trust in AI initiatives, fostering a collaborative environment within the scientific community.
"Ensuring data privacy is not just a regulatory requirement; it is a fundamental ethical responsibility that scientists bear when integrating AI into their research."
Bias in AI Algorithms
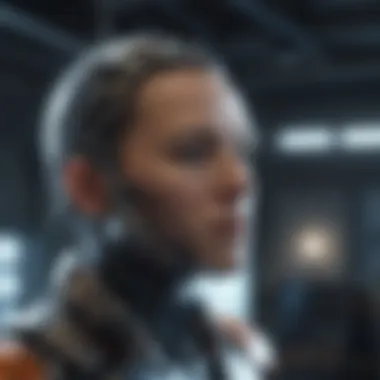
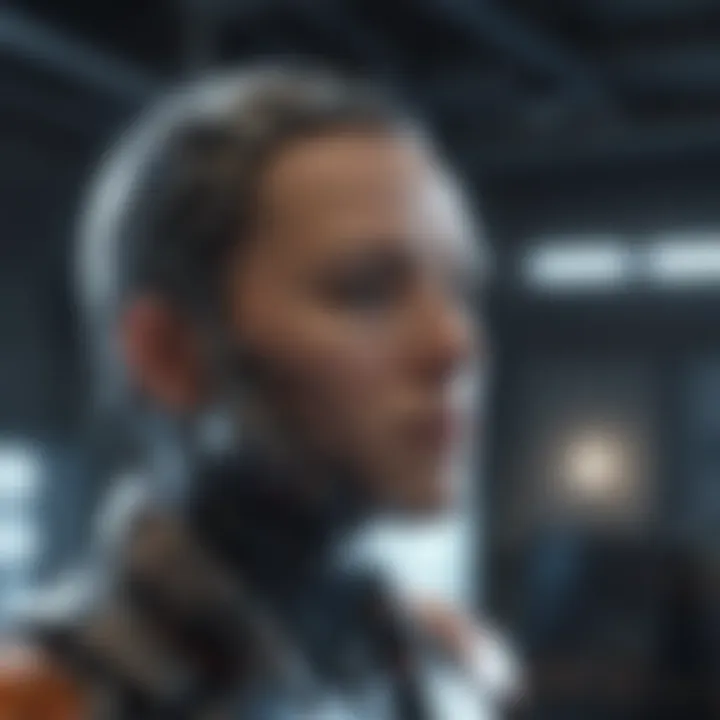
AI algorithms learn from historical data, and if this data carries bias, it can lead to skewed results, which can adversely affect research outcomes. For instance, if a dataset used in training an AI model is predominantly representative of one demographic group, any conclusions drawn may not accurately reflect broader populations. This is particularly concerning in fields like health research where decisions based on biased data can have dire consequences.
The ethical implications of biased algorithms are enormous, as they can perpetuate inequalities and reinforce existing disparities. To counteract this problem, researchers need to be aware of the potential for bias at every step of the AI development process:
- Diverse Data Collection: Focus on gathering a wide-ranging dataset that adequately represents all relevant groups.
- Regular Evaluation: Continuously assess models for biased outputs and refine processes refine as needed.
- Transparency in Algorithms: Develop mechanisms that clarify how decisions are made by AI systems, allowing researchers to identify potential biases.
By acknowledging and addressing bias in AI algorithms, the scientific community can ensure that AI integration not only enhances research but does so in a way that is equitable and just.
Challenges of Integrating AI into Research
As researchers delve deeper into the myriad ways AI can enhance scientific inquiry, it becomes increasingly clear that several hurdles accompany this integration. These challenges are not merely obstacles; they define the landscape in which AI interfaces with traditional scientific methodologies. Understanding these elements is crucial, as they can shape both the future applications of AI and the realization of its full potential in research.
Interdisciplinary Collaboration Needs
The first challenge in the integration of AI within research domains is fostering effective interdisciplinary collaboration. Scientific exploration traditionally relies on singular disciplines; however, harnessing AI necessitates the collaboration of experts across various fields. For instance, a biologist working on genomics must often partner with a data scientist proficient in machine learning. This blending of experts can yield fruitful results, but it is not without its difficulties.
- Communication Barriers: Specialists from different disciplines might possess distinct terminologies and methodologies. For example, indeed, a physicist's approach to a problem can vastly differ from that of a chemist. Such differences can lead to misunderstandings or challenges in conveying ideas and results.
- Cultural Issues: The differing cultures of scientific disciplines can also create friction. In fields like biology, empirical observation might take precedence over theoretical models, while in physics, calculations may dominate. Bridging these gaps is essential for seamless integration and can be quite the feat.
Besides overcoming these barriers, there’s also a need for shared platforms where knowledge exchange can occur. Collaborative projects like the Human Cell Atlas initiative exemplify this, where biologists and data scientists come together, leveraging AI to map every cell type in the human body.
Technical Barriers and Solutions
On the technical front, the integration of AI technology into research methodologies introduces a series of technical barriers. Researchers may face several issues such as inadequate infrastructure, lack of access to robust datasets, and the steep learning curve associated with new tools. Each of these challenges can impede progress, but solutions do exist.
- Infrastructure Limitations: Not all institutions have top-tier computational resources. This disparity can hinder those in less funded organizations, preventing them from utilizing advanced AI technologies. Solutions might include cloud computing resources which provide access to vast computational power.
- Data Access and Quality: High-quality data is a cornerstone of effective AI applications. However, access to extensive datasets is often restricted. Collaborations between institutions and open data initiatives may offer pathways to mitigate this problem. For example, initiatives like Open Data Program foster transparency and accessibility.
- Upskilling Researchers: The adoption of AI tools requires a certain level of expertise. Thus, training and professional development programs focusing on data science and machine learning are essential. Institutions should consider implementing short courses or workshops that aid researchers in acquiring these necessary skills.
The successful navigation of these technical challenges requires a cooperative approach involving industry leaders, researchers, and educational establishments. By addressing gaps collaboratively, the scientific community can better harness the capabilities of AI, propelling research into new horizons.
"To truly advance scientific inquiry with AI, we cannot overlook the vital role of collaboration and robust technical frameworks. Without these, even the most promising technologies can fall flat."
Future Directions of AI in Scientific Research
The convergence of artificial intelligence and scientific research is at the brink of a new frontier. With the relentless pace of technological advancements, the future is ripe with possibilities. Highlighting the significance of this topic reveals a landscape rich with potential, where AI can catalyze groundbreaking methodologies and elevate research across disciplines, from biology to physics. This section outlines emerging technologies and explores how AI may drive innovation in scientific investigations.
Emerging Technologies
In the evolution of AI within scientific research, several emerging technologies stand poised to make a significant impact. Some notable advancements include:
- Quantum Computing: This technology could revolutionize data processing speeds, making it possible to analyze vast datasets almost instantaneously. Scientists could manage complex simulations that current technology struggles with, paving the way for discoveries that seemed out of reach.
- Natural Language Processing: This area of AI focuses on the interaction between computers and humans through natural language. In research, it can help automate literature reviews, making it easier to synthesize vast amounts of research articles and identify relevant studies without manual filtering.
- Robotics: With AI-powered robotic systems, researchers can automate experimental processes. For instance, in labs where high-throughput screening is essential, these robots can perform experiments far quicker than human hands.
The fusion of these sophisticated technologies with scientific research can lead to unprecedented precision and efficiency. It also prompts researchers to rethink traditional practices in light of new possibilities.
Potential for Innovation Across Disciplines
The innovation instigated by AI is not confined to a single field; instead, it echoes through multiple disciplines, creating a ripple effect of transformative potential. Understanding this potential is crucial:
- Cross-Disciplinary Collaborations: AI can bridge gaps between different fields, facilitating collaboration that accelerates discovery. For example, bioinformatics merges biology and computer science, allowing for the analysis of genetic sequences using AI techniques.
- Enhanced Predictive Models: By integrating AI algorithms, researchers in fields such as meteorology can improve their predictive models, leading to more accurate climate forecasts. This not only serves science but aids in policy-making that responds to environmental changes.
- Personalized Solutions: In medicine, AI enables researchers to create personalized treatment plans by analyzing individual genetic data. This shift from a one-size-fits-all approach to tailored therapies can dramatically impact patient outcomes.
AI's integration across scientific fields may redefine what is possible, enhancing both the scope and depth of research initiatives.
The future of AI in science is not merely about automation or efficiency; it's about unlocking the potential of human creativity and intellect in ways previously unimagined. By fully embracing these emerging technologies and the innovation they drive, scientists can advance their fields to levels that may seem like the stuff of science fiction today.
Finale
The integration of artificial intelligence in scientific research is not just a fleeting trend, but a transformative shift that redefines the ways various disciplines are approached. As we have explored throughout the article, AI offers numerous advantages that enhance the efficiency and accuracy of research across biology, chemistry, physics, and earth sciences. This conclusion aims to distill the key takeaways from our discussion, emphasizing the immense potential AI holds for scientific practice.
One of the primary benefits of AI integration is the enhancement of data analysis capabilities. Traditional methods often struggle to keep up with the sheer volume of data being generated today. AI, with its ability to sift through large datasets quickly and identify patterns, equips researchers with the tools necessary to make sense of complex information with unprecedented speed.
Additionally, the article highlighted how collaborative innovation can flourish through AI applications. By breaking down silos between disciplines, AI encourages interdisciplinary teams to work towards common goals. This synergy paves the way for revolutionary discoveries that might otherwise remain undiscovered within the confines of each discipline.
"AI serves as a bridge, connecting the expertise of various fields and fostering a spirit of cooperative exploration."
However, it's essential to navigate the complexities that come with this technology. Ethical considerations, such as data privacy and algorithmic bias, are paramount. These issues call for a collective effort from researchers, tech developers, and policymakers to ensure that the benefits of AI are harnessed responsibly. Understanding the ramifications of AI on society and scientific integrity must be a priority moving forward.
As we look to the future, one can't help but feel a sense of anticipation regarding the next breakthroughs that AI integration will usher in. The potential seems boundless, not just for enhancing research methodologies but also for transforming the very fabric of how scientific inquiry is conducted.
Recap of Key Insights
- Enhanced Data Analysis: AI provides tools for rapid data evaluation, leading to quicker and more accurate research outcomes.
- Collaborative Innovation: AI fosters collaboration by bridging the gaps between different scientific fields, resulting in new discoveries.
- Ethical Challenges: Alongside its benefits, AI brings challenges such as bias in algorithms and privacy issues that need careful attention.
- Future Potential: The continued integration of AI holds promising opportunities that could redefine scientific research, emphasizing the necessity for ongoing dialogue about its implications.
In summary, the integration of AI within scientific research is both a current reality and a promising frontier. The insights gleaned from this exploration provide a foundation for educators, researchers, and students to engage thoughtfully with a rapidly evolving technological landscape.