Understanding the Bias Meter: Evaluating Information
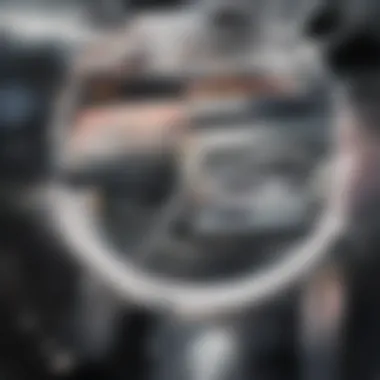
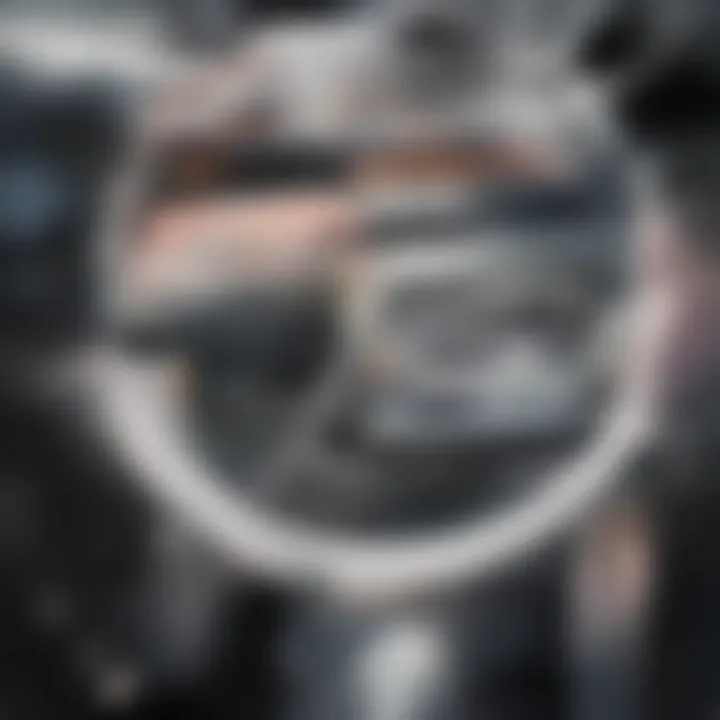
Intro
In a world overflowing with information, discerning the reliability of data has become paramount. The rise of misinformation, especially in journalism and online content, necessitates robust tools for evaluation. The Bias Meter stands out as a significant player in this arena. This article aims to delve deeper into what the Bias Meter is, how it operates, and its broader implications for accuracy in information dissemination.
Research Background
Overview of the Scientific Problem Addressed
As societies become more reliant on digital platforms for news and information, the risk of biased or misleading content increases. This presents a scientific challenge in understanding how bias affects perception and knowledge. The Bias Meter is designed to address this issue by evaluating the neutrality of information, contributing to a better understanding of media credibility.
Historical Context and Previous Studies
The development of bias detection tools has been a response to the growing prevalence of content that lacks objectivity. Early studies focused on manual bias detection through critical analysis but were often time-consuming and inconsistent. Over time, automated tools emerged, using algorithms to gauge bias levels in texts. Research from various fields has shown that biases can subtly affect even well-intentioned reporting—leading to skewed narratives. The Bias Meter builds on these foundational studies, employing advanced technologies and methodologies.
Findings and Discussion
Key Results of the Research
The Bias Meter utilizes a blend of linguistic analysis and machine learning to determine the bias level of written content. Initial findings indicate that it can successfully identify biased language and evaluate the objectivity of sources. This capability has proven helpful in multiple scenarios, from academic research to journalism.
Interpretation of the Findings
Understanding these results sheds light on the practical applications of the Bias Meter in various fields. Its effectiveness lies in not just identifying bias but also in encouraging a culture of careful information engagement. As users become more aware of how biases work, they can make informed decisions on the credibility of the content they consume.
"The Bias Meter represents not just a tool, but a shift towards responsible information consumption practices."
Implications for Information Accuracy
The implications of using the Bias Meter extend beyond mere detection. By encouraging users to scrutinize content, it promotes healthier discourse in public spheres. This goes beyond journalism; educators and students can benefit from such a tool to ensure the integrity of academic materials.
Finale
Prologue to Bias and Its Relevance
In today’s fast-paced information landscape, it is essential to recognize the influence and pervasiveness of bias. Understanding bias is not just an academic exercise but a practical necessity for anyone engaging with information. This section aims to illuminate the concept of bias, its significance, and how it shapes our interpretation and acceptance of information across various fields.
Defining Bias in Information
Bias can be understood as a tendency to lean in a certain direction, affecting judgment or perception. It is not necessarily malintent; rather, it often arises from contextual factors such as cultural backgrounds, personal beliefs, and societal influences. Information can be biased in many forms: selection bias, confirmation bias, and framing bias. Each type alters the message conveyed and can distort our understanding of facts.
Recognizing bias helps in critically evaluating content. For students, researchers, and professionals, this discernment enables them to filter out misinformation and seek truth in data. Consider examining sources carefully, questioning the intent behind the information provided, and appreciating multiple viewpoints. This approach leads to more informed conclusions and decisions.
The Role of Bias in Scientific Discourse
Bias plays a significant role in scientific discourse. Scientists are not impervious to personal biases that can affect research outcomes and interpretations. Peer pressure, funding sources, and prevailing theories may alter the objectivity of scientific studies. Certain results may be given preferential treatment based on their alignment with existing paradigms.
Understanding these biases is crucial for researchers. It shapes the integrity of published work and impacts how knowledge is disseminated. Accountability in scientific research compels scholars to adopt rigorous methodologies. They must consider all variables that might influence results, ensuring a balanced representation of findings. Recognizing bias in science encourages a culture of skepticism and inquiry—fundamental principles for any genuine scholarly pursuit.
Historical Perspectives on Bias
Bias is not a new phenomenon. Historically, the concept has evolved, with various examples illuminating its impact across different periods. For instance, during the Enlightenment, biased interpretations of scientific discoveries often clashed with traditional beliefs. The heliocentric model proposed by Copernicus instigated conflict as it challenged the long-held geocentric views. Such historical precedents highlight the push and pull of bias against objective truth.
Further, the media's role in shaping public perception has always been intertwined with bias. Events like the U.S. Civil Rights Movement reveal how media representation can shape societal attitudes. Biased reporting often affected the public's understanding of complex issues, influencing policy decisions and social movements.
The Concept of the Bias Meter
In today's information-rich environment, the concept of the Bias Meter is pivotal. It serves as a beacon guiding individuals through the complexities of biased information. This section will detail what a Bias Meter is, its essential components, and the theoretical framework that supports it. By grasping these elements, readers will enhance their ability to apply this tool effectively in their analysis of information.
What Is a Bias Meter?
A Bias Meter is a tool designed to analyze the level of bias in a piece of information. This can be in the context of news articles, research papers, or other forms of communication. The tool quantifies bias to present a clear picture of how objective or subjective the information may be. The main purpose of the Bias Meter is to aid users in understanding potential slants in content, allowing for more informed consumption of information.
Some features of a Bias Meter may include:
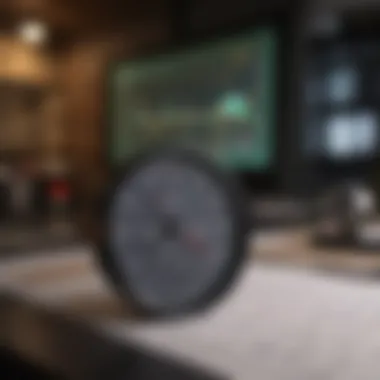
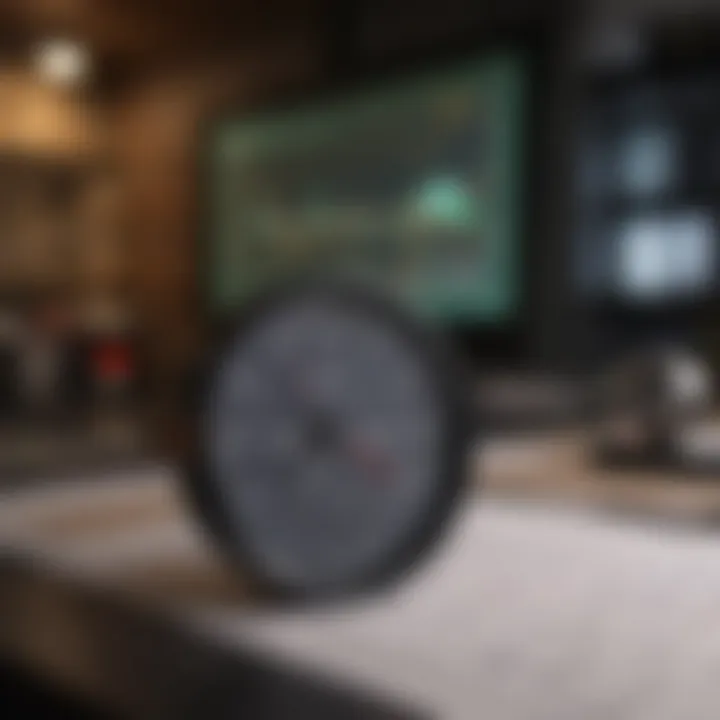
- A scoring system to evaluate the degree of bias.
- Metadata analysis that includes the author's background and intent.
- Contextual consideration to assess external influences on the content.
By providing these insights, users can make better decisions about the reliability of the information they consume.
Components of a Bias Meter
The composition of a Bias Meter typically involves several key elements that work together to assess bias effectively. These components include:
- Data Sources: Information is collected from diverse media and academic sources, ensuring a broad perspective on bias.
- Analysis Algorithms: Sophisticated algorithms are employed to evaluate language, tone, and structure against known bias indicators.
- User Interface: An intuitive interface allows users to easily visualize bias metrics and understand the results at a glance.
- Feedback Mechanisms: Users can provide input on the reliability of bias ratings, enhancing the learning algorithms over time.
These components interact to produce reliable assessments of bias. Understanding these elements helps users navigate the tools more skillfully.
Theoretical Framework Underpinning the Bias Meter
The efficacy of a Bias Meter is grounded in several theoretical frameworks from various disciplines. One significant area is psychology, particularly the study of cognitive biases. The human tendency to favor certain information over others significantly influences how bias manifests in writing and communication. Furthermore, frameworks from critical theory examine how power structures shape narratives, leading to inherent biases.
Aspects of the theoretical framework include:
- Constructivism: This asserts that individuals construct knowledge based on their experiences and biases.
- Linguistic Relativity: Language shapes thought, which can affect perception of bias.
- Social Constructivism: Emphasizes how societal context influences the understanding of bias in communication.
By applying these theories, the Bias Meter becomes not just a tool but a sophisticated instrument that reflects the complexities of human understanding and communication.
"Tools like the Bias Meter are vital in a world filled with information. They help us discern fact from opinion."
Understanding the Bias Meter's components and underpinning theories is essential for anyone seeking to evaluate information critically. This knowledge empowers individuals to challenge biases and appreciate the intricacies in communication.
Methodologies Behind Bias Detection
The methodologies behind bias detection are integral to the development and functionality of the Bias Meter. These approaches shape how bias is identified and evaluated, influencing user trust in the information presented. By understanding the various methodologies employed, one can appreciate the complex layers that contribute to an accurate assessment of bias in different contexts. This section outlines key methodologies focusing on algorithmic, human, and combined approaches, showcasing their respective strengths and limitations.
Algorithmic Approaches to Measuring Bias
Algorithmic methods rely on computational techniques to analyze text and determine bias. This involves the use of natural language processing (NLP) algorithms designed to examine word choice, context, and sentiment. These algorithms evaluate data patterns, which helps in recognizing biased language or the slant of information.
Key aspects of algorithmic bias detection include:
- Scalability: Algorithms can process vast amounts of text quickly, making them suitable for analyzing large datasets.
- Objectivity: Automated systems can minimize human bias in evaluation; however, they are not entirely immune to bias ingrained in their programming.
- Speed: Algorithmic approaches can produce results immediately, beneficial in situations requiring rapid assessments.
Despite these advantages, algorithmic methods often struggle with context. They may misinterpret sarcasm or fail to recognize subtle biases. Thus, while they provide a foundation for bias detection, they should not be solely relied upon.
Human-Centric Bias Assessment Techniques
Human-centric bias assessment recognizes that humans play a crucial role in evaluating bias. These techniques often involve qualitative analysis, where individuals interpret nuances that algorithms might miss. This method tends to be more subjective, relying on experts’ insights and cultural context.
Considerations in human-centric assessments include:
- Contextual Awareness: Humans can factor in context, such as cultural references or societal norms.
- Nuanced Judgments: Trained professionals can detect subtle biases that automated systems may overlook.
- Ethical Considerations: Human assessors can apply ethical standards to their evaluations, considering the potential implications of their findings.
However, human biases can also influence assessments. Personal beliefs may skew evaluation, leading to inconsistencies. This reality highlights the necessity of combining both algorithmic tools and human judgment for comprehensive bias detection.
Combining AI and Human Insights in Bias Detection
The integration of AI and human insights is a promising approach in bias detection. By leveraging the strengths of both methodologies, this hybrid technique aims for accuracy and depth in the evaluation process.
Benefits of this combination include:
- Enhanced Accuracy: Algorithms can filter and rank information, while human reviewers can provide critical insights based on context, improving overall accuracy.
- Iterative Learning: This approach allows for continuous learning. Human feedback can refine algorithms, enabling systems to learn from mistakes over time.
- Balanced Evaluation: Combining objectivity with nuanced interpretation allows for a more holistic view of bias within content.
Despite the advantages, challenges remain in ensuring effective communication between human and AI systems. Training data must be immaculately curated to further reduce bias and improve efficiency.
Overall, understanding these methodologies is essential for effectively utilizing the Bias Meter. Each method has unique strengths and weaknesses, making it critical to approach bias detection with a multi-faceted perspective. By doing so, the journey toward genuine objectivity in evaluating information can be navigated more skillfully.
Applications of the Bias Meter
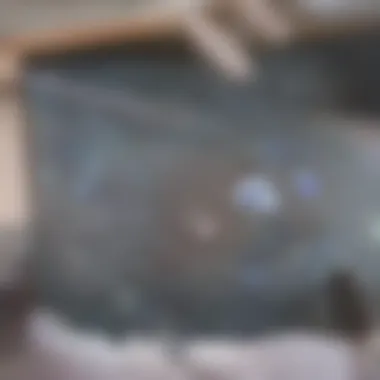
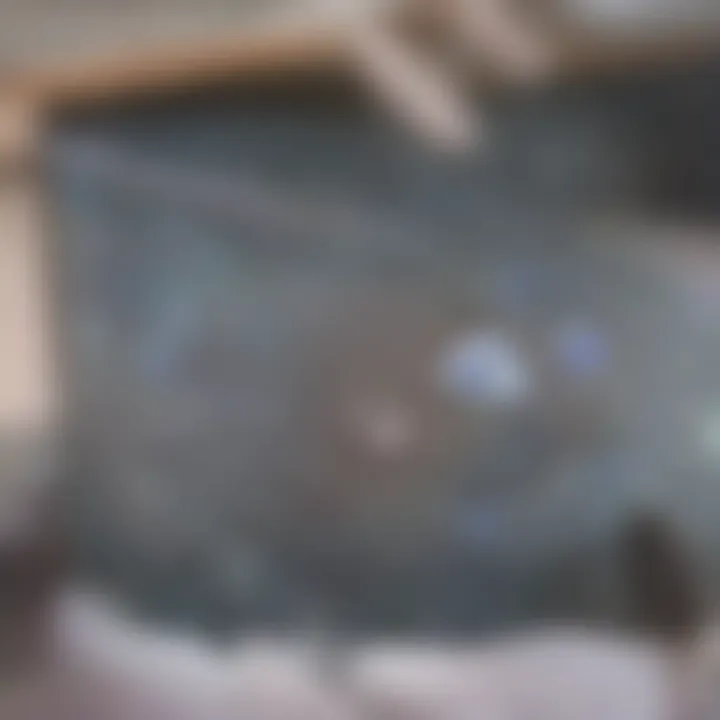
The applications of the Bias Meter are essential for understanding its role in different domains. This tool is not only about identifying bias but also about enhancing the integrity of information. By quantifying bias, it allows users to navigate complex narratives in journalism, academia, and education more effectively. This section explains these applications in detail, covering aspects such as their benefits and considerations.
Use in Journalism and Media
In journalism, the Bias Meter serves a critical function. News organizations utilize it to ensure fairness and objectivity in reporting. Many journalists strive to provide balanced accounts, yet bias can seep into coverage unrecognized. By employing a Bias Meter, they can assess their narratives holistically. This leads to several benefits:
- Increased transparency: Readers can trust that the news is presented with minimal bias.
- Enhanced credibility: News outlets that use the Bias Meter can improve their reputation by demonstrating their commitment to impartiality.
- Reader engagement: An awareness of bias fosters a discerning audience that questions the information presented.
Nevertheless, challenges do exist. The nuanced nature of events and personal opinions can complicate bias detection. Implementing a Bias Meter without understanding context can lead to misleading interpretations of neutrality.
Implications for Academic Research
The applications of the Bias Meter extend into the realm of academic research. Researchers face the continuous challenge of ensuring their work is free from bias. This can significantly impact the validity of findings and the perception of research integrity. Here, the Bias Meter can help:
- Evaluating sources: Researchers can determine the reliability of materials more effectively.
- Guiding the research process: Understanding potential biases can inform the methodology used, thus enhancing the robustness of the study.
- Promoting ethical standards: Employing a Bias Meter helps maintain high ethical standards within the academic community.
Academic institutions can benefit from adopting this tool. It encourages a culture of critical evaluation and responsibility among researchers. However, it is important to recognize that no tool is infallible, and bias detection should complement traditional methodologies rather than replace them.
Educational Uses of the Bias Meter
Education is another area where the Bias Meter finds significant application. It can foster critical thinking skills among students. The inclusion of bias measurement in curricula can lead to several outcomes:
- Improved analytical skills: Students learn to assess information critically, a vital skill in today's information-rich environment.
- Informed citizenry: Understanding bias enhances students' ability to engage with media and news responsibly.
- Active participation in discourse: Students become more empowered to contribute to discussions on societal issues.
Despite these advantages, there are challenges to consider. Some educators may find it difficult to integrate tools like the Bias Meter into existing curriculums. Furthermore, students may require guidance to understand the implications of bias fully.
The Bias Meter acts as a lens, helping users in journalism, academia, and education scrutinize the reliability of information effectively.
The applications of the Bias Meter are wide-ranging and impactful. In journalism, it ensures fair reporting; in academia, it maintains research integrity; and in education, it nurtures critical thinkers. As information continues to evolve, the relevance of the Bias Meter becomes increasingly vital in promoting a more discerning society.
Navigating Ethical Concerns
The incorporation of the Bias Meter into information evaluation raises several ethical concerns. These concerns are critical, as they affect the tool's reliability and acceptance. Ethical considerations guide its implementation in practice, ensuring that the choices made during the bias measurement process do not inadvertently harm users or skew the information presented. In this section, we will explore key ethical aspects surrounding bias measurement, potential misuse, and the balance between objectivity and subjectivity.
The Ethics of Bias Measurement
The ethics of bias measurement come down to how tools interpret data and the impact of those interpretations. Instruments like the Bias Meter must be transparent in their methodology. Users must understand how the bias is detected and measured. This transparency prevents the tool from being simply a black box, where hidden algorithms could manipulate or misrepresent information.
Moreover, the developers of bias measurement tools have the responsibility to ensure accuracy and fairness. When biases are identified, they should not just label information as biased without context. It is essential to explain the extent and type of bias, allowing users to make informed judgments. Ethical practices also involve updating the measurement tools according to evolving norms and biases in society.
"Ethics in bias measurement is not just preferred; it is necessary for the integrity and credibility of the process."
Potential Misuse of Bias Metrics
While the Bias Meter serves as a useful tool, it can be misused in various ways. One concern is the potential for individuals or organizations to selectively highlight bias metrics to drive a specific agenda. For instance, a biased portrayal might arise when only certain results are showcased, ignoring others that could paint a more nuanced picture. This selective use can exacerbate polarization and misinformation.
Another aspect of misuse is the reliance on numerical metrics without adequate context. Users may take bias scores at face value, leading to oversimplified conclusions about complex issues. Furthermore, if the public does not critically engage with the data provided, it could encourage complacency in verifying actual information sources.
To combat potential misuse, frameworks for responsible use must be established along with guidelines for interpreting bias metrics correctly. Users must become educated consumers of bias data rather than mere recipients.
Balancing Objectivity with Subjectivity
In the realm of bias measurement, an important challenge is the balance between objectivity and subjectivity. Although bias detection aims to apply objective criteria, interpretations may still inherit subjective elements. Varying perspectives can lead to different conclusions about what constitutes bias in any piece of information or data set.
It is crucial to strike a balance here. Developers should create bias meters grounded in robust frameworks while remaining open to different angles of perception. Rapport within the community of users is vital for achieving a nuanced understanding. This can involve engaging consultations with stakeholders, researchers, and ethicists to gather insights on the most credible metrics and perspectives available.
In summary, navigating ethical concerns surrounding the use of a Bias Meter is fundamental. Understanding the ethical dimensions, recognizing potential misuse, and finding harmony between objective and subjective interpretations will instill greater trust in the Bias Meter. Through this careful consideration, we can foster better practices in information consumption.
Challenges and Limitations of the Bias Meter
The topic of challenges and limitations concerning the Bias Meter warrants serious consideration. Even as this tool seeks to enhance our understanding of bias, it is not without its shortcomings. Recognizing these limitations is essential for educators, researchers, and professionals who are keen on effectively utilizing the Bias Meter in their analyses. The limitations generally fall into three categories: technical, cultural, and adaptability issues.
Technical Limitations of Current Bias Meters
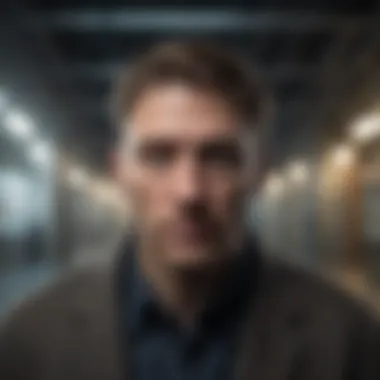
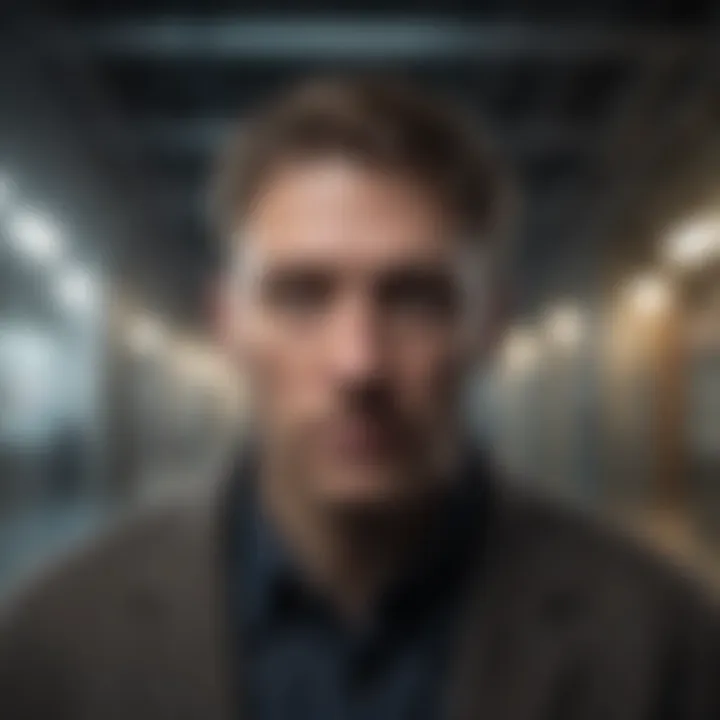
Current versions of bias detection tools face several technical challenges. One significant limitation lies in the algorithms themselves. Often, algorithms rely on predetermined datasets which may not capture the nuances of every context. The reliance on these datasets can result in misinterpretations. Some tools may mislabel content, which could mislead users seeking objective analysis. Moreover, the inability to update these systems in real-time hinders their effectiveness. As language and societal norms evolve, static models can quickly become outdated.
At the same time, there are also limitations linked to the data that these tools analyze. The quality of input data directly influences the output. If a bias meter receives biased information or a limited range of sources, its readings will reflect those biases, undermining the credibility of the results.
Cultural and Contextual Biases in Algorithm Design
Cultural and contextual biases present another layer of complexity when addressing the Bias Meter's effectiveness. The algorithms often reflect the values and perspectives of their creators. If a bias meter is developed in one cultural context, it may underestimate or overlook biases prevalent in another context.
For instance, an algorithm trained predominantly on Western media sources may not effectively analyze content from non-Western cultures. This gap can create disparities in understanding bias across different communities. Users need to consider the underlying biases brought by the design and development phase. These issues highlight the importance of diverse teams in developing bias detection tools, ensuring multiple perspectives are represented.
The Challenge of Continuous Adaptability
Adapting bias meters to keep pace with changing societal dynamics is a significant challenge. The discourse surrounding bias is ever-evolving, reflecting shifts in cultural attitudes, political sentiments, and technological advancements. As individuals become more aware of biases, new forms of bias may emerge, making it necessary for bias meters to continuously evolve.
A static tool lacks the capability to respond to these new forms of bias effectively. Thus, developers must prioritize an iterative design approach, updating algorithms regularly and enhancing their data training sets. Collaboration among researchers, software developers, and users is crucial for optimizing these tools to maintain relevance and accuracy in an ever-changing landscape.
Understanding these challenges helps stakeholders use the Bias Meter more effectively, ensuring it serves as a reliable resource in evaluating information.
Future Directions in Bias Measurement
The topic of future directions in bias measurement holds significant importance in the context of how we assess the reliability of information today. As biases permeate many aspects of communication, the ability to identify and measure them accurately is essential. The upcoming evolution in bias measurement can have profound implications not only for journalism and scholarship but also for how individuals interpret information in their daily lives.
Emerging Technologies and Their Impact
Emerging technologies are rapidly transforming the landscape of bias detection. Artificial intelligence and machine learning algorithms are at the forefront, enabling deeper analysis of textual and audiovisual content. These technologies facilitate the analysis of large datasets, allowing for the detection of subtle biases that human judgment might overlook.
Consider the role of natural language processing. This technology analyzes word choice, tone, and context in real-time, uncovering biases in news articles and social media posts. As advancements continue, the goal is to create frameworks that adapt and learn from feedback, improving their accuracy over time. This adaptability is essential in an environment where the nature of information and public discourse is constantly shifting. The potential benefits of using emerging technologies include:
- Enhanced detection of bias patterns in various types of media.
- Improved accuracy in identifying misinformation and disinformation.
- Increased accessibility of bias assessment tools for the public.
The Evolution of Public Perception of Bias
Public perception of bias is evolving alongside the tools used to measure it. Previously, many individuals might have considered bias solely as a flaw in reporting. Now, with tools like the Bias Meter, awareness of the complexity of bias has increased. People begin to understand that bias is not always intentional, but can manifest through systemic factors or cultural influences.
Educating the public about these nuances is vital. As individuals become more informed, their skepticism towards sources of information grows. This skepticism plays a crucial role in ensuring accountability among content creators and institutions. Furthermore, social media's impact on bias perception cannot be ignored. Users now engage in discussions surrounding media literacy, helping to shape a more discerning audience.
Creating Standards for Bias Measurement
Establishing standardized criteria for bias measurement is a pressing need. Currently, various tools assess bias differently, which can lead to confusion among users. As more organizations develop their methodologies, a unified set of standards would enhance transparency and reliability.
Such standards could include:
- Clearly defined metrics for assessing bias.
- Guidelines for evaluating the effectiveness of bias detection tools.
- Protocols for regular updates based on new research and societal changes.
By establishing these standards, the aim is to foster trust in bias measurement tools. Users should feel confident that these tools provide accurate assessments. Not only does this help in academic and professional settings, but it also empowers individuals to make informed choices about the information they consume.
Closure
The conclusion of this article serves as a vital synthesis of the numerous threads woven throughout the discussion on the Bias Meter. This section is not merely a recap; instead, it distills the essence of what has been explored, emphasizing the relevance and significance of the Bias Meter in our information-saturated society.
In reviewing the content, several key elements stand out. First, the concept of the Bias Meter is crucial in evaluating the reliability and credibility of information sources. By utilizing a structured approach to identify bias, it empowers users to make informed decisions. This tool is essential in an age where misinformation can spread rapidly across various platforms.
Benefits also arise from understanding how the Bias Meter functions. Users can develop a critical mindset, enhancing their ability to assess content beyond surface impressions. Educational institutions, journalists, and researchers benefit significantly from integrating the Bias Meter into their workflows, leading to higher standards of information integrity.
However, it is essential to balance the use of this tool with an awareness of its limitations. While the Bias Meter provides invaluable insights, users must consider external factors such as cultural contexts and the evolving nature of language used in various discourses.
Ultimately, this section reaffirms the importance of continuing the dialogue surrounding bias detection tools. As biases evolve, so too must our methods of evaluation. Therefore, a commitment to refining the Bias Meter is required to meet the challenges posed by an increasingly complex information landscape.
"A tool is only as effective as the user’s understanding of it; the Bias Meter demands a thoughtful approach to its application."
Through this Enlightenment about the Bias Meter, we move towards a more discerning consumption of information, fostering a culture that values accuracy and integrity.
Summarizing Key Insights
In summary, the key insights conveyed throughout the article highlight several crucial points:
- The Bias Meter serves as an essential tool for evaluating information, particularly in journalism and academic research.
- Understanding the methodologies that drive bias detection enhances the reliability of the data we consume.
- Ethical concerns around bias measurement are significant; users must be aware of potential misuse and strive for objectivity in their assessments.
- Continuous improvement and adaptability of the Bias Meter are fundamental to maintaining its relevance in a changing digital landscape.
These insights establish a foundational understanding of how the Bias Meter can shape our approach to information consumption, thereby enhancing the quality of discourse in society. Each stakeholder, from educators to digital content creators, plays a role in this process, reaffirming that the evaluation of bias transcends the individual to impact collective knowledge.