Machine Learning Revolutionizing Material Science
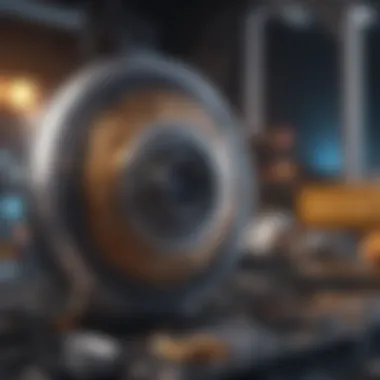
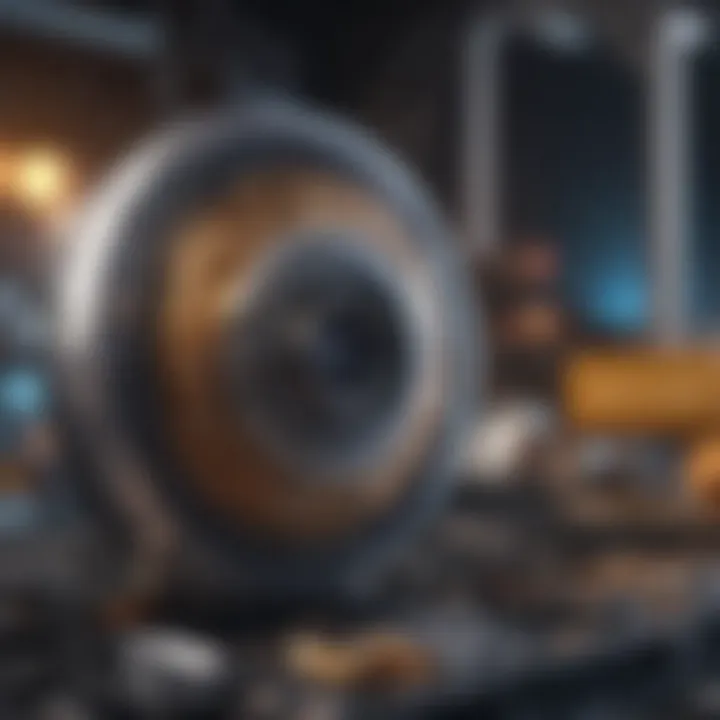
Intro
The integration of machine learning into material science represents a transformative shift in how materials are developed and understood. By leveraging the computational power of algorithms, researchers can unlock patterns within complex datasets that were previously challenging to analyze. This intersection marks a revolution for material discovery, leading to innovations across industries from aerospace to nanotechnology.
Research Background
Overview of the Scientific Problem Addressed
Material science faces numerous challenges, particularly in the areas of material selection, optimization, and development. Traditionally, experimentation relied on a labor-intensive process, often yielding results after extensive trial and error. Machine learning aims to expedite this process by utilizing existing data to predict material properties and behavior, fundamentally changing how researchers approach material design.
Historical Context and Previous Studies
Historically, material science has relied heavily on empirical methods and intuition. Basic theories from physics and chemistry provided a framework for understanding materials. Early studies began to integrate statistical methods and computational models in the late 20th century. However, it wasnβt until the rise of machine learning in the 21st century that the true potential of algorithmic approaches emerged.
Notably, researchers like Jha and Ward have pioneered work that connects machine learning algorithms with large databases of materials. This combination enhances the predictive capabilities of researchers, shifting focus from isolated experiments to a broader analytical approach.
The pioneering work surrounding the Materials Project exemplifies how data-driven strategies can lead to meaningful discoveries in material science.
Findings and Discussion
Key Results of the Research
Recent findings indicate that machine learning can significantly reduce the time and cost associated with material discovery. Techniques such as supervised learning, neural networks, and support vector machines have shown promise in accurately predicting material properties. By training algorithms on existing data, researchers can identify promising new materials more rapidly.
Interpretation of the Findings
The integration of machine learning leads to an increased understanding of materials at a microstructural level. An important aspect of this is how analytical methods enable the extraction of insights from complex datasets. For instance, using convolutional neural networks, researchers can analyze images of materials to predict their features and functional behaviors accurately.
"The future of material science may rest less on the intuition of scientists and more on the computations of machines."
As researchers adopt these technologies, they face both opportunities and challenges. The opportunity lies in the ability to create materials tailored for specific needs through advanced predictive modeling. However, ethical concerns and the need for interpretability in AI-driven results pose important questions for the community.
Prelims to Machine Learning in Material Science
The integration of machine learning into material science provides significant advancements in the way materials are studied, discovered, and utilized. This intersection not only enhances research efficiency but also opens op new avenues for innovation in the field. Understanding this relationship is crucial for students, researchers, and professionals eager to stay ahead in a rapidly evolving landscape.
Definition of Machine Learning
Machine learning refers to the use of algorithms and statistical models that enable computers to perform tasks without explicit instructions. By leveraging large data sets, machine learning systems can identify patterns, make predictions, and improve their performance over time. This iterative process allows for more accurate analyses in material science than traditional methods.
There are various types of machine learning, including supervised, unsupervised, and reinforcement learning. Each type has unique characteristics and applications that contribute to the advancement of the field. Supervised learning uses labeled data for training, whereas unsupervised learning identifies patterns without predefined labels. Reinforcement learning focuses on evaluating the outcomes of actions to enhance decision-making.
Overview of Material Science
Material science is the study of materials and their properties, structure, performance, and applications. It encompasses aspects from physics, chemistry, and engineering to understand how materials behave under various conditions. This field is instrumental in developing new materials, improving existing ones, and innovative applications in technology and industry.
Research in material science often requires extensive experimentation and computational modeling. However, these traditional approaches can be time-consuming and costly. Thus, the integration of machine learning can streamline processes, leading to faster discovery and optimization of materials
Significance of Their Integration
The combination of machine learning and material science holds substantial promise for enhancing research output. This integration allows for:
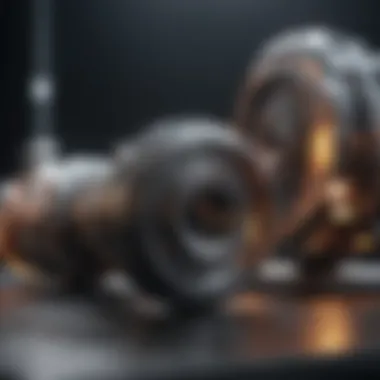
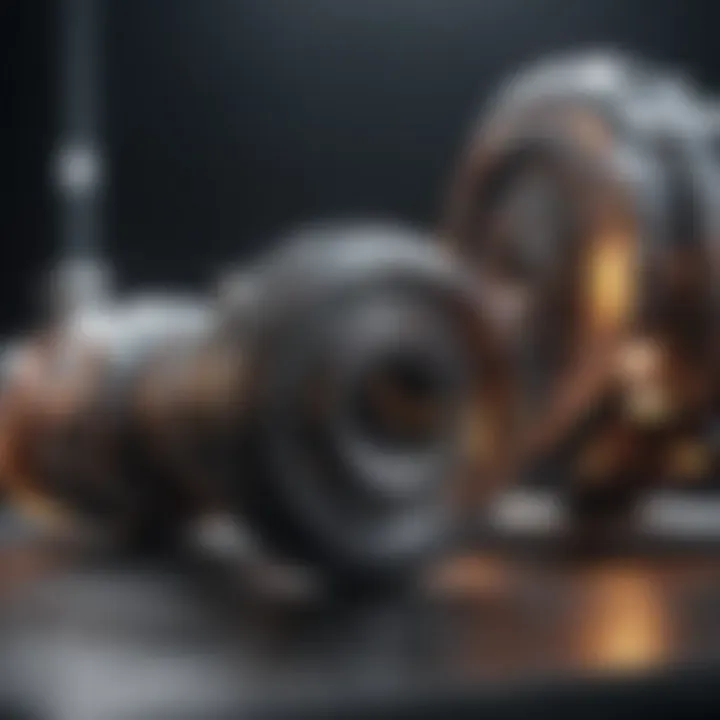
- Accelerated Data Analysis: Machine learning algorithms process large data sets quickly, freeing researchers to focus on interpretation and application.
- Enhanced Predictive Modeling: Predictive models can help forecast material properties and performance based on past data, thus reducing trial and error in material design.
- Discovery of New Materials: Automated discovery approaches can identify novel materials, pushing the boundaries of what is possible in various applications.
"Integrating machine learning into material science empowers researchers to capitalize on vast amounts of data, paving the way for innovative discoveries and applications."
Key Concepts in Machine Learning
Understanding machine learning is essential for its application in material science. The integration of these two areas is becoming increasingly relevant as it allows for innovative solutions and improved efficiency in research processes. Machine learning encompasses various concepts that provide the foundation for its application in material science. This section will delve into these key concepts: supervised learning, unsupervised learning, and reinforcement learning. Each concept plays a unique role in how data is analyzed and predictions are made.
Supervised Learning
Supervised learning is a process where a model is trained using labeled input data. This means that the dataset used provides both the input features and the corresponding output labels. The model learns to map inputs to outputs, which can be employed in applications such as predicting material properties. The primary advantage of supervised learning is its ability to make accurate predictions once trained adequately. Examples include predicting tensile strength from composition data or estimating conductivity based on temperature.
A key consideration in supervised learning is the quality of the training data. If the data is not comprehensive or representative, the model's performance will be compromised. Therefore, obtaining high-quality, labeled datasets is crucial. In material science, this could involve extensive measurements and tests to build a reliable dataset.
Unsupervised Learning
Unsupervised learning differs significantly from supervised learning in that it deals with data that does not have labels. The goal here is to find hidden patterns or intrinsic structures in the input data. This can be particularly useful in material science for tasks such as clustering materials with similar properties or reducing dimensionality in large datasets.
One application of unsupervised learning is in the analysis of material microstructures. By examining images of materials, unsupervised algorithms can identify various phases present in a sample. This can lead to better understanding how different phases affect overall material behavior. Although it is less straightforward than supervised learning, its ability to uncover insights without predefined labels can yield valuable information in research and development.
Reinforcement Learning
Reinforcement learning is based on the idea of learning through interaction with an environment. The model, referred to as an agent, takes actions to maximize some notion of cumulative reward. In material science, reinforcement learning can optimize processes by systematically exploring different parameters and learning from the outcomes.
For instance, it can be used to tailor material compositions or processing parameters to achieve desired characteristics. By simulating various scenarios, the algorithm learns which actions yield the best results. This iterative process can lead to more efficient material designs and innovations.
"Machine learning not only enhances predictive accuracy but also transforms our approach to knowledge discovery in materials science."
By comprehensively grasping these fundamental concepts, students, researchers, and professionals can harness machine learning effectively to solve complex problems in material science.
Data Types and Sources in Material Science
Understanding the different data types and sources in material science is crucial for leveraging machine learning effectively. The integration of these disciplines significantly relies on the quality and variety of data available. With accurate data, researchers can train algorithms to predict material properties, optimize processes, and discover new substances. In this section, we will dive into three key types of data used in material science: experimental data, computational models, and database utilization.
Experimental Data
Experimental data is foundational to material science. This type of data comes from laboratory experiments that assess the physical and chemical properties of materials. It could include various measurements such as tensile strength, thermal conductivity, and fracture behavior. The value of experimental data lies in its reliability; it reflects real-world behaviors that are crucial for validating theoretical models. Moreover, machine learning systems thrive on high-quality experimental data to ensure accurate predictions. However, collecting such data can be labor-intensive and time-consuming, making access to well-documented datasets vital.
Computational Models
Computational models serve as another essential source of data. These models simulate material behavior using mathematical representations. For instance, density functional theory (DFT) and molecular dynamics are commonly used to predict how materials will perform under different conditions. By generating synthetic data, computational models can fill gaps where experimental data might be insufficient. However, while computationally derived data is valuable, it also needs to be correlated with experimental findings to verify its accuracy and applicability.
Database Utilization
Database utilization is a modern approach for managing and accessing vast amounts of data generated in material science. Numerous databases exist, such as the Materials Project and the AFLOW database, which aggregate material properties from various sources. These databases provide an easily accessible platform for researchers to explore existing data, thus facilitating comparisons and aiding in the training of machine learning algorithms. Utilizing these resources allows scientists to leverage historical data effectively, driving forward research and reducing the redundant work of gathering similar data.
"A critical aspect of advancing material science is not just the data itself but how effectively it is integrated and utilized within machine learning frameworks."
In summary, understanding data types and sources is integral to the effective application of machine learning in material science. Experimental data provides the foundation, computational models offer simulations, and databases organize and streamline access to essential information. Together, these elements enhance the analysis and discovery of new materials.
Machine Learning Algorithms Applied to Materials Science
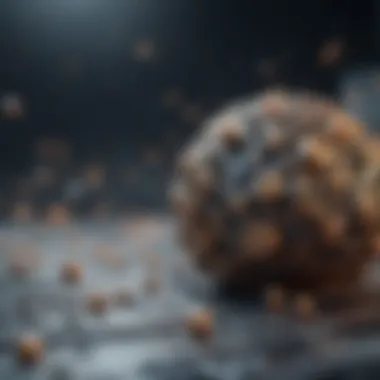
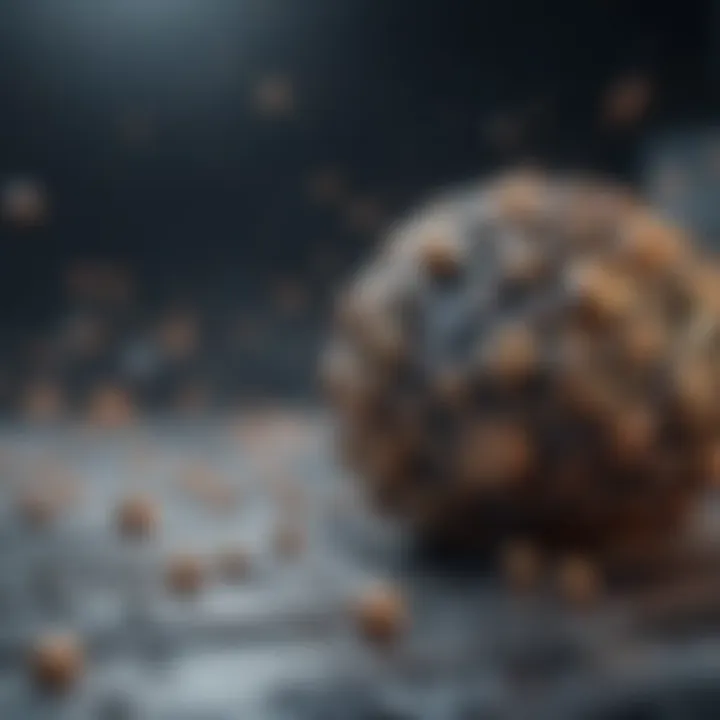
Machine learning algorithms are critical in the realm of material science, primarily due to their capability to analyze vast datasets and uncover patterns that may not be immediately visible to human researchers. These algorithms facilitate the discovery of materials with desirable properties and optimize processes, which can lead to significant advancements in various sectors, including manufacturing, electronics, and nanotechnology. With ongoing developments in machine learning, researchers can expect increased accuracy in predictions and more efficient workflows. The integration of these algorithms opens up new avenues for exploration, allowing scientists to tackle complex problems that traditional methods may struggle to resolve.
Regression Techniques
Regression techniques are vital tools in predictive modeling. They allow researchers to estimate the relationships between variables and predict outcomes from input data. In material science, regression models can be used to correlate material properties to their compositions or processing conditions. Highly relevant examples include linear regression and polynomial regression, which are employed to model the tensile strength of alloys based on their chemical composition.
Using regression techniques enables:
- Assessing how different elements influence material characteristics.
- Making precise predictions about new material formulations.
- Decreasing the time required for experimental testing.
Nonetheless, the efficacy of regression models largely depends on the quality of the data being input. Unreliable or sparse data can lead to flawed predictions, which may affect the material's performance.
Classification Algorithms
Classification algorithms play a crucial role by categorizing materials based on learned features from datasets. They are essential for applications such as identifying suitable candidates for specific applications or grouping materials by performance metrics. Common algorithms such as decision trees and support vector machines excel in these scenarios.
Benefits of classification algorithms include:
- Ability to efficiently analyze complex datasets.
- Enhanced speed in discovering new materials with required features.
- Facilitating databases that classify materials for easy access.
Despite their advantages, careful consideration must be given to the algorithm selection, as the wrong choice may yield misleading classifications. It is essential to continuously refine models with new data to ensure high accuracy.
Neural Networks
Neural networks represent a finding frontier in machine learning applications within materials science. These algorithms, which mimic the way the human brain operates, excel in recognizing intricate patterns within large datasets, making them particularly suitable for predicting material behaviors under various conditions. Convolutional neural networks, for instance, have shown promise in analyzing microstructures and predicting their mechanical properties.
Key aspects of neural networks include:
- Their ability to process unstructured data, such as images or text, directly.
- The potential to uncover non-linear relationships that traditional models may overlook.
- Scalability, allowing for their application to increasingly complex problems as more data becomes available.
However, the designed neural network must be optimized to avoid issues like overfitting. Adjustments to model architecture and training methods can drastically impact performance.
Machine learning algorithms, including regression techniques, classification algorithms, and neural networks, empower material scientists to innovate and optimize material development processes.
In summary, machine learning algorithms have a transformative impact on materials science. Each algorithm has unique advantages and considerations that researchers must understand to effectively apply these tools. As the intersection of these fields continues to evolve, the potential for groundbreaking discoveries increases, underscoring the importance of ongoing research and refinement.
Case Studies of Machine Learning in Material Science
Case studies serve as vital references in the integration of machine learning and material science. They provide concrete examples of how theoretical concepts manifest in real-world applications. Not only do they showcase successful implementations, but they also reveal the process and decisions taken during research. These case studies help bridge the gap between fundamental research and practical applications. They offer insights into the optimization of materials and contribute to advancing knowledge in the field.
Predictive Modeling of Alloy Properties
Predicting alloy properties is a complex task, given the multitude of factors that influence material behavior. Machine learning techniques have shown great promise in this area. By analyzing existing data on alloy compositions and their corresponding properties, algorithms can establish patterns that traditional methods might overlook. For example, a study utilizing random forest algorithms analyzed hundreds of alloy compositions to predict tensile strength with remarkable accuracy. The benefit of predictive modeling is evident; researchers can quickly identify promising new alloys without extensive experimental work.
Optimization of Polymer Characteristics
Polymers exhibit unique properties that can be tailored through processing and formulation. Machine learning connects the dots between polymer chemistry and physical properties. In one significant case, researchers employed support vector machines to optimize the mechanical properties of polyethylene materials. The machine learning model analyzed numerous experimental data points, enabling the team to determine optimal processing conditions efficiently. This application helps in producing materials that meet specific performance criteria while reducing time and cost.
Discovery of New Materials
The discovery of new materials is essential for innovation, yet it often requires extensive experimentation. This is where machine learning excels. Algorithms can sift through vast data sets to identify potential candidates for new materials. In a recent project, convolutional neural networks were used to analyze properties of existing materials to discover new semiconductors. The results led to identifying several new compounds that had not been previously considered. This approach not only accelerates the pace of discovery but also lowers the risk associated with material experimentation.
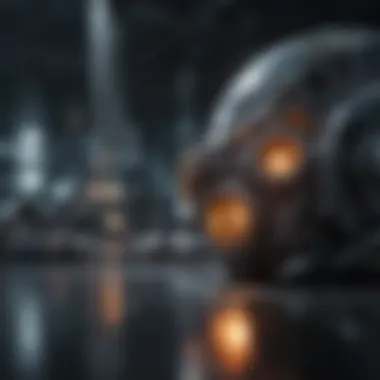
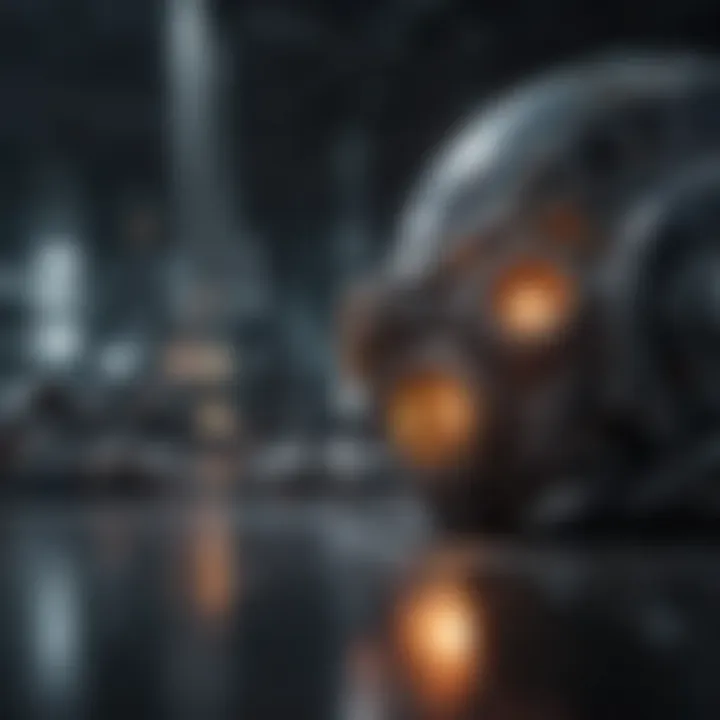
"Machine learning transforms the landscape of material science, shifting the focus from trial and error to data-driven exploration."
Through case studies such as these, it is evident that machine learning enhances the capabilities within material science. Advances in predictive modeling, optimization, and discovery methods illustrate a future where material innovation is revolutionized by data.
Challenges in Implementing Machine Learning in Material Science
The integration of machine learning in material science offers great promise, but it is not devoid of challenges. These hurdles need to be systematically addressed to fully leverage machine learning for advancements in materials innovation. Key areas of focus include data quality and availability, model interpretability, and the integration of machine learning with traditional methods. Understanding these challenges is crucial for researchers and practitioners who aim to utilize machine learning effectively.
Data Quality and Availability
Data quality plays a paramount role in the effectiveness of machine learning models. Poor-quality data can lead to inaccurate predictions and flawed analysis. The materials science community faces a challenge with the availability of high-quality datasets. A common issue is the inconsistency of data due to variations in experimental conditions or measurement techniques. Furthermore, the need for large datasets to train robust machine learning models often exposes the scarcity of comprehensive databases.
To tackle these challenges, researchers must prioritize the standardization of data collection and storage. Collaborative efforts to build open-access databases can enhance data sharing. This will facilitate more effective machine learning applications. Institutions such as Materials Project and the National Renewable Energy Laboratory are examples of efforts being made to make high-quality datasets available to the community.
Model Interpretability
Model interpretability is another significant challenge when applying machine learning in material science. Many advanced machine learning models, such as deep neural networks, often function as black boxes. They can provide predictions, but understanding how they arrive at these decisions is often unclear. This lack of transparency can hinder the trust of scientists in machine learning outputs, particularly in critical applications like material development and safety evaluations.
To overcome issues regarding interpretability, researchers have been developing methods to elucidate the reasoning behind machine learning predictions. Techniques such as SHAP (SHapley Additive exPlanations) and LIME (Local Interpretable Model-agnostic Explanations) are essential in making complex models more interpretable. By employing these techniques, scientists can gain insights into model behaviors, leading to greater confidence in the proposed solutions.
Integration with Traditional Methods
The successful integration of machine learning with traditional materials science methodologies presents unique challenges. Often, established techniques have served researchers for decades, and transitioning to more data-driven approaches requires adaptation and a cultural shift within the scientific community.
Some traditional approaches emphasize experimentation and theoretical modeling, while machine learning relies heavily on data-driven insights. Bridging this gap involves not only technical challenges but also educational ones. Researchers need to be trained in both fields to navigate this intersection effectively. Furthermore, hybrid approaches, which combine machine learning with conventional methods, may offer a balanced way forward. Utilizing machine learning to enhance traditional methods could lead to a more comprehensive understanding of material behaviors.
Future Directions in the Field
The integration of machine learning with material science is still in its nascent stages, yet it holds enormous potential for transformation. As research progresses, understanding future directions becomes paramount. This section aims to discuss advancements in algorithms, interdisciplinary approaches, and the role of artificial intelligence in fostering innovations in materials.
Advancements in Algorithms
Advancements in algorithms are critical for enhancing the capabilities of machine learning in material science. New methods like deep learning and ensemble techniques are being perfected to deal with complex datasets. Researchers are focusing on optimizing algorithms that can better predict material properties and behaviors. For instance, gradient boosting and support vector machines have shown significant promise in predictive modeling tasks.
Additionally, the development of generative models allows scientists to not only predict outcomes but also discover new materials. By utilizing algorithms that can simulate and predict experimental outcomes, researchers can reduce the trial-and-error process typically associated with material design. Moreover, active learning techniques are emerging, enabling machines to select the most informative experiments, thereby improving efficiency and effectiveness in research.
Interdisciplinary Approaches
Interdisciplinary approaches are essential to harness the full potential of machine learning in material science. Collaborations between chemists, physicists, computer scientists, and engineers stimulate innovation and enrich the research environment. By combining expertise from various fields, researchers can tackle challenges more effectively.
For example, material informatics merges data science approaches with material discovery, allowing for a deeper understanding of the relationships between material structure and properties. Cross-disciplinary education programs are also gaining traction, equipping professionals with diverse knowledge and skills. This convergence promotes a holistic perspective that can lead to groundbreaking discoveries in material science through machine learning applications.
Role of AI in Materials Innovation
Artificial intelligence is set to play a transformative role in material innovation. AI technologies facilitate high-throughput screening of materials, dramatically speeding up the discovery process. For instance, researchers utilize AI to analyze extensive datasets generated from simulations and experiments. This approach enables faster identification of candidate materials for specific applications.
Moreover, AI aids in the optimization of existing materials. Machine learning models can predict how various environmental conditions impact material performance, guiding improvements in design and manufacturing processes. As AI continues to evolve, its ability to innovate and enhance materials should expand significantly.
Epilogue
The conclusion serves as a crucial component of this article, providing a succinct synthesis of the main concepts discussed throughout. This section ties together the intricate relationship between machine learning and material science, emphasizing their collective potential to drive innovation and enhance research methodologies. As machine learning algorithms continuously evolve, their integration into material science holds significant promise for advancing our understanding and capabilities in this field.
Summary of Key Points
- Integration benefits: Machine learning offers powerful tools to analyze vast datasets, improving material discovery and optimization processes.
- Key algorithms: Various machine learning algorithms have been discussed, such as regression techniques, classification algorithms, and neural networks, each with unique applications in material science.
- Case studies: Real-world applications showcased predictive modeling of alloy properties, optimization of polymer characteristics, and the discovery of new materials, demonstrating successful integration.
- Challenges: Addressing data quality, model interpretability, and the need for integration with traditional methods remain significant hurdles to overcome as the fields continue to merge.
- Future directions: Ongoing advancements in algorithms and interdisciplinary approaches suggest a pathway for substantial progress in materials innovation.
Final Thoughts on the Integration
The integration of machine learning into material science is not simply a trend; it represents a fundamental shift in how research can be conducted. The capacity of machine learning to uncover patterns within complex data sets accelerates the pace of discovery and enhances our ability to tailor materials to specific applications. However, achieving this potential requires careful consideration of both the opportunities and challenges involved in implementation. Future research will benefit from a continued focus on improving data quality, enhancing model transparency, and fostering collaboration between computational scientists and traditional material scientists. As these fields overlap, they will likely yield innovative breakthroughs that could redefine material applications across various industries.