Exploring Probability Learning: Concepts and Implications
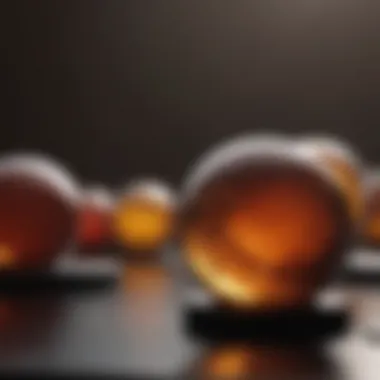
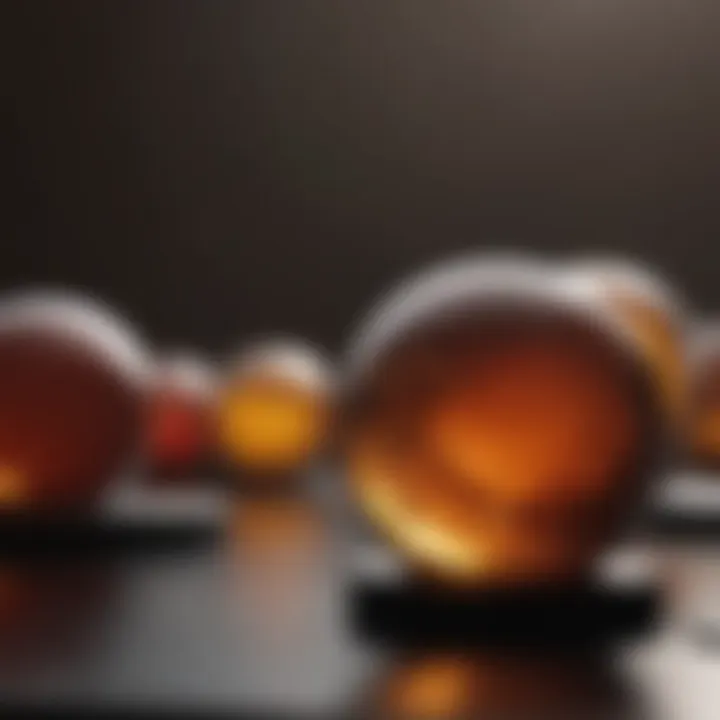
Intro
In an unpredictable world, understanding how we assess risks and probabilities is essential. Probability learning serves as a pivotal mechanism, guiding decisions in everyday life—from the simple act of betting on a horse to the complex algorithms that predict consumer behavior. This exploration aims to unravel the layers of this intricate process. By grasping its facets, we can better appreciate its significant impact across multiple disciplines such as psychology, machine learning, and data science.
Research Background
Overview of the Scientific Problem Addressed
Probability learning centers on an individual's ability to evaluate likelihoods. It's not just about having the numbers; it's about how we internalize these probabilities and apply them to real-world situations. The challenge lies in understanding the cognitive processes that underlie this learning and decision making. For instance, how do individuals weigh past experiences against new information when faced with uncertainty?
Historical Context and Previous Studies
The concept of probability has evolved over centuries. Early philosophers like Pascal and Fermat laid the groundwork for probabilistic thought, while modern psychologists have explored how cognitive biases affect our understanding of chance. By the turn of the 20th century, studies began to emerge that scrutinized how humans estimate probabilities, revealing that our intuitions often lead us astray. For example, the phenomenon known as the "gambler's fallacy" illustrates how people erroneously believe that past events influence future probabilities in random sequences.
Evolution of Theoretical Frameworks
Several theoretical models have emerged to explain how we learn probabilities. Bayesian reasoning, for example, emphasizes updating beliefs based on prior knowledge. In contrast, frequentist approaches focus on the long-run frequency of events. Understanding the nuances between these frameworks is crucial not just for academia but also for practical applications in fields such as finance and healthcare.
Understanding these historical contexts lays the foundation for contemporary applications. Researchers continue to refine theories and conduct experiments that deepen our grasp of how probability learning shapes behavior. This research might influence how we approach decision-making, whether in clinical settings or artificial intelligence models.
Findings and Discussion
Key Results of the Research
Recent studies have shown that probability learning is influenced by various factors, such as cognitive load and emotional state. For instance, individuals under stress may rely on heuristic shortcuts rather than thorough analysis. This has implications for sectors like marketing, where understanding consumer behavior can dictate strategies.
Moreover, advancements in data science allow for more sophisticated modeling of probability learning processes through machine learning techniques. Utilizing vast datasets, researchers can observe patterns that inform predictions about human behavior.
Interpretation of the Findings
The ability to learn probabilities is not merely an academic pursuit; it has real-world ramifications. In psychology, understanding how we make decisions under uncertainty can lead to improved therapeutic strategies. For data scientists, refining models to account for these human factors enhances their predictive accuracy.
Moreover, as artificial intelligence continues to evolve, incorporating principles of probability learning could lead to more human-like decision-making capabilities in machines. This confluence of cognitive science and technology poses exciting possibilities, reshaping industries and redefining the very essence of decision-making.
"Probability learning isn't just about numbers; it shapes how we navigate through uncertainty every day."
To sum up, probability learning emerges as a critical component influencing decisions in various fields. The understanding of its underlying cognitive processes paves the way for advancements in theory and practice. This exploration serves not only to inform but also to inspire future research.
Prelims to Probability Learning
In a world saturated with information and unpredictability, understanding the nuances of probability learning becomes essential. This section sets the stage for a deeper exploration of probability learning, touching on some of its key facets. The relevance of this topic resonates widely across various fields, from psychology to data science, and it serves as a lynchpin in aiding individuals to interpret outcomes and make informed decisions. By grasping the concept of probability learning, we prepare ourselves to navigate uncertainties in a more educated, strategic manner.
Probability learning encompasses the processes by which individuals enhance their comprehension of likelihoods associated with different events. Whether it’s predicting weather, assessing risks in investments, or even making daily life choices, the dictates of probability shape our actions profoundly. By engaging with probability, we open up avenues for improved judgment and reasoning in various situations.
A few significant benefits emerge from appreciating the significance of probability learning:
- Enhanced Decision-Making: Understanding probability informs better predictions about outcomes, ultimately leading to more favorable choices.
- Informed Risk Assessment: By employing probability concepts, individuals can better gauge risks, understanding not just the chances of success but also the potential downsides.
- Foundation for Statistical Literacy: In an age inundated with data, a solid grasp of probability is foundational for interpreting statistical claims and studies, which are ubiquitous in research and media.
Yet, while the advantages of probability learning are considerable, one must also ponder on certain considerations. For instance, cognitive biases can heavily influence how probabilities are perceived, leading to miscalculations or poor judgments. The importance of critical thinking and skepticism cannot be understated, as the integration of probability in everyday thought processes is susceptible to distortions.
In this landscape, we delve deeper into various components, beginning with a solid understanding of what probability learning entails.
Understanding Probability Learning
Probability learning centers around the cognitive mechanisms people utilize to assimilate knowledge about the likelihood of various outcomes. It's essentially an ongoing process through which individuals refine their ability to predict uncertainties based on past experiences. For instance, consider someone who frequently plays the lottery. Over time, they may develop a sense of which numbers come up more often, leading to specific choices that maximize their perceived chances of winning.
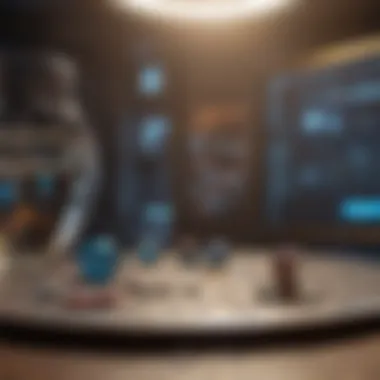
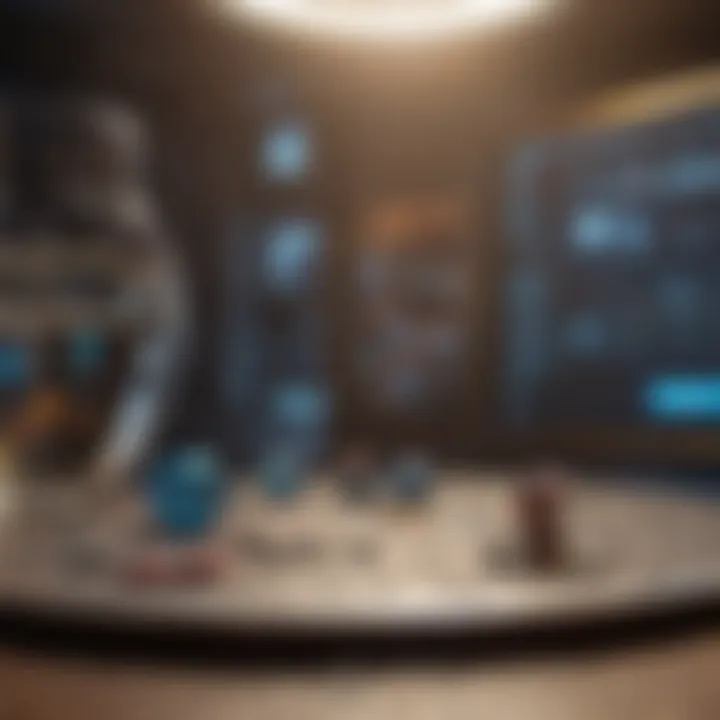
In simpler terms, people learn probabilities not just from direct experiences but also from observing patterns and feedback from the environment. This learning process can be both explicit, where one consciously evaluates and calculates probabilities, and implicit, where understanding develops through repeated exposure without formal instruction.
Historical Context
To appreciate the evolution of probability learning, we must glance back at its historical roots. The concept of probability can be traced back to ancient civilizations, where early forms of gambling and risk assessment hinted at an innate understanding of chance. However, it wasn't until the 17th century that probability began gaining traction as a formalized area of study.
Early mathematicians like Blaise Pascal and Pierre de Fermat laid the groundwork through correspondence focused on gambling, thus heralding the birth of probability theory. Here, probability began intertwining not just with mathematics but also with philosophy, stewing new insights into uncertainty and decision-making. Over the years, the development of probabilistic models, particularly in fields such as economics and psychology, has transformed how we perceive and interact with the concept of chance.
The historical progression has seen shifts from simplistic views on luck to sophisticated frameworks that underpin modern statistical practices. The increasingly complex world we now live in demands a higher level of competency in managing uncertainties, leading to the implementation of probability learning in diverse domains like genetics, finance, and artificial intelligence.
In wrapping up this section, understanding the journey of probability learning enhances our perspectives on how far we've come—and perhaps how much further we still need to go in mastering the art of evaluating probability in everyday life.
Theoretical Foundations
Understanding the theoretical foundations of probability learning is essential for grasping how individuals process the likelihood of various outcomes in uncertain situations. This section dives into the concepts that underlie probability learning, providing a framework that informs both academic inquiry and practical applications across several domains.
Classic Theories of Probability
Probability has been a significant focus in mathematical study since its inception. Classic theories of probability provide groundwork upon which modern approaches are built. These theories often stem from intuition or empirical observations, leading to established calculations of likelihood. The two main branches of classical probability are:
- A Priori Probability: This is derived from theoretical reasoning rather than empirical observation. For example, when tossing a fair coin, one can assert that the probability of landing either heads or tails is 50%. This assertion relies on the symmetry of the situation.
- A Posteriori Probability: In contrast, a posteriori probability is based on empirical data. Consider a weather forecast; if data indicates that similar conditions have led to rain 70% of the time, one might estimate a 70% chance of rain under current conditions.
Understanding these theories is crucial as they inform how data and outcomes are evaluated in various fields. They highlight the importance of not just calculations but also the reasoning behind those figures.
Bayesian Inference
Bayesian inference represents a significant shift from classical theories, allowing for updates in probability assessments with new evidence. Developed from Bayes' Theorem, it emphasizes the use of prior knowledge, or beliefs, when estimating probabilities.
In real-world applications, this can be particularly powerful. For example, a doctor diagnosing a patient may use known statistics about symptoms alongside individual patient data to update the probability of a particular illness. This iterative process is essential in fields such as medicine, finance, and machine learning.
Key Components of Bayesian Inference:
- Prior Probability: It reflects our beliefs before seeing new data.
- Likelihood: The chance of the observed data given a hypothesis.
- Posterior Probability: The updated belief after taking into consideration the new evidence.
This method provides a structured approach to uncertainty, making Bayesian inference a fundamental concept in probability learning.
Frequentist vs. Bayesian Approaches
The debate between Frequentist and Bayesian approaches illustrates the contrast in handling probability. Frequentists interpret probability in terms of long-run frequencies, focusing strictly on the data observed without incorporating prior beliefs. These methods often rely on p-values and confidence intervals, positioning them firmly in traditional statistical paradigms.
On the other hand, Bayesian approaches embrace subjectivity, allowing researchers to quantify the uncertainty of their knowledge. This flexibility can lead to more tailored analyses, especially in scenarios that are heavily dependent on unique data or variables.
Key Differences Between the Approaches:
- Interpretation of Probability: Frequentist sees probability as long-term frequency, while Bayesian views it through degrees of belief.
- Use of Prior Information: Bayesian methods include priors; Frequentist strictly relies on data gathered.
- Flexibility: Bayesian methods can adapt more readily to new information in dynamic environments, while Frequentist methods are more rigid and often rely on established models.
Understanding these differences is crucial for students, researchers, and professionals working with probabilities, as the choice between these approaches may influence their findings and the implications derived from their analyses.
"The theoretical foundations of probability learning not only inform how outcomes are interpreted, but also enhance decision-making in professional practices where certainty is often elusive."
Cognitive Mechanisms in Probability Learning
Understanding cognitive mechanisms is crucial for grasping how we learn probability. These mechanisms not only shape our approach to assessing chances but also influence decision-making processes in multifaceted environments. They cover a range of psychological functions, including memory, experiences, and reasoning strategies, all of which play a pivotal role in how individuals make sense of uncertainty.
Role of Memory and Experience
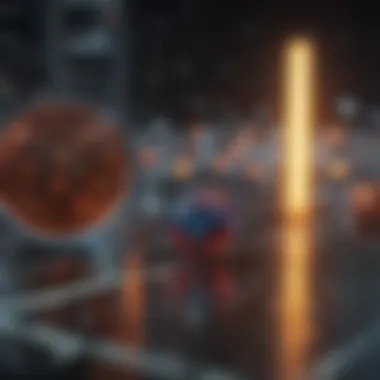
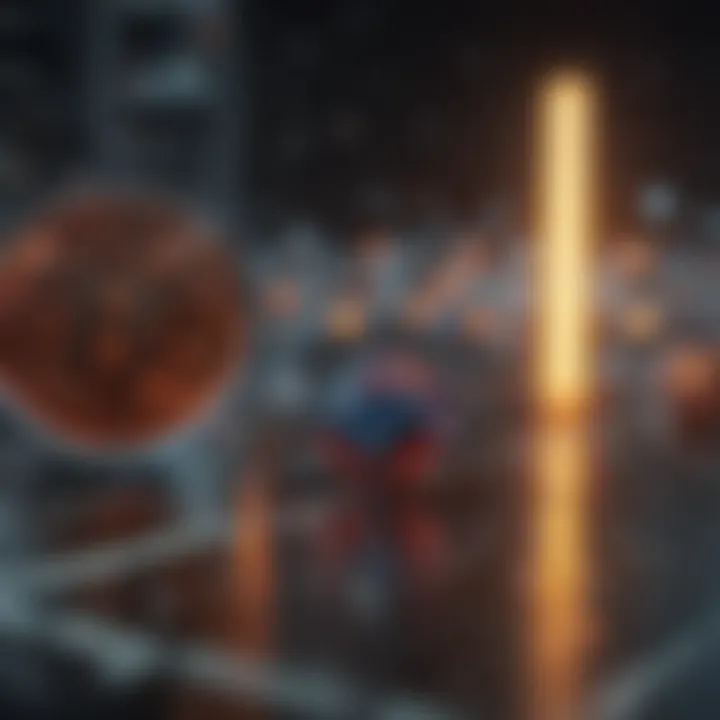
Memory serves as a fundamental conveyor of learned probabilities, acting as the repository of past events and outcomes. When we engage in probability learning, we pull from this well of experiences, employing both explicit and implicit memory. For instance, consider how someone might remember a game's previous outcomes to gauge their future odds of winning. This interplay means that our recollections can shape how we understand probabilities moving forward.
Moreover, the bias of recency, where the most recent experiences weigh heavier in our assessments, can drastically skew our judgment. If one were to witness a series of unfortunate events, like losing several bets in a row, they may unjustly estimate the chance of winning in subsequent trials as lower—an effect known as the gambler's fallacy.
In contrast, another individual, drawing from a longer memory span or more varied experiences, might maintain a more tempered view of odds. Here, experience acts as a double-edged sword—it enriches our understanding yet can cloud our judgment, especially under emotional distress or cognitive fatigue.
Heuristics in Decision Making
Heuristics, those mental shortcuts derived from experience, significantly impact how we estimate probabilities. They allow for quicker decision-making but are often fraught with cognitive biases. A prime example is the availability heuristic, where people evaluate the likelihood of events based on how easily examples come to mind. If an individual frequently sees news reports about plane crashes, they might overestimate the risk of flying, despite statistical data indicating it's one of the safest modes of transport.
Another common heuristic is the representativeness heuristic, which involves making judgments based on how closely something matches a prototype or stereotype. For example, if a person fits a certain mold—let’s say, a quiet library-goer—they might incorrectly assess their behavior based on that stereotype, not considering the full context. This can distort our understanding of probabilities, leading to flawed decision-making.
> Understanding these cognitive mechanisms is vital. They don’t just help us comprehend the probabilities; they also steer our actions and responses. As we navigate through situations laden with uncertainty, recognizing these influences can significantly improve our decision-making processes and overall judgments.
Empirical Studies on Probability Learning
Examining empirical studies on probability learning provides a window into how individuals and groups internalize the likelihood of various outcomes. This rigorous research illuminates not just theoretical concepts, but also practical applications that vary widely across fields—from education to economics. Engaging with these studies encourages a deeper appreciation of the dynamic interplay between theory and real-world context. As we delve into actual findings, we begin to grasp how probability learning influences decisions, shapes perceptions, and informs behaviors in uncertain settings.
Key Findings from Research
Empirical investigations reveal pivotal insights that challenge preconceptions and expand understanding of probability learning. Here are some of the main takeaways from such research:
- Adaptive Learning: Many studies indicate that learning probabilities tends to shift based on environmental feedback. In a classroom setting, this means students adjust their understanding of odds with practice and exposure, moving from intuitive guesses to calculated estimations.
- Influence of Cognitive Biases: Research underlines how cognitive biases, such as the availability heuristic, significantly skew probability assessments. For instance, individuals might overestimate the likelihood of events simply because those instances are more memorable or recently encountered, affecting judgement.
- Statistical Competence Across Cultures: A fascinating body of research suggests variations in probability understanding exist across cultures. Studies have shown that individuals accustomed to specific statistical traditions display different approaches in estimating probabilities, thereby highlighting that context matters.
"The heart of probability learning resides in our ability to adapt and refine our understanding through feedback from the world around us—often more so than what any static textbook could teach."
Moreover, several experimental frameworks have been utilized to examine how people derive probabilities from limited information. For example, research involving simple coin toss games illustrates how participants utilize prior outcomes to predict future results, illustrating adaptive behavior in probability learning.
Case Studies in Different Domains
When exploring practical implications, case studies from diverse areas provide compelling narratives about how probability learning plays out in everyday situations. Here are some illustrative examples:
- Education: In educational settings, studies show how incorporating simulations that require students to estimate probabilities leads to a marked improvement in statistical reasoning. For instance, interactive games that involve predicting weather outcomes can sharpen critical thinking skills.
- Healthcare: Research within the healthcare domain sheds light on probability learning’s role in decision-making. Physicians, when confronted with complex case scenarios, often mingle risk assessments with probability, dictating treatment paths based on statistical evidence derived from past patient data.
- Finance: In financial markets, traders make decisions influenced by probability learning. Case studies focus on how traders analyze historical trends and chart patterns, training themselves to discern underlying probabilities concerning stock movements.
- Digital Platforms: Social media platforms, like Facebook, utilize probability learning in their algorithms, predicting user behavior while tailoring content. Research highlights user engagement metrics, emphasizing how data on user interactions help refine future predictions.
Combining findings from various studies illustrates the far-reaching impact of understanding probabilities in real-world contexts, laying bare the nuances of how this knowledge is employed across professional landscapes. Readers can begin to see the full tapestry woven from these empirical threads, showcasing the multifaceted nature of probability learning.
Applications of Probability Learning
Understanding how probability learning functions can profoundly impact various fields. It works like a compass, guiding decisions in contexts where uncertainty reigns. Learning to evaluate probabilities is not just an abstract theory; it shapes practical applications that permeate daily life and professional practices.
Probability Learning in Education
Education is one vital area where applying probability learning can enhance student understanding. When teachers incorporate probability concepts into their curriculum, it enables learners to grasp not just math, but critical thinking skills. For instance, using real-life scenarios like weather forecasts can make abstract principles tangible.
- Improved Decision-Making: Students who learn probability can analyze potential outcomes of their choices, fostering better decision-making.
- Engagement Through Context: Presenting probability through relevant examples—like sports statistics or gambling odds—can lead to more engaged students.
- Critical Thinking Development: It encourages students to question and evaluate the likelihood of events, a skill that extends beyond math classes.
"Teaching probability not only cultivates mathematical skills but nurtures a mindset geared towards critical evaluation."
Impact on Machine Learning Algorithms
The influence of probability learning is magnified in the realm of machine learning. Algorithms often rely on probabilistic models to make predictions or classify data. These models use historical data patterns to assess the likelihood of future events, enabling various applications, from recommendation systems on streaming platforms to predictive text in messaging apps.
- Enhancement of Predictions: Machine learning models, much like finely tuned sports cars, require accurate probability assessments to run smoothly. The more precise the estimations, the better the performance of these systems.
- Risk Management: Companies employ probability methodologies to anticipate risks, allowing for a proactive approach rather than reactive measures.
- Algorithm Adaptability: As new data comes in, algorithms adjust their probabilities, learning continuously and thus improving their outputs over time.
Probability in Economic Decision Making
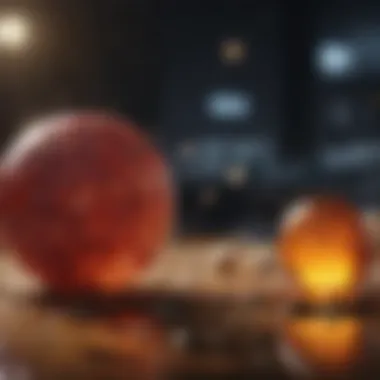
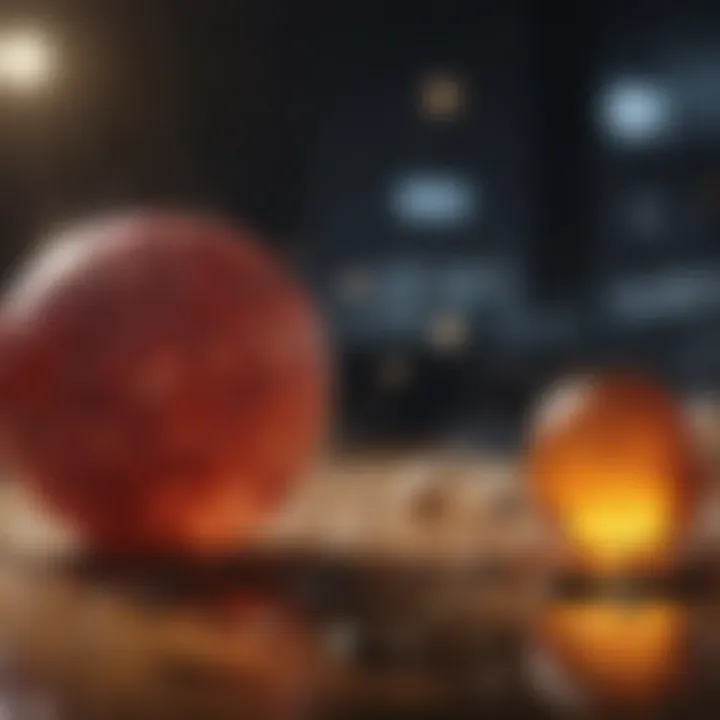
In the world of economics, probability learning figures substantially in shaping decisions made by individuals and institutions. Whether considering investments or understanding market trends, a firm grasp of probabilities can be the difference between profit and loss.
- Investment Strategies: Investors often look at probabilities to judge the risk versus reward of a potential investment. Knowledge of risk helps in crafting portfolios that align with one’s financial goals.
- Market Predictions: Analysts rely on probability models to forecast economic trends, driving strategic planning.
- Consumer Behavior Insights: Businesses harness probability to understand consumer choices, allowing them to meet market demands more effectively.
In summary, the applications of probability learning are extensive and diverse. From shaping educational practices to enhancing machine learning algorithms and guiding economic decisions, the relevance cannot be overstated. Recognizing these applications provides a clearer view of how integral probability learning is to navigating our complex world.
Challenges and Limitations
The realm of probability learning is not without its hiccups. While the concept holds significance across various disciplines, the challenges and limitations present obstacles that can skew our understanding and application of probabilities. Recognizing these hurdles is crucial for anyone involved in research, education, or practical applications of probability.
Biases in Probability Estimation
When we think about estimating probabilities, it becomes clear that human perception plays a critical role. Cognitive biases can deeply impact how individuals assess likelihoods. People might lean on personal experiences or societal norms, which tend to cloud judgment. The availability bias, wherein we estimate probabilities based on recent memories, is a prime example. Say you hear about a string of robberies in your neighborhood; suddenly, you might irrationally believe that crime is on the rise, even if statistically, it's at a historical low.
Another common bias is the confirmation bias. This occurs when individuals seek out or interpret information in a way that confirms their preexisting beliefs. In probabilistic contexts, this can lead to a warped estimation of outcomes, further compounding errors in reasoning about risks and chances.
To combat these biases, awareness is paramount. Tools such as structured decision-making techniques or simulations can aid in mitigating human error.
"Awareness of our cognitive pitfalls is the first step in addressing them."
Complexity of Real-World Applications
Taking a step beyond mere theories, real-world applications of probability learning often unveil another layer of complexity. Probability isn't static; it's influenced by a multitude of variables ranging from environmental factors to individual behavioral patterns. For instance, in fields like economics and healthcare, the intertwining of various elements can turn even the most straightforward probability assessments into intricate puzzles.
One must consider the dynamic nature of probabilities in systems like stock markets, where fluctuations are not solely dictated by numbers but heavily impacted by human emotion and news cycles. Similarly, in healthcare, patient behavior and unpredictable epidemic trends can distort the anticipated probabilities of certain conditions.
Additionally, it's worth noting that many algorithms designed to predict outcomes are often built on idealized models. When these models are applied to chaotic real-world situations, outcomes can diverge swiftly from predictions. Incorporating feedback loops and adapting to new information becomes essential, yet that introduces its own set of challenges.
Future Directions
The exploration of probability learning is increasingly crucial in our fast-paced, data-driven world. Understanding how individuals develop insights into probabilities not only enhances learning strategies but also supports decision-making across various disciplines. As we look toward future directions in this field, there are specific elements that rise to the surface, waving their flags for attention.
Advancements in Research Methodologies
With technology evolving like a whirlwind, the methodologies used in studying probability learning will also need to adapt. Advanced statistical tools and computational models are becoming more prevalent. For instance, machine learning techniques can crunch numbers faster and uncover patterns hidden in data that traditional methods might overlook.
Doing this allows researchers to simulate different scenarios, thereby providing deeper insights into how probabilities are internalized.
Consider the rise of neuroimaging techniques as well; they can offer a peek into the brain's workings during probability assessments. This approach can lead to a richer understanding of cognitive processes underpinning learning and decision-making.
✅ Embracing these advancements enhances the accuracy of research conclusions and paves the way for the refinement of theoretical frameworks.
Interdisciplinary Approaches
The task of dissecting probability learning does not belong solely to one discipline. Instead, it thrives within an interdisciplinary landscape. Incorporating insights from psychology, economics, and cognitive science allows for a more holistic understanding.
For example, principles from behavioral economics can uncover how emotional biases impact probability judgments. Insights from psychology can illuminate memory's pivotal role in how probabilities are learned and recalled.
As we move forward, collaborations across these fields should be emphasized. Interdisciplinary teams can develop multifaceted approaches to research, leveraging diverse expertise.
- Integration of Teaching Practices: Educators can adapt findings from cognitive psychology to improve teaching in statistics and probability.
- Economic Modelling: Economists can apply what is learned about probability to refine models affecting market predictions and risk assessments.
- AI and Machine Learning: The tech realm, particularly in AI, can benefit from probability learning to enhance algorithms that predict user behavior or market trends.
Such collaborative efforts can yield richer insights than a singular focus. They will also create more effective strategies for applying these insights, ultimately enhancing decision-making in real-world contexts.
"Interdisciplinary collaboration is not just a nice-to-have; it’s an essential engine driving innovation and depth in research across fields."
As we venture into the future, integrating methodologies and fostering interdisciplinary relationships will be key to expanding our understanding of probability learning and its implications.
Closure
The concluding section of this article holds significant weight as it synthesizes the myriad concepts explored throughout the discourse on probability learning. It serves not just as a wrap-up but as a pivotal moment to reflect on the breadth of insights gained about the ways individuals estimate and respond to uncertainty in decision-making.
Summarizing Key Insights
At the heart of our exploration, we unearthed several critical takeaways:
- Understanding Probability Learning: Individuals acquire skills to assess the likelihood of events through both experience and instruction. This is not merely academic; it has practical implications in everyday life, from simple decisions about weather predictions to complex financial forecasts.
- Cognitive Mechanisms: Memory's role, along with cognitive biases, shapes how we interpret probabilities. Our understanding of heuristics informs us that often, reliance on mental shortcuts can lead to systematic errors, but they also enhance speed in decision-making processes.
- Empirical Findings: Various studies corroborate that probability learning is influenced by context and can vary across different domains, such as education and machine learning applications. This reinforces that while there are common threads across scenarios, each field brings unique challenges and insights.
- Applications and Challenges: The benefits of applying probability in contexts like education and economics are evident, yet obstacles, such as biases and the complexity of real-world data, remain. Addressing these challenges is fundamental to enhancing the effectiveness of probability learning across fields.
In sum, understanding the nuances of probability learning enlightens us about human behavior and decision-making, emphasizing that how we discern risks and opportunities is critical in nearly every aspect of life.
Implications for Future Research
As we gaze into the horizon of probability learning, several intriguing avenues beckon:
- Enhanced Methodologies: Continued development of research methods will better capture how individuals navigate uncertainty. Advanced techniques in neuroimaging could provide deeper insights into the cognitive processes underpinning probability learning.
- Cross-Disciplinary Integration: Bridging insights from psychology, economics, and artificial intelligence may yield richer frameworks for understanding probability learning. This integration might reveal new patterns and heuristics that apply across disciplines, offering a more robust model of decision-making.
- Intervention Strategies: Future studies could focus on interventions designed to mitigate biases identified in probability estimation. This may empower individuals to make more informed decisions while cultivating awareness of their own cognitive limitations.
- Impact of Technology: As technological advances reshape data analysis and machine learning, it would be prudent to examine their effects on our understanding of probability. Investigating how AI systems influence human decision-making can unveil underlying shifts in what it means to comprehend and interpret probabilities.