Machine Learning's Impact on Ad Targeting Strategies
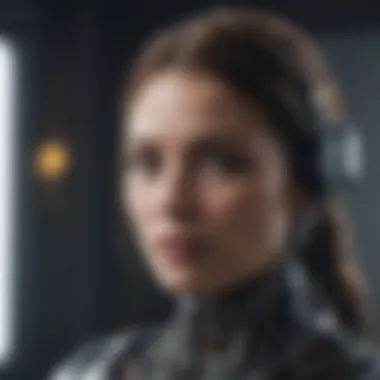
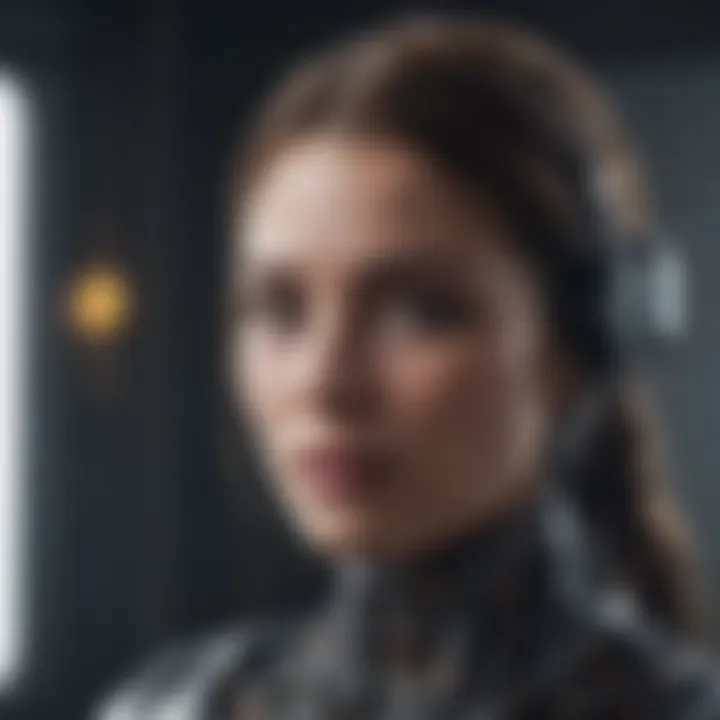
Intro
In an age where digital realms dictate much of our interactions, the realm of advertising finds itself at a pivotal juncture. The introduction of machine learning into ad targeting strategies has dramatically transformed how marketers reach potential customers. As algorithms evolve, they are not just refining the messages we see but also reshaping our understanding of visibility and engagement in the crowded landscape of media.
Understanding ad targeting through the lens of machine learning requires more than a superficial glance; it demands an engagement with its layers, intricacies, and implications. By illuminating how algorithms sift through mountains of data to predict user behavior, one gains insight into the core of modern advertising. The convergence of technology and marketing practices raises pivotal questions surrounding ethics, privacy, and the veracity of these complex systems.
Through this exploration, we aim to sketch out the fundamentals of how machine learning operates, while simultaneously addressing the broader societal issues that accompany its proliferation in advertising. What does it mean to be targeted? How deep does this aim extend, and what are its repercussions? These inquiries will guide our investigation into the dynamic interplay between machine learning and ad targeting, embarking on a journey that promises to shed light rather than obfuscate.
Prologue to Machine Learning in Advertising
In the ever-evolving world of advertising, machine learning has emerged as a key player, transforming how businesses connect with their audiences. The application of machine learning in ad targeting is not just a passing trend; it reflects a significant shift in how advertisers analyze data and predict consumer behavior. This development is vital, especially in an age where digital noise is ubiquitous, and capturing consumer attention is more challenging than ever.
Defining Machine Learning
When one thinks of machine learning in a marketing context, it’s essential first to clarify what is meant by machine learning. Essentially, it's a branch of artificial intelligence that empowers systems to learn from data, identifying patterns and making decisions with minimal human intervention. This learning process can range from straightforward algorithms that predict outcomes based on historical data, to more complex models that adapt over time as new data streams in.
The integration of this technology into advertising has substantially enhanced precision in targeting. Instead of relying solely on demographic data, advertisers now leverage a wealth of information, analyzing user behaviors, preferences, and even emotional responses. The result? Ads are not just shown to people based on what they might statistically fit into but rather tailored to resonate with individuals as they navigate the online landscape.
The Evolution of Ad Targeting
Looking back, the evolution of ad targeting paints a vivid picture of progress driven by technology. In the early days of advertising, targeting was rudimentary at best. Advertisers employed broad approaches, such as age brackets or geographic locations, believing that reaching a large audience was the ticket to success. However, as the digital revolution took hold, this one-size-fits-all method quickly became outdated.
With the rise of the internet and the increasing availability of user data, targeting tactics have undergone radical changes. Today, we see a more nuanced approach that blends traditional focusing methods with the sophisticated analytics made possible through machine learning. The shift began with basic web analytics and cookie tracking, evolving into intricate systems that scour data sourced from various platforms. Machine learning allows these systems to predict user affinities and behaviors based on their past interactions.
Now, advertisers can design campaigns that adjust in real-time, optimizing ad delivery according to the effectiveness of various strategies. This dynamic process ensures that ads reach the right people at the right time, enhancing both relevance and ROI.
"In advertising, machine learning is not just a tool; it represents a paradigm shift, allowing marketers to move from hindsight to foresight by actively engaging with consumer data."
With this continuous evolution, the landscape of advertising is poised for further transformation. As data becomes increasingly abundant and computational power expands, understanding the finer nuances of machine learning in ad targeting becomes not only beneficial but necessary for successful advertising strategies.
Core Concepts of Machine Learning
In the realm of advertising, the significance of understanding core concepts of machine learning cannot be overstated. By grasping these foundational principles, professionals can better leverage machine learning technologies to optimize ad targeting. The following concepts are paramount: the various types of machine learning algorithms, the nature of data sets, and how these elements come together to enhance advertising strategies.
Types of Machine Learning Algorithms
Supervised Learning
Supervised learning is a prominent type of algorithm that plays a crucial role in ad targeting. This method uses labeled data to train models, allowing them to learn patterns for predicting outcomes based on new input data. A key characteristic of supervised learning is that it relies on historical data with predefined labels, making it a choice favored by advertisers eager to predict user behaviors effectively.
The unique feature of supervised learning lies in its ability to provide clear insights into user preferences and behaviors. By analyzing past interactions, marketers can tailor their campaigns more finely. However, one disadvantage is that it requires a significant amount of labeled data, which may not always be readily available. Still, its effectiveness in generating actionable insights makes it a staple in the advertising toolkit.
Unsupervised Learning
Unsupervised learning shows its value when it comes to discovering underlying patterns and structures in data without the need for labeled inputs. Its main thrust is to identify clusters or group relationships among users. The key characteristic here is its ability to analyze vast amounts of user data to segment audiences dynamically based on behaviors rather than predetermined categorizations.
One unique aspect of unsupervised learning is its flexibility. It can adapt to new data, offering fresh insights that might not emerge in supervised models. However, it also comes with the challenge of interpretability; the outcomes are less predictable, which can leave advertisers in a bit of a fog regarding the best practices derived from such analysis. Nevertheless, its exploratory nature is invaluable in ad targeting strategies.
Reinforcement Learning
Reinforcement learning is another distinct type of machine learning that contributes significantly to ad targeting. Unlike the previous two, this approach involves training algorithms through rewards and penalties based on actions taken in a dynamic environment. This method is particularly beneficial in optimizing real-time bidding for online ad placements.
Its standout characteristic is the trial and error learning process, where the model gradually adjusts based on feedback received from its actions. This adaptability can lead to highly efficient ad placements that maximize return on investment. However, reinforcement learning can be resource intensive and time-consuming, as it typically requires sufficient time to converge to an optimal solution. Despite these challenges, its ability to dynamically adjust strategies makes it a powerful asset in the advertising landscape.
Understanding Data Sets
Data Collection
Data collection is a vital first step in the machine learning landscape of advertising. It involves gathering information from various sources, such as user interactions on websites or social media platforms. One important aspect of data collection is the sheer volume of data that can be amassed. This abundance allows machine learning models to function with a richer context about user behavior.
The uniqueness of data collection lies in its diverse methodologies, ranging from passive monitoring of user behavior to active surveys and engagement. However, it can pose challenges regarding data relevance and cleanliness. Proper collection practices ensure that the data used is representative and useful, making this an essential area of focus for machine learning implementations.
Data Labeling
Once data is collected, data labeling becomes essential, particularly in supervised learning contexts. This process assigns categories or tags to data points, allowing the algorithms to learn from labeled examples. The key characteristic of data labeling is that it transforms raw data into a structured format that can effectively teach models.
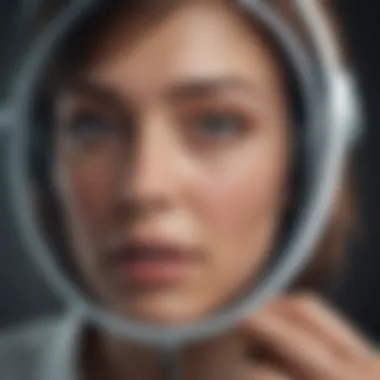
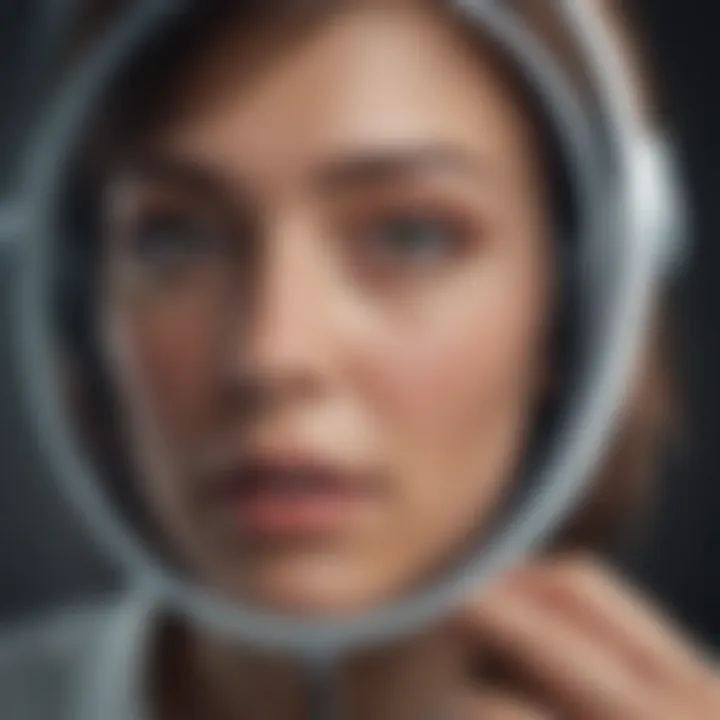
A unique feature of data labeling is the manual and sometimes laborious process it entails. Initial labeling can drive substantial returns in the machine learning space. However, it often requires constant updating and maintenance to reflect shifting behaviors and trends, turning it into a continuous effort.
Data Quality Considerations
Data quality is other critical element that can significantly influence the outcomes of machine learning in ad targeting. High-quality data—accurate, complete, and timely—enables algorithms to make trustworthy predictions. The key characteristic to focus on is that even the most sophisticated algorithms can yield poor results if fed with inferior data.
One unique advantage of prioritizing data quality is the resulting efficiency in training models. Cleaner data leads to reduced computational needs and faster iterations during model training. Conversely, low-quality data can introduce biases and errors, jeopardizing the efficacy of ad campaigns. Therefore, meeting data quality standards is paramount in achieving successful outcomes in machine learning-driven advertising.
Mechanics of Ad Targeting with Machine Learning
Understanding the mechanics that drive ad targeting through machine learning is crucial for grasping how contemporary advertising operates. Machine learning brings an analytical sharpness to ad targeting, allowing marketers to sift through vast datasets and derive actionable insights. By leveraging user data, businesses can create bespoke advertising experiences that resonate more deeply with individuals. This section details specific analytical approaches and personalization techniques that optimize user engagement and subsequently enhance ad performance.
User Behavior Analysis
User behavior analysis is at the heart of how machine learning optimizes advertising efforts. By scrutinizing how users interact with ads, companies can identify patterns that inform their targeting strategies. This not only streamlines the marketing process but also heightens user satisfaction by delivering relevant content at the right moment.
Click-Through Rates
Click-Through Rates (CTR) offer a clear picture of how often users engage with ads. This metric is significant for evaluating an ad's appeal. A high CTR suggests that an ad resonates well with its target audience, while a low rate may indicate that content isn't hitting the mark. Companies heavily rely on CTR because it's a direct reflection of user interest and engagement.
A unique feature of CTR is its ability to provide immediate feedback. Advertisers can quickly assess which ads draw clicks and which should be adjusted or scrapped. However, it’s important to remember that a high CTR doesn't necessarily guarantee conversions. Thus, while valuable, it's only one piece of the puzzle.
Conversion Tracking
Conversion tracking measures the effectiveness of ads by tracking specific actions users take after interacting with them. This aspect is essential for understanding the full impact of advertising efforts. By tracking conversions—such as purchases, sign-ups, or downloads—marketers can gauge the ROI of their campaigns.
What makes conversion tracking particularly appealing is the clarity it brings to advertising success. Advertisers can allocate budgets more effectively based on the performance of various ads. Yet, it does have a downside: tracking can sometimes lead to over-optimization, where marketers focus solely on short-term conversions, potentially neglecting long-term brand-building strategies.
Engagement Metrics
Engagement metrics provide insights into how users interact with both the ad content and the brand as a whole. These metrics measure time spent on the page, social media interactions, likes, shares, and comments. By analyzing engagement, companies can better understand audience interests and refine their advertising strategies accordingly.
The key characteristic of engagement metrics is their ability to reflect not just immediate interest, but also a longer-term connection with users. A high level of engagement suggests that users perceive value in the content. However, it's a double-edged sword; a focus solely on engagement might lead brands to chase trends rather than cultivate lasting relationships.
Personalization Techniques
Personalization techniques are essential to effectively tailoring ads to individual users, increasing relevancy and significantly enhancing user experience. Understanding audience segments and crafting messages for specific demographics is an art and science that pairs well with machine learning capabilities.
User Segmentation
User segmentation divides the target audience into distinct groups based on various criteria such as demographics, behaviors, or preferences. This segmentation is a powerful tool for marketers because it allows for customized messaging that speaks directly to the needs and interests of each group. By applying machine learning algorithms, advertisers can fine-tune their segments dynamically as new data becomes available.
The unique feature of user segmentation lies in its ability to create targeted ad strategies that resonate more effectively with specific audiences. However, over-segmentation can pose risks as it may lead to missed opportunities if potential customers fall outside established categories.
Content Recommendations
Content recommendations enhance ad targeting by suggesting personalized content to users based on their previous interactions. This method effectively nudges users toward additional products or services they may find appealing.
The benefit of content recommendations lies in their ability to create a seamless experience that keeps users engaged. However, an overload of recommendations can lead to user fatigue or irritation, potentially driving customers away rather than pulling them in.
Dynamic Creative Optimization
Dynamic Creative Optimization (DCO) is a strategy that involves generating and serving personalized ads in real time based on user data. This approach allows advertisers to automatically adjust and optimize ad content to better suit the viewer's preferences at that moment.
What sets DCO apart is its responsiveness—ad content can morph instantaneously based on factors such as user behavior, time of day, and even location. However, it requires a robust infrastructure and comprehensive data collection to be effective, which could limit its accessibility for smaller businesses.
In summary, the mechanics of ad targeting with machine learning represent a merging of science and creativity that revolutionizes how advertisers reach and engage their audiences. Through a careful analysis of user behavior and the application of personalized techniques, companies can craft campaigns that are not just effective but resonate deeply with consumers.
Impact of Machine Learning on Ad Effectiveness
Machine learning has revolutionized the advertising landscape, enhancing the effectiveness of ad targeting in numerous ways. It has shifted the focus from generic advertising strategies to highly personalized and targeted approaches. This precision is achieved through the utilization of algorithms that analyze vast datasets related to consumer behavior, preferences, and engagement patterns. Understanding the impact of machine learning on ad effectiveness is essential for advertisers looking to optimize their campaigns and achieve better returns on investment.
With machine learning, advertisers are not just reaching out to the masses but engaging the right individuals with the right messages at the right time. This paradigm shift enables brands to build stronger connections with their target audiences, leading to increased conversion rates and overall campaign success.
Moreover, as machine learning algorithms iterate and learn from interactions in real-time, they continuously optimize advertising strategies. This dynamic adjustment to user behavior can significantly enhance the effectiveness of campaigns, allowing advertisers to respond rapidly to trends and shifting consumer priorities. Let's delve into specific case studies that illustrate these principles in action and examine the metrics that underscore machine learning's contributions to ad effectiveness.
Case Studies of Successful Implementations
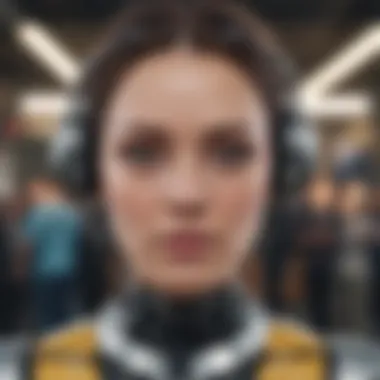
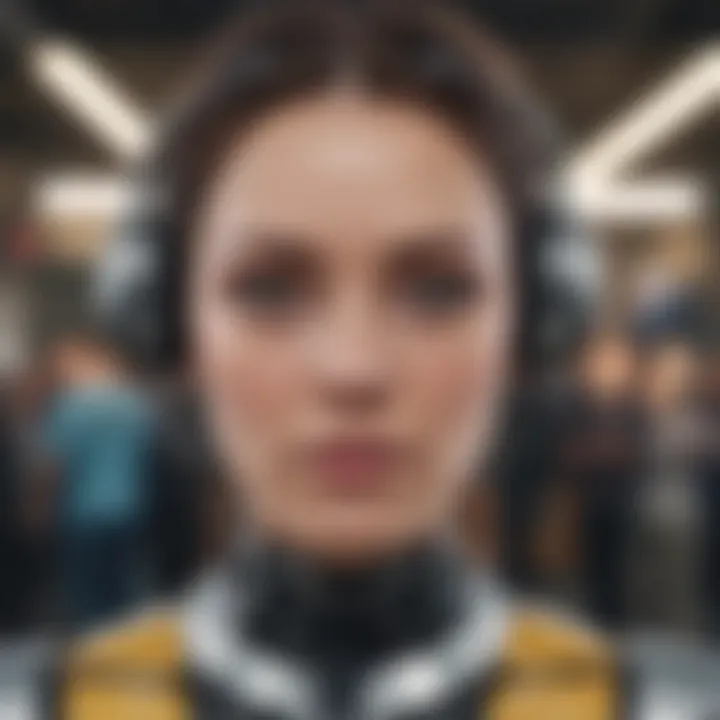
When we look at real-world applications, several case studies stand out, showcasing how different brands harness machine learning for ad targeting. One notable example is Netflix, which uses sophisticated algorithms to analyze user viewing habits. By understanding what genres or shows users engage with, Netflix personalizes recommendations effectively. This strategy doesn't just improve user satisfaction but also drives higher viewership and, consequently, retention rates.
Similarly, Amazon employs machine learning to analyze shopping behaviors. The targeted ads and recommendations based on previous purchases, browsing history, and wish lists have shown remarkable success in increasing sales volumes. This kind of tailored advertising strategy allows consumers to discover products they might not have found otherwise, underscoring the value of machine learning in ad targeting.
"Machine learning doesn’t just change how ads are targeted; it transforms the entire conversation between the brand and the consumer."
Measuring Return on Investment
To understand the true impact of machine learning on ad effectiveness, it is crucial for businesses to measure return on investment (ROI). Two key components in achieving this are Attribution Models and Key Performance Indicators.
Attribution Models
Attribution models play a critical role in determining how credit for conversions is assigned across various marketing channels. In a world where interactions with brands can occur over several touchpoints, these models provide clarity on which ads or interactions are driving results.
A popular choice among marketers is the Multi-Touch Attribution model. Unlike a last-click model, this method gives credit along the entire customer journey. This comprehensive view makes it easier to understand the nuances of user interactions and the effectiveness of various ads.
However, determining the right attribution model isn’t without its challenges. The complexity of data and variability in customer behavior can make it a daunting task. Marketers must weigh the advantages of detailed insights against the potential difficulties in accurately tracing every interaction.
Key Performance Indicators
Key Performance Indicators (KPIs) are metrics that help advertisers evaluate success against predefined objectives. In the realm of machine learning and ad targeting, KPIs like Click-Through Rates (CTR), Conversion Rates, and Cost Per Acquisition (CPA) are particularly significant. Each of these indicators sheds light on ad performance and helps marketers adjust strategies accordingly.
A standout KPI is Customer Lifetime Value (CLV), which estimates the total revenue a business can expect from a single customer over time. By applying machine learning models, companies can forecast CLV with greater accuracy and tailor their marketing efforts to maximize long-term profitability. Knowing how to interpret and utilize KPIs ensures that businesses can align their strategies with real outcomes, reaching deeper insight into how their ads are performing and where adjustments are needed.
In summary, the integration of machine learning into advertising practices is a game-changer in enhancing ad effectiveness. Through successful implementations and the ability to measure return on investment accurately, businesses can achieve optimized results and finely-tuned ad strategies.
Challenges and Limitations of Machine Learning in Ad Targeting
Machine learning has become a powerful ally in the realm of advertising, enhancing the ability to target the right audiences with precision. However, the technology is not without its obstacles. Understanding the challenges and limitations is crucial as they shape the ethical landscape and effectiveness of machine learning in ad targeting. As practitioners and researchers navigate this terrain, they must remain vigilant about the implications of these hurdles, particularly regarding data privacy and algorithm bias.
Data Privacy Concerns
GDPR Compliance
One major aspect here is GDPR Compliance, rolling out in the European Union to protect user data. The regulation establishes a strict framework for how personal information should be handled, impacting how marketers deploy machine learning. Its key characteristic is the requirement for explicit consent from users before processing their data. This aspect makes GDPR a noteworthy consideration, especially in this article, as it emphasizes the necessity of trust in digital advertising.
GDPR mandates a unique feature that businesses can’t simply harvest user data indiscriminately. They need to be transparent about what data they collect and how it's used. The advantages are clear, as it helps safeguard user privacy and can build consumer confidence. However, it can also present disadvantages like limiting the scope of targetable audiences, potentially hampering the effectiveness of machine learning algorithms. Marketers find themselves in a tricky situation, trying to comply while still wanting to hit their targets effectively.
User Consent
Moving beyond GDPR, User Consent is another vital concern that directly relates to how ads are tailored. User consent means that individuals must opt-in before their data can be used for ad targeting. The key characteristic of this feature is that it hinges heavily on transparency and user autonomy. This is a pivotal focal point in this article because consent represents both legal and ethical standards in advertising practices today.
The unique feature of User Consent empowers individuals to have a say in their personal data usage. It’s a significant step forward as consumers become more aware of their digital footprints. However, obtaining consent can also lead to lower data availability, which in turn can stifle the performance of machine learning models. It becomes a balance: while there are advantages in building respect and trust with users, the disadvantages might include challenges in gathering sufficient data for accurate targeting.
Algorithm Bias
Sources of Bias
Another critical component that adds complexity to our discussion is Algorithm Bias, which can adversely affect both targeting and consumer perception. Sources of Bias can stem from various places—training data being a primary one. If the data collected is skewed, it can lead to biased outcomes in ad targeting. A key characteristic of this is that bias often exists unwittingly, embedded in the very data sets we rely on.
Understanding the unique feature here brings to light that even well-intentioned machine learning models can perpetuate stereotypes or miss entire demographic groups if the training data isn’t adequately representative. Acknowledging these advantages and disadvantages surrounding bias is critical; while sophisticated algorithms can enhance efficiency, unchecked bias may lead to negative brand perceptions and reduced effectiveness of advertising efforts.
Mitigating Bias
Lastly, Mitigating Bias is essential for ensuring that machine learning systems operate fairly. The approaches to mitigating bias can include enhancing diversity in data sets and regular audits of algorithm outputs. A key characteristic of this effort focuses on continuous improvement and responsiveness to data inequalities. This is significant because it shapes not only how ads perform but also how brands are viewed in society.
Among the unique features of bias mitigation is its dual approach—it’s about refining algorithms while also creating awareness amongst designers and marketers. The advantages lie in establishing a reputation for inclusivity and fairness, which resonates well with consumers today. But, on the flip side, the disadvantages involve the additional resources required to implement such strategies, potentially slowing down deployment times for ad campaigns.
In summary, as machine learning continues to revolutionize ad targeting, navigating these challenges and limitations becomes a delicate dance, impacting both the technical execution and ethical standing of advertising practices. As the future unfolds, keeping a close eye on these aspects will prove paramount for all stakeholders involved.
Ethical Considerations in Machine Learning Ad Targeting
As machine learning increasingly becomes central to advertising, the question of ethics is an unavoidable topic. It's not just about making things work; it's also about making them work right. In ad targeting, algorithms sift through vast amounts of data to predict what product or service a user might be interested in. However, this capability brings with it significant ethical concerns that need to be navigated thoughtfully.
The stakes are high. With data collection at the core of machine learning, issues around privacy, consent, and data usage become hot-button topics. Companies leveraging these algorithms must tread carefully to avoid trampling on user rights while still reaping the benefits of hyper-targeted advertising.
Balancing Effectiveness with Ethics
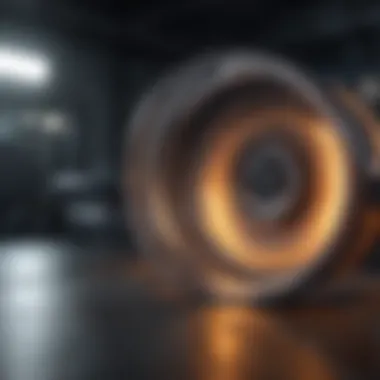
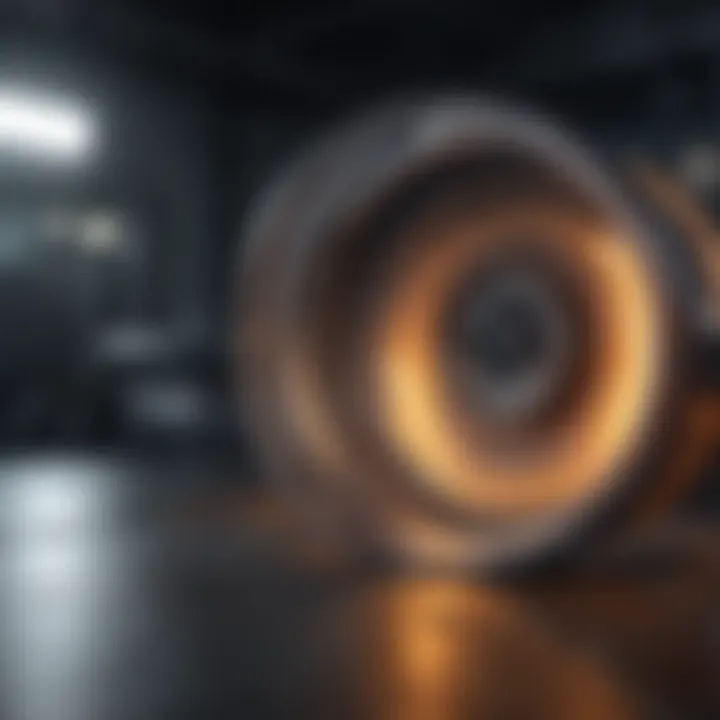
In this digital landscape, a delicate balance must be maintained. On one hand, businesses need to harness the power of machine learning to create effective advertising strategies that drive growth and engagement. On the other hand, they must commit to ethical practices that protect user data and maintain consumer trust. This balancing act is crucial.
One pertinent example involves personalized ads. While targeted advertisements can lead to higher conversion rates and sales, they also risk being perceived as invasive. Users may feel like they are under constant surveillance, leading to backlash against brands that cross ethical boundaries. Therefore, crafting a comprehensive and transparent user consent process is essential. This can ensure users know how their data is being used and reassure them that they have control over their information.
Regulatory Frameworks
Current Regulations
Current regulations like the General Data Protection Regulation (GDPR) in Europe have profoundly shaped the landscape of data usage in advertising. The GDPR lays down strict rules on how personal data should be collected, stored, and utilized. Its key characteristic is its heavy emphasis on user consent and transparency, which resonates with the growing demand for privacy in today’s data-driven environment.
The benefit of adhering to these regulations is twofold. First, companies that comply avoid heavy fines and potential legal issues. Second, they cultivate trust among users. People are more likely to engage with a brand that openly communicates its data practices. This transparent approach will likely yield a loyal customer base, providing a long-term competitive edge. However, adherence to such regulations can also slow down the speed at which companies can implement new advertising strategies, presenting a unique challenge for marketers.
Future Directions
Looking forward, the landscape of regulatory frameworks is likely to evolve. Future directions in compliance may focus on expanding the scope of regulations to better protect user data globally. This could mean stricter oversight of how algorithms operate and increased accountability for advertisers.
One noteworthy aspect of these potential future regulations is their proactive nature. Instead of merely responding to breaches or misuses of data, they could establish stricter guidelines that prevent ethical dilemmas from arising in the first place. The benefit here is creating a more secure data environment for users, fostering a healthier relationship between businesses and consumers. Yet, this could also lead to increased operational costs and compliance burdens for companies, as they adjust to newly established prerequisites.
"As the world of advertising continues to evolve, so too must our understanding of the ethical implications and the regulations surrounding it."
Future Trends in Machine Learning for Ad Targeting
As the digital landscape evolves, advertisers are compelled to keep pace with the rapid developments in machine learning. Understanding future trends is not just a matter of staying relevant; it’s essential for leveraging the full potential of ad targeting strategies. Machine learning is reshaping how ad campaigns are executed, leading to more efficient allocation of resources, which ultimately boosts return on investment (ROI). Among the trends to watch closely are advancements in AI technologies and the pivotal role of big data, both of which underpin the future of ad targeting.
Advancements in AI Technologies
The continual refinement of AI technologies is perhaps the most discreet yet profound driver of change in advertising. Machine learning models are evolving at breakneck speed, improving performance and decision-making capabilities. Suppliers are adopting models that not only predict user behavior but also learn in real time, adapting to shifts in consumer sentiment, preferences, and behavior. For instance, Natural Language Processing systems are now capable of analyzing user-generated content much more effectively, allowing brands to tailor their messaging strategies based on what resonates best with their audience.
Moreover, the integration of generative models can create unique ad content on-the-fly, adjusting visual stimuli to cater to real-time user reactions. This kind of responsiveness significantly enhances engagement metrics, making users feel more connected with the brands they interact with. In a nutshell, advancements in AI technologies are streamlining processes and ensuring that advertising remains highly relevant to consumers' evolving needs.
The Role of Big Data
Big data represents a goldmine for advertisers looking to refine their targeting efforts. The sheer volume of data available—from click patterns to social media interactions—allows for granular insights into consumer behavior. This information is crucial for creating effective ad strategies, as it informs everything from audience segmentation to content optimization.
Data Integration
Data integration stands out as a critical component within the big data realm. It involves consolidating various data sources into a coherent dataset that can be analyzed comprehensively. By synthesizing data from disparate agegories—such as web analytics, customer feedback, and transaction records—organizations can create a holistic view of their target audience. This unified approach enhances data correlation and leads to more informed decision-making.
One key characteristic of data integration is its scalability; as businesses grow, their data needs expand. Having a solid integration strategy in place allows for seamless incorporation of new data points without disrupting existing workflows
However, while data integration is often advantageous, it's not without its challenges. Ensuring data quality and consistency is paramount. If data from different sources conflicts or is inaccurate, it can lead to misguided strategies and decisions.
Real-Time Processing
Real-time processing also plays a pivotal role in how big data is harnessed in ad targeting. This technology enables advertisers to analyze data streams instantaneously, facilitating timely responses to consumer behaviors and trends. Take, for instance, the ability to adjust ad placements in response to live browsing behavior; this shifts the paradigm from hypothetical predictions to actual user actions.
The value of real-time processing lies in its immediacy. Advertisers can capitalize on fleeting opportunities to engage their audience effectively. Imagine a consumer who’s shown interest in hiking gear; with real-time processing, brands can serve tailored ads just as that consumer is researching hiking trails.
However, this technology also brings its complications. The need for robust infrastructure to handle vast data loads can strain resources and budgets—staying on top of the technological capabilities necessary to trace user behavior in real time is key to leveraging its full potential.
"The next era of advertising will be driven by precision and relevance, rooted in intelligent systems that understand consumers deeply."
Culmination
In wrapping up this exploration of machine learning's role in ad targeting, it's essential to understand its profound significance in shaping contemporary advertising landscapes. Not only do these technologies enhance precision in reaching target audiences, they also directly impact the consumer experience.
The integration of machine learning algorithms allows advertisers to harness vast amounts of data effectively. This streamlining of information leads to more relevant advertisements, fostering user engagement. Precise targeting can result in improved click-through rates, which directly correlate to better return on investment for brands. This kind of targeted outreach, however, must be balanced with considerations of data privacy and ethical practices.
Machine learning, while a boon in optimizing ad strategies, poses questions about algorithm bias and user consent. As we navigate these complexities, it becomes clear that understanding this technology is not just necessary for marketers but essential for any stakeholder involved in the advertising ecosystem.
Summarizing Key Insights
As we reflect on the myriad points discussed throughout this article, several key insights emerge:
- Data-Driven Decisions: The effectiveness of machine learning in ad targeting hinges on the ability to analyze vast data sets for informed decision-making.
- User Experience Enhancement: Targeted advertising improves user experience by pushing relevant products, which can lead to higher satisfaction and loyalty.
- Privacy Considerations: With increased effectiveness comes heightened scrutiny over privacy, signaling the need for transparent practices in data usage.
- Ethical Implications: Bias in algorithms must be addressed to ensure fairness and inclusivity in advertising.
Looking Ahead
Looking to the future, the dynamics of machine learning in ad targeting promise ongoing evolution and innovation. Here are some considerations for what lies ahead:
- Continued Technological Advancements: As AI technologies progress, advertisers will harness even more sophisticated algorithms, refining targeting strategies.
- Real-Time Data Processing: The capability to process data in real time will allow for hyper-personalized ads that respond instantaneously to user behavior.
- Regulatory Landscapes: With shifting regulations concerning data privacy, the advertising industry must adapt practices to align with new laws while still achieving marketing goals.
In summary, the future of machine learning in advertising appears bright yet complex. Keeping abreast of these changes and ethical considerations will be paramount for anyone looking to thrive in this digital marketing era.