Essential Insights into the NGS QC Toolkit for Research
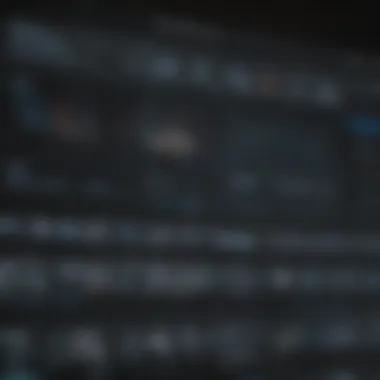
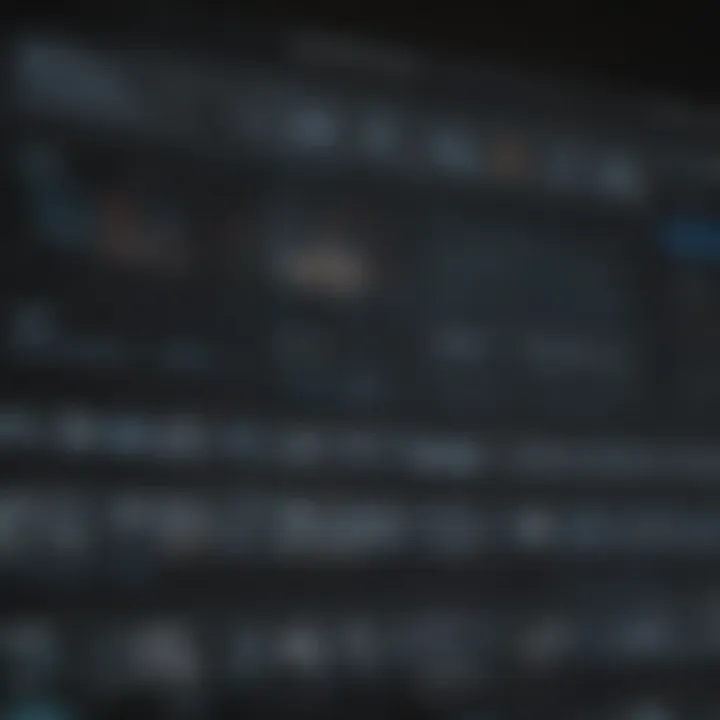
Intro
In recent years, the explosion of genomic data has transformed the field of biology. However, with great data comes great responsibility—specifically, ensuring the quality and reliability of that data. Researchers seeking to understand the genetic underpinnings of health and disease are increasingly turning to Next Generation Sequencing (NGS) technologies. In the quest for accuracy, the NGS Quality Control (QC) Toolkit has emerged as an essential component for researchers navigating this complex landscape.
This toolkit encompasses various tools and methodologies designed to assess and assure data integrity throughout the sequencing process. As the modern scientific endeavor demands precision, understanding the functionalities and implications of the NGS QC Toolkit is of paramount importance for students, educators, and professionals alike.
Far from being just another set of tools, the NGS QC Toolkit represents a commitment to scientific rigor, emphasizing that quality data is the foundation upon which valid research is built. In this article, we will explore the fundamental aspects of the NGS QC Toolkit, including its components, the methodologies it employs, the importance it holds in biological research, and the challenges associated with quality assessment. This endeavor seeks to provide a comprehensive overview, enabling our discerning audience to grasp the nuances of genomic data quality control.
Prolusion to NGS QC Toolkit
In the realm of genomics, the quality of data generated through Next Generation Sequencing (NGS) has become a cornerstone for reliable research outcomes. Understanding the NGS QC Toolkit is paramount for researchers who navigate through a plethora of genomic data. This toolkit encompasses various strategies and components that aid in assessing and ensuring the integrity of sequencing results, thus providing a solid foundation for scientific discoveries.
Understanding Next Generation Sequencing
Next Generation Sequencing represents a significant leap in genetic research capabilities. Unlike traditional methods, NGS enables high-throughput sequencing, allowing researchers to analyze vast quantities of genetic material rapidly. By using platforms such as Illumina and Oxford Nanopore, scientists can explore intricate genetic architectures and mutations at a genomic scale. However, with these opportunities come a myriad of challenges, primarily focusing on maintaining quality throughout the sequencing process. Researchers must be aware that even a small error in sequencing can result in misleading interpretations and conclusions, potentially derailing entire studies.
The accuracy in genomic analysis directly correlates with the reliability of NGS results — this is where quality control becomes essential.
Importance of Quality Control
Quality Control (QC) in the context of NGS is not merely a preliminary checklist; it is a critical process that permeates every stage of genomic analysis. Without stringent QC measures, the risk of erroneous data escalates, which can lead to flawed hypotheses and wasted resources. The importance of QC can be highlighted through several key points:
- Data Integrity: Regular assessments prevent sequencing errors from corrupting entire datasets.
- Reproducibility: Adopting robust QC practices allows for consistent results across multiple experiments, enhancing the validity of findings.
- Biological Significance: High-quality data provides a clearer picture of biological processes, thus facilitating accurate biological interpretations and treatments.
By recognizing the pivotal role of the NGS QC Toolkit, researchers can better harness the power of sequencing technologies, ensuring that their findings contribute meaningfully to scientific knowledge.
Components of the NGS QC Toolkit
The NGS QC Toolkit comprises several pivotal components that function synergistically to uphold the integrity of genomic data throughout the research process. Each element plays a distinct role, ensuring that only high-quality sequences are analyzed. Understanding these components not only aids researchers in selecting the right tools but also enhances the reproducibility of their results. The ramifications of neglecting quality control in next-generation sequencing can be severe, leading to misinterpretations and potentially erroneous conclusions.
Quality Assessment Metrics
Quality assessment metrics serve as the backbone of the NGS QC Toolkit. These metrics help in quantifying the quality of the sequencing data, which is fundamental in making informed decisions regarding further analysis. Among the key metrics employed are Phred scores, which indicate the confidence level in base calling, and sequence duplication rates, which can signal potential issues in library preparation.
Utilizing these metrics allows researchers to efficiently identify problematic areas in their sequencing data. Hence, implementing a robust assessment strategy can lead to more precise genomic interpretations. These practices not only uphold the research standard but also nourish the credibility of scientific findings.
Data Filtering Techniques
Data filtering techniques involve processing raw sequencing data to eliminate lower quality sequences and artifacts, thereby maximizing data usability.
Trimming
Trimming is vital for enhancing data quality by removing low-quality bases from the ends of reads. This aspect of Trimming ensures that the remaining sequences are representative of the original template, which is crucial for accurate downstream analyses.
One key characteristic of trimming is its ability to significantly reduce sequence errors. This makes it a popular choice among researchers entrenched in genomic studies since it improves the overall accuracy of sequence assignments. A unique feature of trimming is its effectiveness in adjusting read lengths, which can aid in aligning sequences against reference genomes, thus providing more reliable alignments. While trimming undoubtedly enhances quality, it may inadvertently lead to the loss of valuable data if overzealously applied, warranting a careful balance.
Adapter Removal
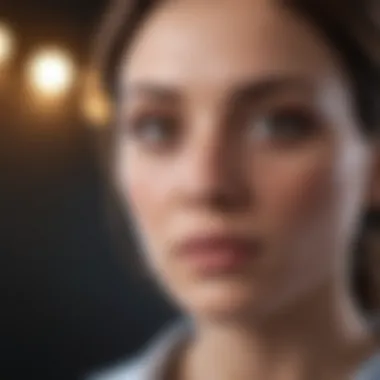
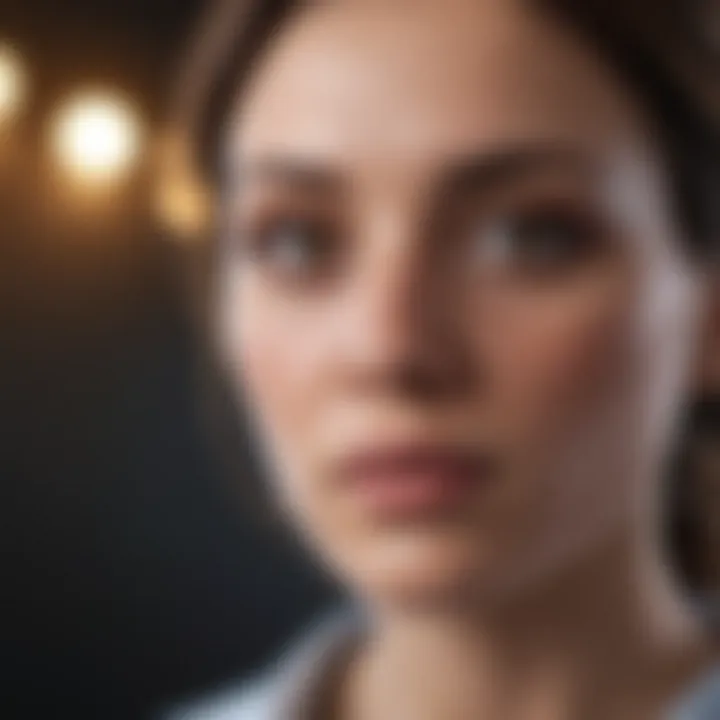
Adapter removal is another critical step in the filtering process. This technique involves detecting and excising adapter sequences that attach to DNA libraries during the sequencing preparation phase. It is essential for yielding clean reads free of synthetic sequences, which could otherwise confound analyses, especially in applications like variant calling or expression profiling.
The key characteristic of adapter removal lies in its capacity to improve the fidelity of read mapping and increase the overall quality of the data. This makes it a beneficial choice to include in the analysis pipeline. Notably, one unique feature of adapter removal tools is their algorithmic sophistication. They employ pattern matching to identify the remnants of adapter sequences accurately. While highly effective, the disadvantage is that improper removal can lead to the introduction of biases, necessitating trustworthy tools and careful parameter settings to mitigate such risks.
Read Mapping Quality
Read mapping quality is a crucial concern in NGS, as it impacts the interpretation of genomic data. High mapping quality indicates confidence in the alignment of reads to a reference genome, serving as a filter for identifying mismatches and potential variant calls. Fluctuations in read mapping quality can arise from various factors, including the variability in read lengths, sequencing depth, and the attributes of the reference genome itself.
By employing robust methodologies to assess read mapping quality, researchers can avoid pitfalls in variant identification. Ensuring a high degree of alignment confidence is paramount in genomic studies, as it influences the reliability of biological interpretations. Consequently, understanding and applying these metrics is invaluable to any researcher aiming to elevate the quality and reliability of their sequencing projects.
Methods of Quality Control in NGS
Quality control in Next Generation Sequencing (NGS) is not just an optional step; it is an essential pillar that underpins the reliability and validity of genomic data analysis. Effective methods of quality control can significantly mitigate issues that might skew results or lead to erroneous interpretations. Establishing robust quality control processes is vital for researchers aiming to produce accurate and reproducible results. When examining the many facets of NGS, one can see that a systematic approach to quality management is integral, as it enhances overall data integrity and helps scientists maintain confidence in their findings.
Software Tools Overview
The emergence of software tools tailored for quality control in NGS has transformed the landscape of genomic research. These tools provide researchers with powerful means to assess and improve the quality of the sequenced data. Here, we delve into three prominent software options, each offering unique features that contribute to quality management in NGS.
FastQC
FastQC has grown to be tremendously popular within the NGS community. Its primary strength lies in its ability to offer a snapshot of the quality of sequencing data in a user-friendly format. This tool highlights potential issues within the data, such as low quality scores or overrepresented sequences.
A key characteristic of FastQC is its versatility in handling various file formats and its capability to generate diverse analyses—including base quality distribution and GC content statistics. Its user-friendly interface makes it particularly appealing for researchers, as it simplifies the quality assessment process. One unique attribute of FastQC is its graphical outputs, which allow for quick insight at a glance.
However, while it gives a watertight overview, FastQC is not infallible. It cannot inherently correct any identified issues; users must take further steps to rectify problems flagged by the tool. Thus, while it’s a strong initial tool for quality checks, it should be complemented with additional software for a thorough QC protocol.
Trimmomatic
Trimmomatic is an invaluable asset in the toolkit when it comes to the trimming of sequence reads. The significance of this tool lies in its ability to improve the overall data quality by removing adapter sequences and low-quality bases from the reads, ensuring that only high-quality data is used in downstream analyses.
One key characteristic of Trimmomatic is its adaptive trimming option. This enables it to examine the quality of the bases across each read and trim off sequences that fail to meet specified quality thresholds. This adaptability is particularly useful in diverse sequencing scenarios where read quality can vary significantly. The tool's unique feature of employing a sliding window mechanism helps maintain the integrity of the data while improving its usability.
Though Trimmomatic excels in its functional roles, it can be somewhat resource-intensive, especially in larger datasets. As such, users may need to allocate adequate computing resources to fully utilize its capabilities without running into performance delays during analysis.
MultiQC
MultiQC stands out as a groundbreaking tool for aggregating results from various quality control analyses, including those from FastQC and Trimmomatic. Its main contribution lies in its ability to compile outputs from different software into a cohesive report, allowing researchers a comprehensive view of their data quality in one consolidated interface.
A noteworthy characteristic of MultiQC is its capability to visualize a multitude of metrics in graphical format, which makes it easier for users to identify patterns and potential issues in their data. This is particularly advantageous for projects involving large datasets where manual review of quality metrics would be impractical. The tool's unique feature of generating interactive reports enhances the user experience and facilitates easier presentations of findings to colleagues or stakeholders.
On the flip side, while MultiQC excels in visualization and aggregation, it doesn’t perform quality checks or data processing on its own. Users must ensure that they have the appropriate tools already implemented in their workflows before utilizing it as part of their QC strategy.
Statistical Methods for Validation
Beyond software tools, applying statistical methods for validation is crucial in confirming the efficacy of quality control measures. Statistical approaches help in detecting anomalies, establishing thresholds for data integrity, and validating the reliability of the results. By employing sophisticated statistical models, researchers can rigorously evaluate data quality, ensuring that they can trust their NGS findings and contribute meaningfully to scientific understanding.
Understanding these multifaceted methods enables researchers to design more robust experiments and effectively communicate their results according to scientific standards.
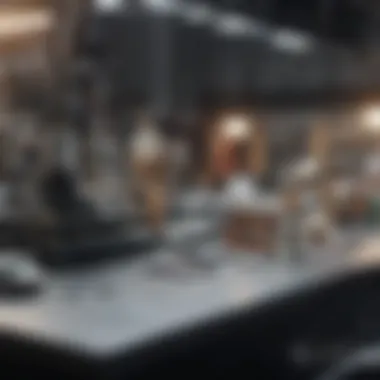
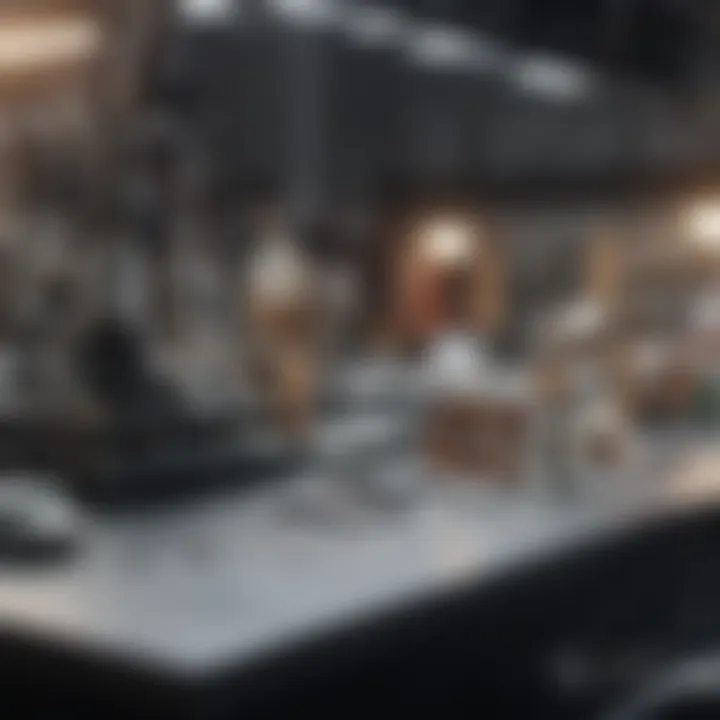
Challenges in NGS Quality Control
Understanding the challenges surrounding quality control in Next Generation Sequencing (NGS) is critical for researchers aiming to synthesize accurate genomic data. Quality assurance measures not only ensure the reliability of results, but also can be the difference between a successful study and one that yields inconclusive or misleading findings. As we dig into this section, we will uncover common issues that plague NGS processes and discuss strategies for overcoming them, thereby providing readers with insights that can directly enhance their research endeavors.
Common Sources of Error
When it comes to quality control in NGS, various factors can lead to errors that compromise data integrity. It’s not all as cut and dry as one might think. Here are some notable sources of error that researchers often encounter:
- Contamination: Contamination during sample preparation and processing can severely affect results. It’s crucial to maintain sterile working conditions to minimize this threat.
- Instrumentation Errors: Devices such as sequencers, if not calibrated or maintained properly, can produce faulty data. Regular equipment checks can help mitigate this.
- Amplification Bias: PCR amplification can introduce biases. Choosing the right primers and optimizing the PCR conditions are essential steps to ensure that the data reflects the true genomic content.
- Handling Variability: Sample handling is particularly sensitive. Variations in the way samples are managed before sequencing can introduce discrepancies in the data.
- Bioinformatics Errors: The tools and algorithms used in data analysis may introduce errors if not properly validated or if used inappropriately.
Each of these issues demands attention. Implementing robust quality control measures at each stage of the NGS process is vital.
Addressing Batch Effects
Batch effects can be a thorn in the side of genomic studies, introducing systematic non-biological differences arising when samples are processed in separate batches. These differences may obscure genuine biological signals, hence compromising the results of the study. Here’s how to approach this:
- Randomization: When designing experiments, randomizing sample processing can be a straightforward yet effective technique to minimize batch effects. This ensures that any potential bias is evenly distributed across all experimental conditions.
- Control Samples: Incorporating control samples that are run alongside experimental ones can help in identifying batch effects. Comparing and harmonizing data from these controls can be invaluable.
- Statistical Adjustments: Software packages and statistical tools now exist that specifically address batch effects. Utilizing these models can assist in correcting results after data collection. For instance, the ComBat method is widely used to adjust for batch effects in genomics data.
- Regular Monitoring: Establishing a routine check to monitor data quality can facilitate early detection of any anomalies that suggest batch effects.
Addressing batch effects is not merely a technical formality; it’s an essential part of extracting valid scientific conclusions from NGS data. When researchers actively engage in identifying and mitigating issues like these, they can enhance the reliability of their genomic research significantly.
"Quality is not an act, it is a habit."
– Aristotle
Best Practices for Effective NGS QC
Ensuring quality control in next generation sequencing (NGS) is not just a matter of running a few basic checks; it's about instilling a mindset of rigorous assessment throughout the entire research process. Best practices in NGS QC function as the foundational pillars that uphold the integrity and trustworthiness of the results gathered from sequencing endeavors. Not only do these practices prevent errors that could skew results, but they also facilitate reproducibility and compliance with regulatory expectations. To maximize the utility of data acquired through NGS, implementing comprehensive and systematic QC protocols is key to driving meaningful scientific advancements.
Establishing Protocols
The establishment of protocols is a cornerstone to effective NGS QC, guiding researchers through the labyrinth of sequencing processes. These protocols should be tailored based on the specific goals of the study and modified as needed throughout the research lifecycle.
- Standard Operating Procedures (SOPs): Writing clear SOPs is essential. They outline every step, from sample collection to data analysis. This consistency ensures that everyone involved is on the same page and that practices are repeatable.
- Documentation: Keeping meticulous records of all procedures followed and the decisions made along the way is vital. Good documentation acts as a roadmap of sorts that can lead to improvements in future research.
- Training and Education: Regular training sessions for personnel can lead to a deeper understanding of quality metrics and tools. This ensures that everyone is equipped to identify potential issues before they compromise data quality.
Continuous Monitoring of Data Quality
The realm of NGS is dynamic, and the quality of data can change based on various factors such as equipment performance, sample integrity, and bioinformatics tools used. Thus, continuous monitoring is not just beneficial; it's imperative.
- Real-Time Analytics: Utilize software that offers real-time data quality statistics. This allows researchers to spot issues, such as sequence bias or low-quality reads, as they happen instead of waiting until after data analysis.
- Routine Calibrations: Periodic calibration of NGS instruments is crucial. Calibration should adhere to manufacturer guidelines and be documented diligently.
- Threshold Settings: Define acceptable thresholds for metrics like read length and quality scores before starting the analysis. By establishing these benchmarks, deviations can be spotted quickly, leading to actionable insights and adjustments.
"Quality isn't an act; it is a habit." – Aristotle
The integration of best practices in NGS QC is not merely an administrative task; it represents a commitment to excellence in scientific inquiry. By laying down robust protocols and investing in ongoing monitoring of data quality, researchers fortify their findings against the whims of chance, thus establishing a more reliable foundation for future research.
Case Studies Highlighting NGS QC Importance
Understanding the real-world application of the NGS QC Toolkit is crucial for grasping its significance in the scientific community. Case studies serve as tangible examples, showcasing how effective quality control can influence research outcomes in various fields. By examining these detailed scenarios, researchers can draw valuable lessons and insights that underscore the importance of implementing rigorous quality control measures in their own work.
Clinical Genomics Applications
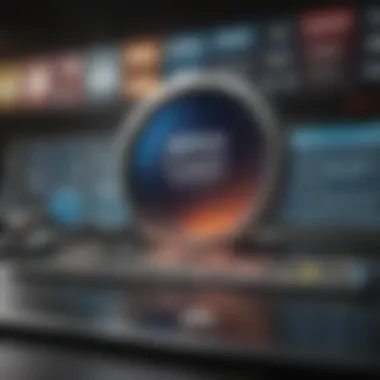
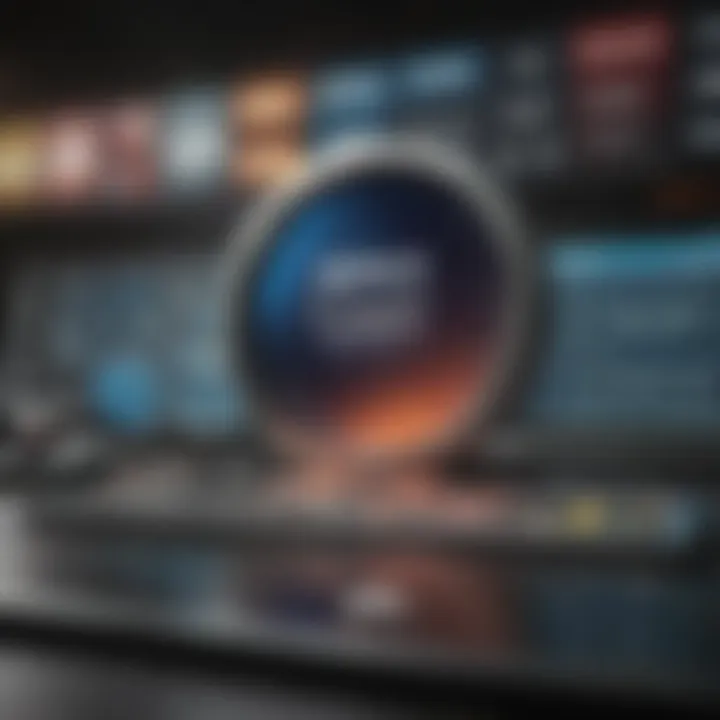
In the realm of clinical genomics, the stakes are often extremely high. Here, precision matters not just in terms of research outcomes, but often in patient care. For example, a study published in a leading medical journal examined the effectiveness of the NGS QC Toolkit in diagnosing genetic disorders. The researchers utilized various components of the toolkit to streamline their quality assessment process, ensuring that the sequencing results they reported were both reliable and reproducible.
By implementing robust quality control procedures, the team was able to significantly reduce the rate of false positives. This improvement not only bolstered the validity of their findings but also enhanced clinical decision-making. Practitioners could confidently use these results to guide treatment plans, ultimately improving patient outcomes.
Another salient point from clinical case studies is how quality control can address potential ethical issues. In a captivating example, a cancer research team adopted the NGS QC Toolkit to scrutinize their genomic profiles. They filtered out any incorrect or low-quality data, thus safeguarding against misdiagnosis. This meticulous approach prevented the propagation of erroneous conclusions, reinforcing the integrity of the entire research study.
Environmental and Evolutionary Studies
NGS QC is equally imperative in the context of environmental and evolutionary studies, where understanding biodiversity and ecosystems is critical. A notable case study involved researchers analyzing soil metagenomes to assess microbial diversity in urban versus rural landscapes. Without quality control, the data collected could have been misleading, as environmental factors often introduce noise into sequencing results.
In this study, the NGS QC Toolkit was employed to remove low-quality reads and perform specific adaptations on the data set. This critical step allowed the researchers to derive more accurate insights regarding microbial communities and their relation to environmental pressures. The outcome highlighted how the application of rigorous quality standards can lead to more informed conclusions about biodiversity and the impact of anthropogenic activities.
Moreover, another project focusing on evolutionary dynamics used the NGS QC Toolkit to compare genomic sequences across species. By controlling for quality, the study was able to identify evolutionary trends with greater clarity. The researchers could track how certain traits evolved and adapted over time based on high-quality genomic data, leading to significant findings in evolutionary biology.
This shows that quality control is not just an oversight; it is foundational to data integrity, affecting the broader implications of scientific research.
Future Directions in NGS QC
As we look ahead, the realm of Next Generation Sequencing (NGS) Quality Control (QC) is on the brink of significant evolution. With rapid technological advancements, the landscape of genomic research is changing, making it crucial to understand the future directions that NGS QC might take. Embracing these changes will provide researchers with the tools needed to enhance data integrity and ensure reliable findings in their studies.
Advancements in Software Development
The march of progress in software tools for NGS QC is quite astonishing. High-quality, accessible software is essential for facilitating seamless data management and analysis processes.
- AI Integration: One compelling focus of future software advancements is the incorporation of artificial intelligence. This can help in automating error detection, predicting potential issues based on historical data, or even suggesting corrective actions. Natural language processing can also make complex outputs more understandable for researchers from non-technical backgrounds.
- Real-time Analysis: The ability to analyze data in real-time is another anticipated trend. Right now, many tools require the entire dataset to be complete before quality metrics can be evaluated. As improvements occur, real-time feedback could allow researchers to make on-the-fly adjustments, enhancing efficiency.
- User-Centric Design: Continued emphasis on user experience will drive software developers to create more intuitive interfaces. This is especially significant because as NGS becomes more widely adopted in various fields, the diversity of users – ranging from seasoned bioinformaticians to newcomers in genomics – increases. User-friendly designs will lower the barriers to entry for many researchers.
The intersection of these advancements will undoubtedly lead to tools that not only improve accuracy but also speed up analysis in genomic workflows.
Integrative Approaches to Data Quality
The future of NGS QC holds exciting possibilities for integrative approaches that aim to enhance data quality across platforms and disciplines. Here, we can think about how the fusion of diverse data types may be the key to unraveling complex biological questions.
- Multi-Omics Integration: With the growth of various omics disciplines – genomics, transcriptomics, proteomics, and others – there’s a pressing need for software that can handle a breadth of data from different sources. This integrative method promises to enrich interpretations and insights derived from genomic data.
- Collaboration Between Different Fields: As NGS is applied more broadly, collaboration among biologists, bioinformaticians, and data scientists will be pivotal. This might lead to the establishment of new standards and frameworks ensuring quality metrics are consistent regardless of the field of application.
- Collaborative Platforms: We may also see a rise in platforms that facilitate collaborative analysis of NGS data. These tools could allow researchers from disparate institutions to pool resources while maintaining data integrity and quality control.
In short, the future of NGS QC is likely to be characterized by dynamism, where cutting-edge software tools, integrated methodologies, and collaborative networks will converge to enhance the reliability of genomic research. There’s much to anticipate, indicating a bright future for those involved in scientific inquiry and exploration.
The End
In bringing all the pieces together, the conclusion of our journey through the NGS QC Toolkit shines a spotlight on its pivotal role in the landscape of scientific research. As we tread deeper into the world of genomic studies, ensuring data integrity has become non-negotiable. The significance of quality control transcends mere statistics; it is the backbone of reliable scientific conclusions.
Summarizing Key Takeaways
To encapsulate the essence of this article, here are the core takeaways:
- Quality Control's Necessity: The integrity of genomic data directly affects the outcomes of research. High-quality data leads to credible results.
- Toolkit Components: Familiarity with the various elements of the NGS QC Toolkit, such as data filtering techniques and statistical validations, equips researchers to tackle quality challenges effectively.
- Software Solutions: Utilizing tools like FastQC and Trimmomatic can streamline quality assessments, making the process more efficient.
- Methodological Adjustments: Different studies may require tailored approaches in quality control to address specific challenges.
- Future Innovations: Keeping an eye on advancements in QC software ensures researchers are well-prepared for the evolving landscape of genomic data.
By grasping these takeaways, readers can better appreciate the intricate web of quality control within NGS workflows and its indispensable role in advancing genomic research.
The Role of NGS QC in Advancing Science
Quality control is not just a checkbox; it's a transformative practice that propels scientific discovery. Through stringent QC methods, researchers can uncover insights that were once clouded by ambiguous data. This leads to not only refined academic discussions but also impactful real-world applications. For example, in clinical genomics, where the stakes are exceptionally high, the reliability of genetic data directly affects patient outcomes and treatment strategies.
Even in environmental studies, the precision of genetic data can inform conservation efforts or ecological assessments that have far-reaching implications. Ultimately, the role of NGS QC is intertwined with the promise of scientific advancement.
"In the age of data, quality prevails over quantity. A single precise datum can eclipse a mountain of flawed information."