Exploring Graphs for Air Pollution Insights
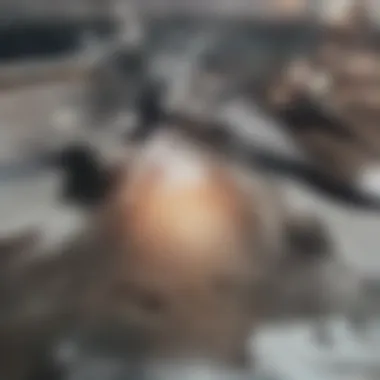
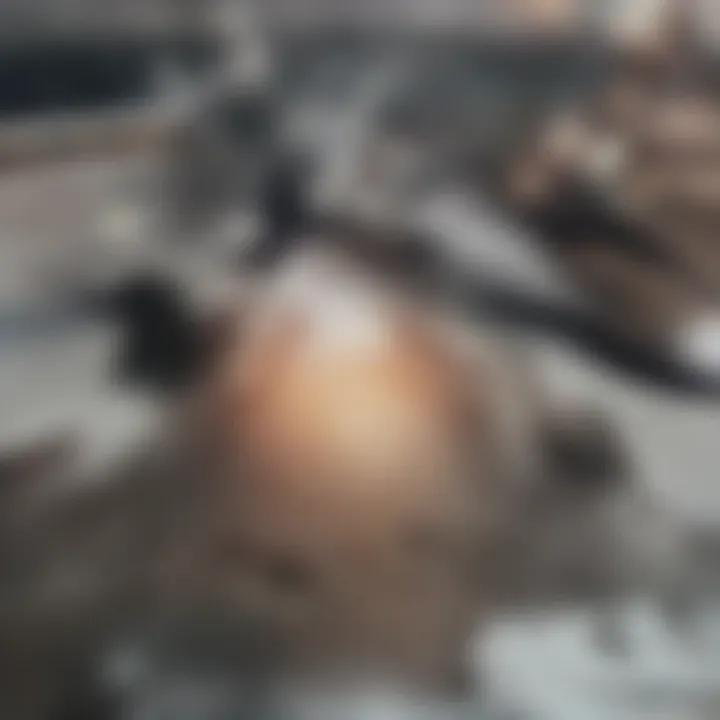
Intro
Understanding air pollution is vital for health, environment, and quality of life. Visual representations, like graphs, play a key role in conveying complex data. They simplify trends, highlight pollutants, and illustrate effects over time. This article examines these aspects in detail.
Graphs serve as an essential tool in scientific research. They facilitate the interpretation of large datasets and showcase relevant information in a clear and meaningful way. Comprehending air quality data through these visualizations is crucial for researchers, policymakers, and the general public. Each graph tells a story. The shapes and colors help to communicate essential aspects of air quality trends, making it easier for audiences to engage critically with the data.
In the sections that follow, we will explore the relationship between different pollutants and their effects. We will look at various graphing methodologies used to represent air pollution data. Moreover, we will dive into the historical context behind these studies and their implications for public policy. Our goal is not only to inform but to encourage deeper analysis and understanding of air quality issues.
Preamble to Air Pollution
Understanding air pollution is essential for comprehending its effects on human health and the environment. This section will clarify what air pollution is, its various sources, and its impacts. By grasping these elements, researchers and the public alike can appreciate the urgency of addressing air quality issues. Moreover, defining air pollution lays a foundation for analyzing data effectively through graphs.
Defining Air Pollution
Air pollution refers to the presence of harmful substances in the atmosphere. These substances can be gases, particulates, or biological molecules. Common air pollutants include carbon monoxide, sulfur dioxide, nitrogen dioxide, and particulate matter. The classification of air pollution encompasses both outdoor and indoor pollution.
Outdoor air pollution arises from emissions from vehicles, industries, and natural sources like wildfires. Indoor air pollution often results from household products, fuels used for cooking, or smoking. The complexities of air pollution necessitate clear definitions to promote better monitoring and management.
Sources of Air Pollution
Identifying the sources of air pollution is crucial to developing effective solutions. The primary sources can be categorized into several groups:
- Transportation: Vehicles emit exhaust fumes that contribute to urban air quality issues.
- Industry: Factories release pollutants during manufacturing processes, impacting both local and regional air quality.
- Agriculture: Agricultural activities, including the use of fertilizers and pesticides, lead to emissions of ammonia and other harmful substances.
- Household: Products used in homes, from cleaning agents to paints, can release volatile organic compounds into the air.
Addressing these diverse sources requires targeted policies that are informed by accurate data and effective graphical representations.
Impact on Human Health and Environment
The consequences of air pollution are profound. Poor air quality has been linked to numerous health issues, including respiratory illnesses, cardiovascular diseases, and even premature death. Moreover, certain pollutants can exacerbate asthma and negatively affect lung development in children.
The environment suffers as well. Air pollution can lead to acid rain, which impacts ecosystems, damage vegetation, and disrupt animal habitats. The cumulative effects of air pollution extend beyond immediate health risks, creating long-term challenges for both human and environmental health. Understanding these impacts is essential for motivating action across various sectors.
Importance of Graphs in Understanding Air Pollution
Graphs play a crucial role in decoding the complexities inherent in air pollution data. The significance of graphs in this context cannot be overstated. They serve as vital tools that convert raw, often overwhelming amounts of data into visual formats, making it easier for researchers, policymakers, and the general public to comprehend the state of air quality and its implications.
One of the core benefits of graphs is their ability to present intricate data sets in a more digestible form. This aids in identifying patterns, trends, and anomalies that may not be readily apparent in numerical data alone. For example, air quality indices can fluctuate with time, and graphs enable a clear visualization of these changes, showcasing peaks of pollution that might correlate with specific events or seasonal variations.
Moreover, graphs foster effective communication. They bridge the gap between scientific findings and public knowledge. Clear visual data representation is essential for encouraging public discourse around air quality issues, as many members of the community may lack a technical background. The interpretation of graphical data transcends language barriers, making it universally accessible. When air pollution data is presented graphically, it becomes a compelling narrative that can spur action among concerned citizens and stakeholders alike.
It is important, though, to note that the type of graph selected can significantly affect the message conveyed. Choosing the right visualization is integral to avoid misleading interpretations. Therefore, critical considerations should include what data is being represented, the intended audience, and the context in which the data will be used.
"Graphs are not just pictures; they’re a synthesis of information that allows better understanding of air quality issues and informs decision-making processes."
Visualizing Complex Data
The capacity of graphs to visualize complex data is transformative. Air pollution data often comes from multiple sources—monitoring stations, satellite observations, and environmental models. Each source provides different dimensions of information. By efficiently integrating these various data points, graphs can reveal multifaceted insights into pollution patterns, types, and concentrations across different regions and times.
This visualization is especially important in longitudinal studies, where changes over time can be tracked. Line graphs, for instance, can clearly show how pollutant levels have fluctuated over years, providing context to policy changes or natural events that have influenced these levels.
Furthermore, heat maps can illustrate spatial variations in air quality across geographical areas, highlighting pollution hotspots. This not only aids in identifying areas of concern but also directs resources and attention where it is most needed.
Enhancing Public Awareness
Enhancing public awareness is another critical aspect of using graphs in air pollution discourse. With the prevalence of air pollution affecting health on multiple levels, from respiratory issues to cardiovascular diseases, understanding its implications is paramount. Graphs empower individuals by providing them with a visual representation of air quality in their areas.
Utilizing simple and clear graphical formats allows for better engagement with the community. For instance, pie charts can effectively depict the proportions of different air pollutants in a specific region, giving citizens insight into the diversity of pollutants they might be exposed to. Regularly updated graphs can also keep the public informed about changes in air quality, fostering a sense of urgency and encouraging proactive behaviors like reducing emissions or advocating for policy changes.
Moreover, social media platforms such as Facebook have become a mainstream avenue for sharing these visualizations. By distributing compelling graphical representations of air pollution data, organizations can spark discussions and mobilize communities around pressing environmental issues.
Types of Graphs Used in Air Pollution Studies
Graphs serve as a crucial tool in understanding air pollution data. They provide contrast, clarity, and straightforward comparison that raw numbers lack. In the realm of air quality studies, the effective visual representation of data can lead to better insight and effective decision making. Each type of graph has its unique advantages and contexts where it thrives, making them suitable for portraying specific elements of air pollution.
Bar Graphs
Bar graphs are a common choice when comparing different categories of data. In air pollution studies, they can display a variety of pollutants across multiple sources or locations, allowing for easy visual comparisons. For example, a bar graph could show levels of particulate matter from different cities, enabling stakeholders to see which areas may be more severely affected. Each bar represents a distinct value, making it intuitive to identify trends, peaks, and variations.
Some benefits of bar graphs include:
- Clarity: Easy to read and understand
- Comparison: Facilitates direct comparison between variables
- Simplicity: Ideal for presenting a limited set of data points
However, bar graphs can oversimplify complex data, leading to a potential lack of nuance in conclusions drawn.
Line Graphs
Line graphs are useful for showing changes over time, making them ideal for tracking air quality trends. For instance, a line graph could illustrate the fluctuation of nitrogen dioxide levels over several years in a specific urban area. The connected points provide a clear picture of increases or decreases in pollutant levels over time.
Advantages include:
- Trend Visualization: Clearly shows rises and falls in data over time
- Continuity: Offers a sense of flow and progression in data
- Comprehensive view: Easily overlays multiple data sets for correlation
Nonetheless, they can be less effective for discrete data or where sharp changes occur in a short period.
Pie Charts
Pie charts display the proportion of different components within a whole. In air pollution studies, they can effectively represent the share of various pollutants contributing to overall air quality in a specific area. For example, showing the composition of air pollutants in 2019 could help in understanding the major contributors to air quality issues.
Benefits of pie charts include:
- Proportional Data Representation: Clearly shows parts of a whole
- Quick Understanding: Easy to grasp at a glance
However, pie charts can be misleading when too many categories are presented or when values are similar, making it hard to discern differences.
Heat Maps
Heat maps visualize complex data, often representing variations in air pollutant concentrations across geographical areas. By using colors, they reveal which regions experience high pollution levels versus those with cleaner air. For example, a heat map of ozone levels in a metropolitan area can highlight pollution hotspots.
Some advantages are:
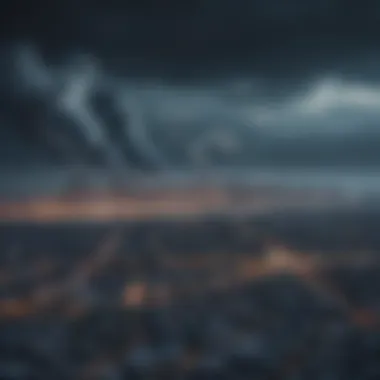
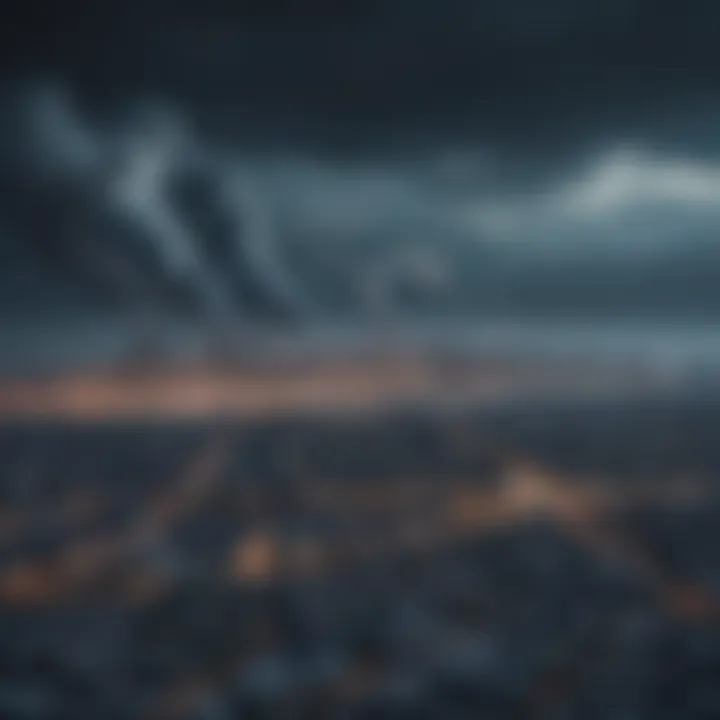
- Geographic Context: Clearly shows spatial distributions of pollutants
- Data Density: Effectively represents varying levels of pollution in one view
However, they can require careful interpretation to avoid misinforming viewers about the extent of pollution.
Scatter Plots
Scatter plots are beneficial for examining relationships between two or more variables. In air pollution, they can quantify correlations between airborne particulates and health outcomes. Each point represents a data pair, allowing analysts to observe patterns and anomalies.
Advantages include:
- Correlation Insights: Effectively shows relationships between variables
- Distribution Visuals: Displays data density and outliers clearly
Yet, scatter plots may require additional statistical analysis to draw meaningful conclusions and are best used in combination with other graph types.
Data Collection Methods
Accurate data collection methods are critical for understanding air pollution. These methods form the backbone of our ability to monitor, analyze, and respond to pollution levels. With reliable data, stakeholders can identify pollution sources, trends, and impacts. In this section, we will explore three significant methods: Ambient Air Quality Monitoring, Satellite Observations, and Modeling and Simulations. Each method plays a unique role in evaluating air quality and informing policy.
Ambient Air Quality Monitoring
Ambient air quality monitoring refers to the systematic measurement of air pollutants in various environments. This process involves the use of sensors and monitoring stations strategically placed in specific locations. These locations can be urban, rural, industrial, or near roads. The primary goal is to gather data about pollutant concentrations, including particulate matter and gases.
The data obtained from these monitoring stations is essential for several reasons:
- Real-Time Monitoring: Ambient air quality monitors provide real-time data, allowing authorities to respond quickly to pollution spikes.
- Trend Analysis: Long-term data collection helps identify trends in air quality over time, thereby assisting in predicting future conditions.
- Health Assessments: By understanding pollution levels, health officials can assess risks to public health and take action to mitigate those risks.
Satellite Observations
Satellite observations offer a different perspective on air quality monitoring. This method uses satellite technology to measure air pollution from space, covering vast areas that ground-based monitors might miss. Satellites such as NASA’s Aura and the European Space Agency’s Sentinel provide valuable information on atmospheric composition.
The advantages of satellite observations include:
- Broad Coverage: Satellites can monitor remote areas, making it possible to gather data on regions where ground stations are not available.
- Global Reach: They provide data on pollution levels globally, enabling scientists to analyze international patterns and impact.
- Integration with Other Data: Satellite data can be combined with information from ground monitors, creating a more comprehensive view of air quality.
Modeling and Simulations
Modeling and simulations involve the use of mathematical and computational techniques to represent and analyze air quality data. These models simulate the physical and chemical processes affecting pollutants in the atmosphere. They can predict how pollutants travel, transform, and disperse.
Key benefits of this method are:
- Scenario Analysis: Models can simulate different scenarios, helping policymakers understand possible outcomes based on various pollution control measures.
- Resource Efficiency: Simulations can reduce the need for extensive field studies, saving time and resources while still providing insights.
- Policy Impact Evaluation: By comparing model predictions with historical data, policymakers can evaluate the effectiveness of current regulations and adjust strategies accordingly.
By understanding these data collection methods, stakeholders can make informed decisions regarding air quality management and policy development.
Reliable data collection is not just about gathering numbers; it is about making informed choices that impact public health and environmental quality. A thorough understanding of the strengths and limitations of each method allows for better overall analysis of air pollution.
Air Pollutants: A Closer Look
Understanding air pollutants is essential in comprehending the broader context of air pollution itself. These substances can have significant effects on both human health and the environment. The examination of specific air pollutants helps clarify their sources, behaviors, and impacts. Recognizing these factors can inform policy decisions and public awareness. Each pollutant possesses distinct characteristics, and analyzing them provides valuable insights into their roles in air quality.
Particulate Matter
Particulate Matter (PM) refers to a mixture of solid particles and liquid droplets found in the air. Its components can include dust, dirt, soot, and smoke. The size of these particles is crucial; PM is classified into PM10 and PM2.5.
- PM10 consists of particles with a diameter of 10 micrometers or smaller. These can be inhaled into the lungs but not reach the bloodstream.
- PM2.5, on the other hand, includes finer particles that can penetrate deeper into the respiratory system and even enter the bloodstream.
Exposure to high levels of particulate matter is linked to various health issues, including respiratory diseases, cardiovascular problems, and premature death. The sources of PM are numerous, ranging from vehicular emissions to industrial activities and natural events like wildfires. The use of graphs to visualize PM levels in different locations can help identify pollution hotspots and trends over time.
Nitrogen Dioxide
Nitrogen Dioxide (NO2) is a reddish-brown gas with a characteristic sharp odor. It is primarily produced from burning fossil fuels, particularly in vehicles and power plants. This gas plays a significant role in the formation of smog and can cause various health issues.
- Respiratory Effects: Short-term exposure can aggravate asthma and decrease lung function.
- Environmental Impact: NO2 contributes to the formation of ground-level ozone, which can harm vegetation and ecosystems.
Graphs depicting nitrogen dioxide concentrations can illuminate how urbanization and traffic patterns affect air quality. Such visualizations can aid officials in devising regulations to limit emissions and improve public health.
Sulfur Dioxide
Sulfur Dioxide (SO2) is a colorless gas with a pungent smell. It is mainly emitted from volcanoes and industrial processes, especially the burning of fossil fuels containing sulfur, like coal and oil. The importance of monitoring SO2 levels becomes clear when considering its impacts:
- Acid Rain Formation: SO2 can react with water vapor in the atmosphere, leading to the production of acid rain, which has harmful effects on water bodies and soil.
- Health Risks: Exposure to sulfur dioxide can result in respiratory problems such as asthma attacks and bronchitis.
Graphing sulfur dioxide emissions and their correlation with industrial activity can highlight the effectiveness of pollution control strategies and the need for better regulations.
Carbon Monoxide
Carbon Monoxide (CO) is a colorless, odorless gas produced by incomplete combustion of carbon-containing fuels. Major sources include vehicles, industrial processes, and wildfires. Due to its properties, CO is undetectable without instrumentation, which adds an element of danger.
- Health Impacts: CO can hinder the blood's ability to carry oxygen, leading to serious health risks, such as impaired vision, reduced cognition, and in severe cases, death.
- Concentration Levels: Even low levels of carbon monoxide can be problematic, particularly in enclosed spaces.
Graphs that track carbon monoxide levels in urban environments can provide insight into pollution levels relative to population density and traffic congestion. They can also serve as a tool to implement necessary policies targeting emission reduction.
In summary, a closer examination of air pollutants like particulate matter, nitrogen dioxide, sulfur dioxide, and carbon monoxide reveals the multifaceted nature of air quality and its implications for public health. Graphical representations of this data can provide crucial insights, guiding effective policy and public awareness.
Temporal Trends in Air Pollution Data
Understanding temporal trends in air pollution data is crucial for addressing current environmental issues. Trends reflect how air pollution levels change over time, allowing for better management and policy implementation. They are essential in evaluating the effectiveness of regulations and public health initiatives aimed at reducing air pollutants.
By analyzing these trends, researchers can identify patterns related to specific pollutants, such as particulate matter or sulfur dioxide. This information often reveals how various factors, like industrial activities or transportation changes, impact air quality.
Longitudinal Studies
Longitudinal studies play an important role in understanding how air pollution evolves over an extended period. These studies gather data at multiple points in time, enabling researchers to observe changes and long-term trends. They often highlight critical findings, such as increases in pollution during specific seasons or declines in certain areas due to regulatory efforts.

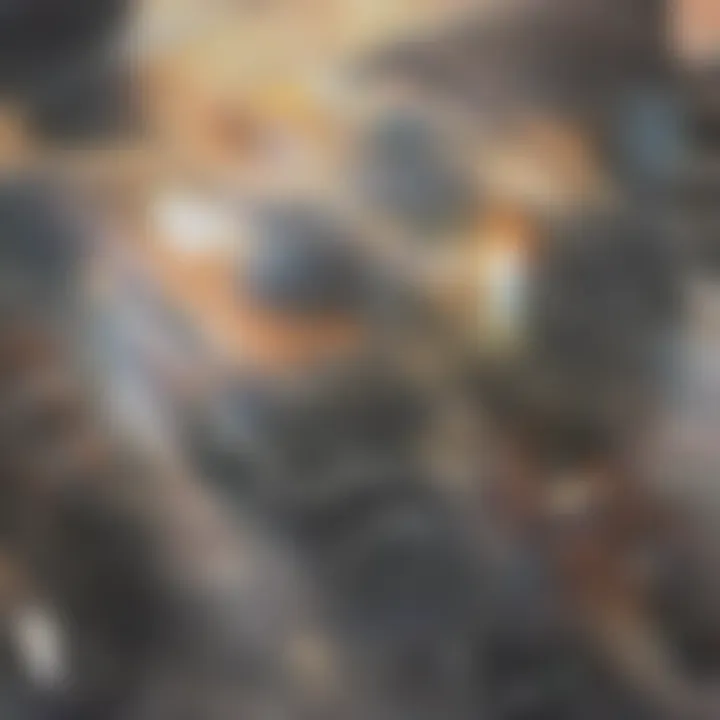
For instance, a longitudinal study may analyze data over a decade and identify periods of significant pollution spikes. This can help pinpoint the causes behind these changes, such as economic developments or major environmental policies. These insights can drive further research and facilitate more effective air quality management strategies.
Seasonal Variations
Seasonal variations significantly affect air pollution levels. Different weather patterns can alter the concentration of pollutants in the atmosphere. For example, higher levels of ozone are typically observed in warmer months, while winter can see increases in particulate matter due to heating systems. Understanding these variations allows for more targeted responses to air quality issues.
Seasonal analyses can also inform public health campaigns. By anticipating periods of higher pollution levels, authorities can take preemptive measures to protect vulnerable populations. Targeted strategies, such as issuing health advisories during expected peaks, can be designed based on these seasonal insights.
Analyzing temporal trends is essential for data-driven decision-making regarding air quality management.
Geographical Variations in Air Quality
Geographical variations in air quality play a critical role in understanding air pollution. They highlight how factors such as location, urbanization, and industrial development affect the levels and types of pollutants present in the atmosphere. These variations are essential for policymakers, researchers, and public health officials to devise effective measures for air quality management and improvement.
Air pollutants do not distribute evenly across different geographic areas. Urban regions often experience higher levels of pollutants like nitrogen dioxide and particulate matter due to high traffic volumes, dense populations, and industrial emissions. In contrast, rural areas may have better air quality, but they can still face challenges such as agricultural pollution or nearby industrial activities.
Key benefits of studying geographical variations in air quality include:
- Targeting interventions: Understanding the specific pollutants in various locations helps direct public policies and pollution reduction efforts more efficiently.
- Enhancing public health: By identifying areas with concerning air quality, health professionals can focus on preventive measures to protect vulnerable populations.
- Raising awareness: Highlighting geographical disparities can educate communities about risks and encourage local actions to improve air quality.
Factors to consider when analyzing geographical variations include terrain, climate, and local regulations. For example, mountainous regions may experience different dispersion patterns for pollutants compared to flat plains. Furthermore, climatic conditions can influence the chemical reactions that form secondary pollutants like ozone. These insights allow for more precise modeling and predictions regarding air quality.
Urban vs. Rural Areas
Urban areas typically have higher levels of air pollution compared to rural regions. This can be attributed to increased vehicle emissions, industrial activities, and population density. The concentration of buildings and infrastructure creates environments where pollutants can accumulate, causing health problems for residents.
In contrast, rural areas, while often perceived as having cleaner air, are not exempt from pollution. Agricultural practices, such as the use of fertilizers and pesticides, contribute to air quality issues. Dust from unpaved roads and open fields can also lead to particulate matter entering the air.
Contrast between urban and rural air quality:
- Urban Areas:
- Rural Areas:
- High levels of nitrogen dioxide and particulate matter.
- Effects of traffic congestion and industrial emissions.
- Historical data shows deteriorating air quality in many cities over time.
- Potential spikes in pollutants from agricultural sources.
- Lower levels of traffic-related emissions overall.
- The presence of natural sources of air pollution, like wildfires.
Global Perspectives
A global perspective on air quality is imperative for understanding how air pollution varies by region due to differing regulations, economic development, and environmental policies. Countries in different stages of development often show stark contrasts in their air quality profiles.
In developed nations, stringent regulations and advanced technologies help maintain relatively better air quality. Meanwhile, developing countries may struggle with inadequate infrastructure, leading to higher pollution levels. Such disparities can impact global health and climate change.
Factors contributing to global variations in air quality:
- Regulatory Differences: Countries with strict environmental laws tend to have lower pollution levels.
- Economic Development: Rapid industrialization in countries can lead to significant increases in emissions.
- Climate Change: Changes in climate patterns can influence air pollution distributions worldwide.
Understanding these global perspectives allows for collaborative international efforts to address air pollution. Organizations such as the World Health Organization and the United Nations Environmental Programme play essential roles in this mission. Through sharing data and best practices, nations can learn from each other to improve air quality universally.
Utilizing Graphs for Policy Making
Graphs are an integral part of formulating policies related to air quality. These visual tools provide critical information that simplifies complex data sets, making them accessible for policymakers and stakeholders. In an era where air pollution poses significant risks to public health and the environment, the ability to utilize graphs effectively can greatly influence regulatory decisions and resource allocation.
Using graphs can help in assessing the impact of environmental regulations and public health initiatives. Graphs illustrate trends and variations in air quality over time, revealing the effectiveness of implemented strategies and highlighting areas needing further attention. When key data points are presented visually, they allow for quicker interpretations and informed discussions. This aids policymakers in identifying pollution hotspots and areas where intervention could yield the highest benefit.
Regulatory Frameworks
Understanding air pollution regulation requires a comprehensive approach that integrates sophisticated data representation. Graphs act as a bridge between scientific data and legal frameworks. They enhance the visibility of air quality issues, allowing for informed decision-making based on empirical evidence.
For instance, regulatory bodies like the United States Environmental Protection Agency (EPA) often utilize graphs to present air quality indices to the public. These visualizations help illustrate compliance with air quality standards set under laws like the Clean Air Act. As such, graphs provide transparency related to air pollution levels, thus boosting public trust in regulatory actions.
Some key points regarding the effectiveness of graphs in regulatory frameworks include:
- Transparency: Visual data reporting makes regulatory findings clear to the public, increasing accountability.
- Trend Analysis: Graphs can highlight improvements or deteriorations in air quality, informing ongoing policy adjustments.
- Comparative Assessments: They allow for comparisons across different regions or timeframes, which can be critical for allocating resources.
Public Health Initiatives
Public health initiatives aimed at combating air pollution depend heavily on data-driven insights, and graphs play a central role in this process. By visualizing data on air quality and health impacts, stakeholders can identify vulnerabilities within communities.
For example, studies often show correlations between air pollution levels and respiratory diseases. Graphs that depict these relationships help public health officials justify intervention programs and mobilize community support. These graphical presentations facilitate a clearer understanding of the stakes involved, making them pivotal in strategizing public health responses to air pollution.
Key considerations in using graphs for public health initiatives include:
- Targeting Populations: Graphs can pinpoint areas and populations most impacted by poor air quality, directing interventions where they are needed most.
- Tracking Progress: Over time, graphs can be used to illustrate the impact of public health campaigns on air quality and health outcomes.
- Community Engagement: Visual data can help engage the public by making complex information relatable, thereby fostering community action against air pollution.
"Graphs are not just images; they are essential tools that translate data into stories that influence policy and drive change."
Limitations of Graphical Representations
In the context of air pollution, graphical representations are essential tools for interpreting data. However, these representations come with notable limitations. Understanding these limitations is crucial for researchers, educators, and policy-makers who seek to draw accurate conclusions from graphical data. This section explores two main pitfalls: data over-simplification and potential misinterpretations.
Data Over-simplification
Graphs excel at distilling complex datasets into more digestible formats. This utility, while beneficial, can often lead to a significant over-simplification of data. When atmospheric pollutants are mapped or charted, subtle variations and intricate patterns may vanish, overshadowed by broad trends. For instance, a line graph showing reductions in sulfur dioxide levels may obscure localized spikes that could indicate severe pollution in certain urban areas.
Moreover, complex interactions between various pollutants might not be fully captured within a singular graphical framework. Consider the case of particulate matter; its impact on health can be influenced by meteorological conditions and local emissions sources. When reduced to basic bars or lines, the multi-faceted nature of air quality data may become an afterthought.
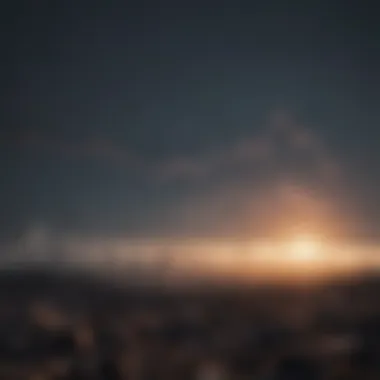
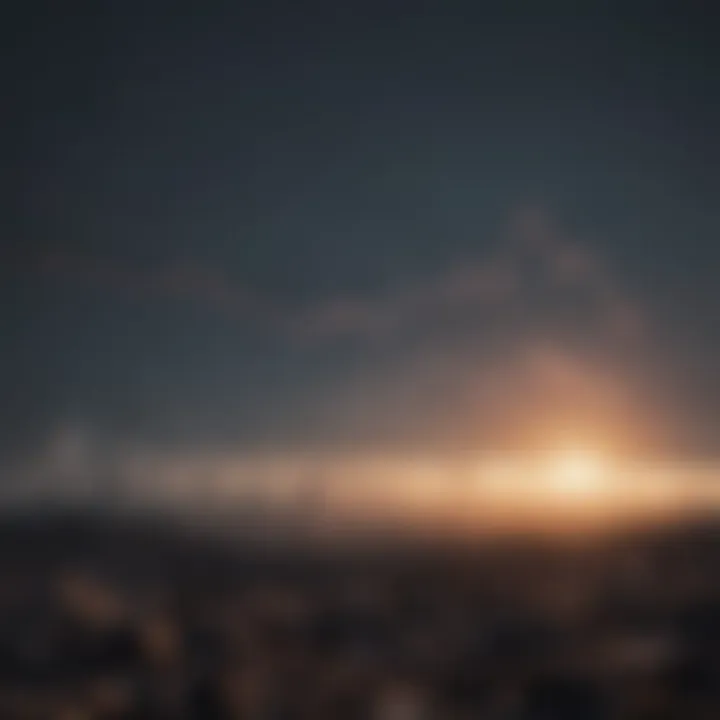
The simplification of data into charts must be balanced against the potential loss of critical insights.
This raises questions about how metrics are defined and selected for presentation. The choice of timeframe, the averaging of data points, and the exclusion of certain variables can fundamentally alter the narrative told by the graphs. Stakeholders must remain cognizant of these aspects to avoid forming inaccurate conclusions based on incomplete data.
Potential Misinterpretations
The risk of misinterpretation looms large with the use of graphs in presenting air pollution data. Graphical representations, if not accompanied by comprehensive context, can lead the audience to misunderstand the nature and severity of pollution issues. For example, a pie chart representing the sources of nitrogen dioxide emissions may imply that transportation is the sole contributor, neglecting industrial and natural sources which also play significant roles.
Additionally, visual elements, such as color choices or scale discrepancies, can lead to skewed perceptions. A heat map indicating areas of high pollution might be interpreted as a direct cause of health issues without recognizing that correlation does not imply causation. The nuances behind why certain areas exhibit high pollution levels require a thorough examination that goes beyond visual data representation.
When stakeholders, including policy-makers and community leaders, rely on these graphical summaries without sufficient context, their decisions may reflect an incomplete understanding. It is vital to depict not just the data but also the underlying factors influencing that data.
Future Directions in Graphing Air Pollution Data
Future directions in graphing air pollution data are pivotal for augmenting public understanding and guiding policy decisions. As technological advancements continue to shape data analytics and visualization capabilities, it becomes necessary to explore and adopt novel methodologies. Increased transparency and accessibility to air quality data can empower stakeholders to make informed choices.
Increasingly, there is a demand for enhanced visualization techniques to effectively convey complex datasets. Traditional graphs may not suffice in presenting multifaceted information. Therefore, researchers and analysts are exploring innovative graphics that can visually represent not only the level of pollutants but also their temporal and spatial dynamics.
There are significant benefits associated with these new directions. Advanced data visualizations can lead to deeper engagement from the public and more informed discussions around air quality issues. Improved clarity could result in better public health initiatives and regulatory responses.
Considerations are critical. The challenge remains how to present an abundance of data without overwhelming the audience. Balance must be achieved so that clarity does not come at the expense of depth. Thus, it is essential to tailor visualizations for diverse audiences, ensuring they can grasp the critical messages conveyed without misinterpretation.
Advanced Visualization Techniques
Advanced visualization techniques hold the potential to revolutionize the way air pollution data is interpreted. Techniques such as interactive graphics, 3D modeling, and dynamic visualizations can provide a more comprehensive understanding of air quality trends. For instance, interactive dashboards can allow users to manipulate variables and explore data from different perspectives.
These techniques enable analysts to showcase real-time data, revealing fluctuations in air quality that could be overlooked in static representations. This can be particularly beneficial for policymakers aiming to assess the impact of environmental regulations or urban planning decisions in real-time.
"Advanced visualizations can transform dense datasets into informative and understandable images that prompt action."
Incorporating tools that enable predictive analytics can also be considered an advanced technique. By modeling future air quality scenarios based on historical data, stakeholders can better prepare and mitigate upcoming challenges.
Incorporating Big Data and AI
The integration of big data and artificial intelligence is reshaping how air pollution is graphed. With the availability of vast amounts of environmental data from sensors, satellites, and ground-based monitoring, traditional graphing methods must evolve. AI algorithms can process this data swiftly and identify patterns that human analysts might not easily see.
For instance, machine learning can be applied to predict pollution levels based on various factors, including weather patterns and human activities. These predictions can then be visually represented through graphs that convey both current and forecasted air quality. Such foresight can support proactive measures in urban planning and pollution control.
Additionally, using big data analytics can enhance the granularity of air quality graphs. Instead of aggregating data into broad geographic regions, micro-level analyses can be conducted. This allows for localized insights, making it easier for communities to address specific air pollution sources.
In summary, the future of graphing air pollution data is bright but comes with challenges. Embracing advanced techniques and the integration of big data and AI will enhance insights into air quality, ultimately fostering healthier environments.
Case Studies: Effective Use of Graphs
In the landscape of air pollution analysis, case studies serve as vital tools for evaluating the effectiveness of graphing methods. Through concrete examples, they illuminate the practical application of theoretical principles discussed in previous sections. Case studies not only highlight the successful visualization strategies but also provide valuable insights into the challenges confronted during data representation. This leads to a better understanding of the real-world implications of air quality information.
National Air Quality Reports
National air quality reports are critical for monitoring pollution levels and trends across regions. These reports employ diverse graphical representations to present data clearly and effectively. Statistically, the Air Quality Index (AQI) is a common component in these reports. The AQI simplifies air quality data into an easily understandable format, allowing the public to grasp daily air pollution levels quickly.
Graphs such as line charts are typically used to represent AQI over time, showing variations caused by seasonality, weather fluctuations, or regulatory changes. This temporal analysis allows policymakers to recognize long-term trends and the impact of interventions. By observing patterns, stakeholders can make informed decisions about public health initiatives.
Moreover, these reports often utilize bar graphs to compare local air quality levels across different regions. This reinforces the concept that air pollution is not uniformly distributed and can vary significantly based on local sources.
"Case studies reveal that visualizing air pollution data can bridge the gap between scientific research and public understanding."
In summary, national air quality reports demonstrate how effective graphing can bring clarity to complex datasets, thus enhancing public awareness and guiding policy decisions.
Local Initiatives and Their Outcomes
Local initiatives that tackle air pollution often provide insightful case studies of effective graph usage. Such initiatives showcase specific actions taken at community levels and their subsequent impacts. For example, cities may launch programs targeting vehicular emissions and use graphs to track changes in air quality before and after implementation.
Scatter plots can visually represent the correlation between emission reductions and corresponding improvements in air quality metrics. These relationships become evident through organized data, revealing which practices yield the best results. This empirical evidence can motivate other regions to implement similar initiatives.
Additionally, through heat maps, communities can visualize pollution hotspots. This localization facilitates targeted interventions where they are most needed, optimizing resource allocation. Stakeholders can identify areas for improvement, while also assessing whether initiatives are effective in decreasing pollution levels.
These local initiatives often include public forums where data is presented graphically. This approach aids in fostering community engagement and awareness. Individuals become informed advocates for air quality improvements in their neighborhoods.
Ultimately, case studies featuring local initiatives highlight how targeted graph representations can drive actionable change. They serve as exemplary models for leveraging data to promote healthier environments.
Culmination: The Role of Graphs in Air Pollution Discourse
The utilization of graphs in the discourse surrounding air pollution has become critical for numerous reasons. Graphs serve not merely as representations of data but as tools that facilitate understanding and communication regarding air quality issues. They play a pivotal role in synthesizing complex information into digestible formats, thus enhancing the ability of stakeholders—from policymakers to the general public—to make informed decisions.
Graphs encapsulate significant insights into temporal trends, geographic variations, and pollutant types. This capability to present multifaceted data sets coherently directly influences how air pollution is perceived and addressed in societal contexts.
Summative Insights
In summary, graphs have proven invaluable in articulating the challenges and developments in air quality. They allow for an immediate grasp of changes over time and correlations between different air pollutants. Additionally, they enhance the dialogue among scientists, policymakers, and the community. When stakeholders can see air quality data visually represented, they can identify patterns, acknowledge trends, and form strategies that target pollution reduction effectively.
Here are several key benefits to consider as we reflect:
- Data Clarity: Graphs clarify complex numerical information.
- Trend Identification: They highlight shifts in air quality over time.
- Comprehensive Communication: Stakeholders can communicate crucial information more effectively.
Graphs essentially bridge gaps. They connect raw data to genuine impacts on public health. This connection is essential for promoting transparency in discussions about air quality.
Encouraging Ongoing Research
Encouraging ongoing research into air pollution through graphing techniques is vital. The dynamic nature of air contaminants and their effects necessitates constant scrutiny and updated data representations. Researchers need to explore advanced graphing methods, integrating technology like artificial intelligence and machine learning. This evolution can render graphs even more insightful.
Moreover, collaborative efforts among researchers can lead to unified visual standards for data presentation. Establishing common frameworks can facilitate broader understanding across disciplines. By promoting teamwork and shared methodologies, researchers can propel the conversation and recommendations surrounding air quality.
Finally, as environmental challenges intensify, the need for clarity in air pollution metrics grows stronger. Continued investment in graphing methods will ensure that vital data remains accessible and actionable. This ongoing commitment will ultimately cultivate a more informed citizenry, ready to engage in the robust discussions necessary for combating air pollution effectively.