Exploring Causation Relationships: A Comprehensive Analysis
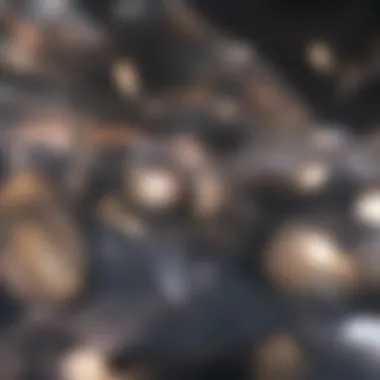
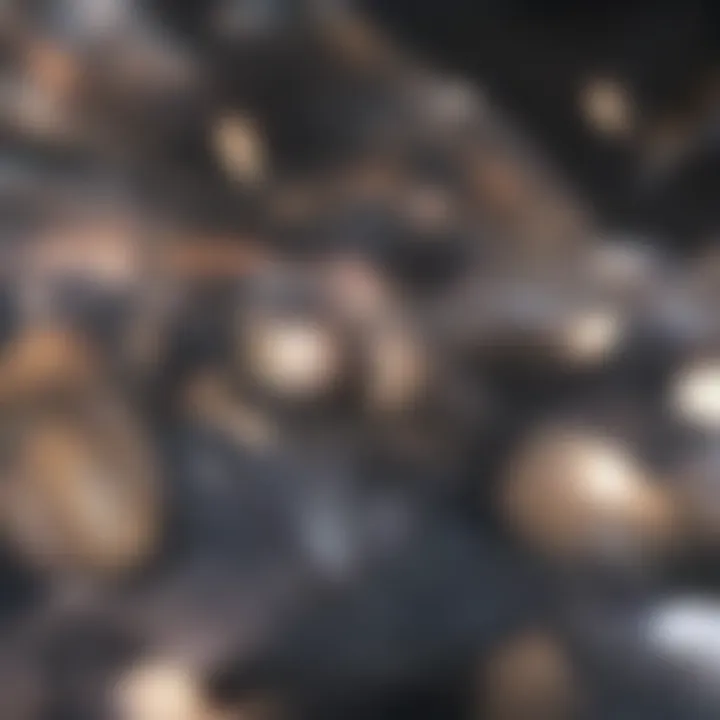
Intro
Causation relationships constitute a fundamental concept across numerous scientific disciplines. Understanding these relationships helps unveil the mechanisms that drive processes, whether biological, physical, or social. Often, the confusion surrounding causation versus correlation leads to significant misinterpretations of data. This article will explore these intricate relationships to enhance clarity and foster informed discussions among students, researchers, educators, and professionals.
Research Background
Overview of the scientific problem addressed
The distinction between causation and correlation is critical in research. Causation implies a direct cause-and-effect relationship, while correlation simply indicates a connection between two variables without implying that one causes the other. Misunderstanding causation can lead to flawed conclusions, incorrect policy decisions, or inadequate research outcomes. The blurred lines between these concepts are prevalent across fields like biology, physics, and social sciences where interpreting data accurately is essential.
Historical context and previous studies
Historically, the philosophical roots of causality can be traced back to thinkers like Aristotle, who first examined the nature of causes in various contexts. Over time, the notion of causation evolved into a more empirical understanding. The works of philosophers such as Hume and Kant laid the groundwork for modern interpretations of causality, influencing fields like psychology and economics.
In contemporary research, numerous studies have attempted to clarify the nuances of causal relationships using statistical methods. For instance, the development of the Granger causality test in econometrics marked a significant advancement in differentiating mere correlations from causative factors. Furthermore, causal inference methodologies, such as those arising from Judea Pearl's work, are pivotal in contemporary data science and research methodology, encouraging more robust findings across various disciplines.
Findings and Discussion
Key results of the research
This article highlights various frameworks and approaches used to establish causation. Empirical studies have shown that statistical measures alone cannot sufficiently confirm causation without understanding the context. Contextual factors, experimental designs, and temporal precedence are critical components in establishing a valid causal link.
Interpretation of the findings
The interpretation of findings must distinguish between correlation and causation. Researchers should approach data with caution, ensuring they apply rigorous methodologies to avoid misinterpretation. Moreover, the implications of establishing causative links extend beyond research; they impact decision-making in policy and practice across industries. Understanding these relationships is vital to avoiding biases and errors in reasoning.
"Understanding causation goes beyond mere data interpretation; it shapes how we understand the world in scientific inquiry."
By synthesizing the discussed information, a clearer picture emerges regarding the complexities of causation relationships. Ultimately, this knowledge equips researchers and practitioners with the tools they need to analyze data critically and draw meaningful conclusions across a variety of contexts.
Understanding Causation Relationships
Understanding causation relationships is vital for comprehending how various entities influence one another. This comprehension goes beyond superficial connections, delving into the reasons behind observed phenomena. In many fields, recognizing causation versus mere correlation is crucial. This understanding not only shapes the development of theories but also informs effective decision-making across scientific research and practical applications.
When we investigate causation, we must acknowledge its intricate nature, often requiring thorough analysis. By discerning the differences between types of causation — direct, indirect, and conditional — one can appreciate the multifaceted interplay of factors. This clarity fosters a deeper understanding of how causes lead to specific effects, which can ultimately influence outcomes in real-world scenarios.
Also, exploring causation impacts research methodologies. By structuring experiments to isolate variables, researchers can define causal relationships more clearly. This is essential for progressing knowledge in fields such as biology, physics, and social sciences, where causative factors can lead to significant advancements in understanding underlying processes.
Defining Causation
Causation refers to the relationship between a cause and its effect. It is the idea that certain events or conditions bring about other events or conditions. Establishing causation requires strong evidence that one factor directly influences another. In contrast to correlation, causation suggests a clear, directional influence. Thus, causation is a fundamental concept in scientific inquiry, pivotal for constructing reliable theories and models.
Types of Causation
Direct Causation
Direct causation occurs when a cause leads straight to an effect without any intermediary steps. For example, striking a match directly causes it to ignite. This type of causation is straightforward and easy to identify, which makes it popular in many scientific discussions. The key characteristic of direct causation is its unambiguous relationship. It simplifies analysis by allowing one to trace effects back to specific causes. However, one limitation is that such clear relationships are not always present in complex systems, making direct causation less applicable in those contexts.
Indirect Causation
Indirect causation involves one or more intermediary steps between a cause and its effect. An example includes how a lack of education may lead to poverty through job opportunities. The difference from direct causation is significant because it introduces layers of complexity. This type is often beneficial for comprehensively analyzing intricate scenarios. It broadens understanding but may complicate the task of establishing clear causal links. As a result, researchers need to consider numerous factors when exploring indirect causation.
Conditional Causation
Conditional causation is when a cause produces an effect only under certain conditions. For instance, heavy rain leads to flooding, but only if the ground is saturated. This type is beneficial for discussing situations where context matters. It highlights that not all causes have uniform effects, which can lead to more tailored conclusions in various fields. However, it makes establishing causation more challenging, as researchers must account for various potential conditions that influence outcomes.
Causation vs. Correlation
The distinction between causation and correlation is a critical aspect of scientific inquiry. Correlation suggests a relationship between two variables, but it does not imply that one causes the other. For instance, ice cream sales and drowning incidents may rise together in summer months, but purchasing ice cream does not lead to drowning. Understanding this difference is crucial to avoid misinterpretation of data and making fallacious conclusions about causal relationships.
The Role of Time in Causation
Time is an essential element in establishing causation. Causative events must occur in a logical sequence. For example, for an action to be considered a cause, it should precede the effect in time. This temporal order is vital for discerning causal links effectively. Additionally, the time frame for observing effects can vary, sometimes leading to delayed outcomes. Recognizing these time-related factors is key for robust analysis of causation relationships.
Historical Perspectives
Understanding historical perspectives on causation is essential as it provides context for current theories and methodologies. This section delves into the foundational ideas that have shaped the evolution of causation studies. Recognizing how past philosophers and scientists approached causation allows contemporary researchers to refine their frameworks and address existing challenges.
Philosophical Foundations
The philosophical underpinnings of causation are crucial in shaping modern inquiries. By examining historical thoughts, one gains insight into how causation has been interpreted through the ages.
Nozick's Theory of Causation
Robert Nozick's theory presents a particular focus on the idea of causation linked with conditions and counterfactual scenarios. A key characteristic of Nozick's approach is the emphasis on how one event can be seen as a cause of another, provided certain theoretical conditions are satisfied. This approach is beneficial in establishing a nuanced understanding of causation that incorporates real-world implications.
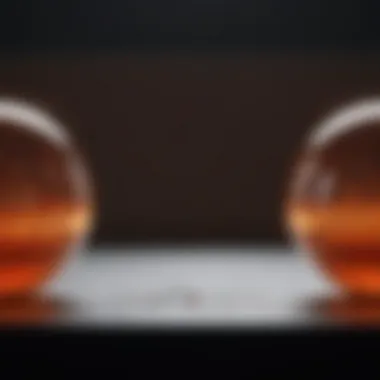
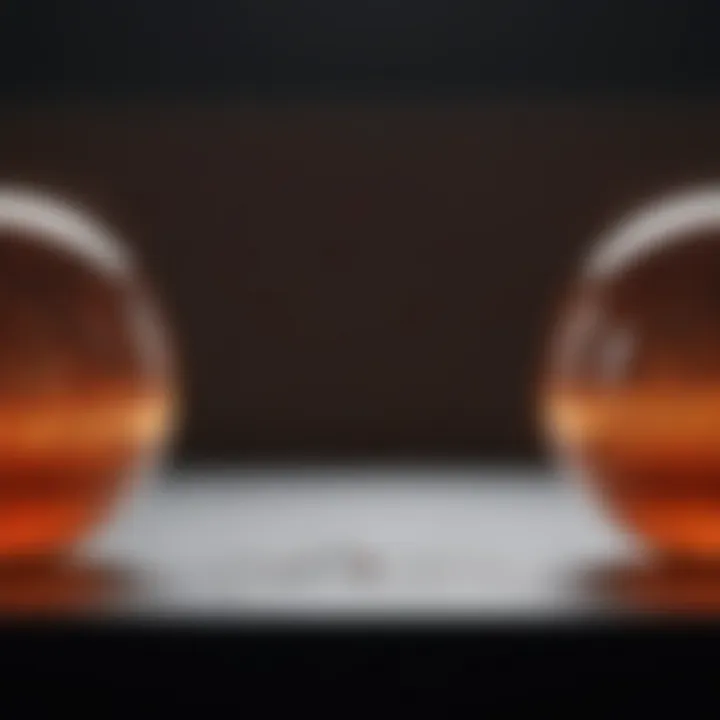
However, the unique feature of Nozick's theory lies in its reliance on the idea of "what could have been" rather than just what has occurred. This counterfactual reasoning aids in exploring causal relationships effectively, but it can also introduce complications when many variables interplay in a situation.
Hume's Problem of Induction
David Hume's work presents a skeptical view of how we infer causation from observed events, emphasizing that just because two events often occur together does not mean one causes the other. This perspective strongly influences contemporary discussions about causation, particularly in the context of scientific reasoning. Hume's detailing of this problem underscores the inherent uncertainties in establishing reliable causal links, making it a popular topic in philosophical debates.
The significant characteristic of Hume's argument lies in its critique of inductive reasoning, which he saw as deeply flawed. While a strong philosophical foundation, the limitation of Hume's theory is that it may lead to a sense of doubt in our ability to comprehend causative relationships fully, complicating discussions in this field.
Development of Causation Theories
This subsection addresses how causation theories have advanced over time, bridging historical context into modern applications. Exploring early and modern paradigms reveals the trajectory of causation studies and their ongoing evolution.
Early Scientific Approaches
The early scientific approaches to causation laid the groundwork for subsequent inquiries, often characterized by empirical observation and initial attempts at systematic experimentation. A noteworthy aspect of these early attempts is their reliance on observable data to determine cause and effect, setting a precedent for future scientific methodologies.
These approaches are significant as they highlight the fundamental role of experimentation in understanding the natural world. While early scientists effectively established basic causal relationships, the unique feature of their approaches rests in their limitations—lack of sophisticated statistical tools and methodologies to analyze complex interactions among variables.
Modern Paradigms
Modern paradigms concerning causation have broadened to encompass advanced statistical techniques and interdisciplinary insights. One major characteristic of this development is the integration of technological advancements that facilitate complex causal analysis. Such integration makes methodologies accessible and applicable to various fields, from social sciences to physics.
These paradigms stand out as beneficial due to their flexibility and the ability to adapt to evolving data landscapes. However, a unique feature is how they grapple with existing uncertainties and the potential for misinterpretation, which can lead to challenges in establishing clear and replicable causal links.
In summary, examining historical perspectives on causation not only enriches our understanding of the field but also highlights the necessity for ongoing critical assessment in current methodologies and theories.
Methodologies for Establishing Causation
Understanding causation requires rigorous methodologies that allow researchers to ascertain links between variables. Methodologies help in differentiating mere correlations from genuine causal relationships. By employing established research methods, one can avoid common pitfalls of misinterpretation. These methodologies serve as necessary tools that provide clarity and structure.
Experimental Design
In many fields, experimental design is crucial for establishing causative links. This often involves controlled conditions where variables can be managed effectively. The success of an experiment heavily relies on the systematic comparison of different conditions to determine the impact of a specific factor.
Control Groups
Control groups are an essential aspect of experimental design. They serve as a baseline against which the treatment or experimental group is compared. The key characteristic of control groups is that they undergo the same procedures as the treatment group, except for the exposure to the independent variable.
The beneficial nature of control groups lies in their ability to minimize the influence of confounding variables. This makes them a popular choice for methodologies focused on causation.
Control groups provide a unique feature of isolating the effect of the independent variable, allowing researchers to draw more reliable conclusions. However, reliance on control groups can become a disadvantage when it comes to ethical concerns in real-life applications, or when it is impractical to withhold treatment from a group expected to benefit.
Randomized Trials
Randomized trials are a cornerstone of evidence-based research. They involve randomly assigning participants to either the treatment group or the control group. The primary benefit of this randomization is that it helps eliminate selection bias, making the results more generalizable.
A key characteristic of randomized trials is their structure, which promotes an unbiased distribution of factors that might confound outcomes. This beneficial quality enhances the internal validity of the study.
However, randomized trials may face ethical dilemmas, especially in behavioral social sciences. Their unique feature of random assignment can be a disadvantage in certain contexts, where not all variables can be controlled, thus impacting the causal inference.
Observational Studies
When experimental design is not feasible, observational studies provide an alternative method for establishing causation. These studies observe variables without manipulation, focusing on natural variations which can still yield significant insights into causal relationships.
Longitudinal Studies
Longitudinal studies follow the same subjects over time, allowing researchers to observe changes and developments in variables. The key aspect of these studies is the ability to track causation over extended periods, which helps in inferring long-term impacts.
A beneficial aspect of longitudinal studies is that they can provide insights into trends and dynamics that might be missed in cross-sectional studies. These studies, however, require substantial time and resources, making them less practical in some research contexts. The unique feature of repeated measures illustrates change, but it can also lead to participant dropout, creating potential biases.
Cross-sectional Studies
Cross-sectional studies analyze data from a population at a specific point in time. They measure the relationship between variables at that one moment, providing a snapshot rather than a continual evolution.
The key characteristic of cross-sectional studies is their efficiency; they are quicker and less costly compared to longitudinal studies. This makes them a beneficial choice for many researchers who need to gather preliminary information.
However, their unique feature of assessing causation at a single time point limits the ability to draw conclusions about long-term effects. This restriction can lead to misconceptions about causality, making careful consideration necessary when interpreting results.
By utilizing these varied methodologies, researchers can better navigate the complex landscape of causation. Each method has its own strengths and weaknesses that contribute to a more nuanced understanding of causality in various fields.
Applications Across Disciplines
Understanding causation relationships is crucial across various disciplines, as it enables a deeper grasp of interactions within systems. Each field brings its own unique perspective to causation, providing insights that can enhance practical applications in areas like healthcare, technology, and social policies. This section will explore three significant disciplines: biology, physics, and social sciences, showcasing how causation shapes understanding and decision-making processes in these areas.
Causation in Biology
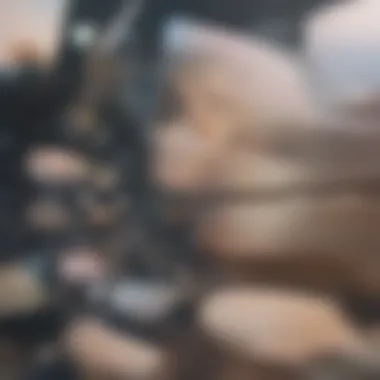
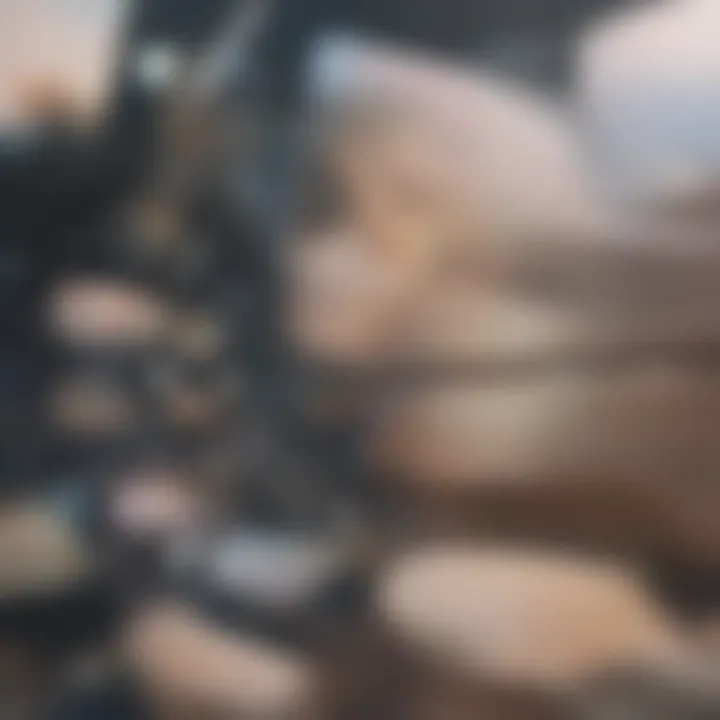
Genetic Causation
Genetic causation is a fundamental concept within biology that addresses how genes influence traits and behaviors. Genetic factors often dictate physical characteristics, susceptibility to diseases, and even certain behaviors. This aspect is significant because it allows researchers to identify potential genetic disorders that could affect populations. Moreover, genetic causation provides insight into how inherited traits can affect an individual's overall health.
A key characteristic of genetic causation is its focus on heredity. Tracking genetic predisposition helps in understanding populations and developing effective strategies for combating genetic diseases. This makes it a popular choice for researchers aiming to make advances in medical genetics. However, while it is beneficial for identifying genetic risks, it can oversimplify the complex interactions between genes and environmental factors. This complexity can lead to challenges in treatment and advising patients regarding their health.
Ecological Interactions
Ecological interactions delve into how different organisms affect one another within ecosystems. These interactions can range from predator-prey dynamics to symbiotic relationships, showcasing the intricate web of life. Understanding these relationships sheds light on biodiversity and ecosystem stability.
A standout feature of ecological interactions is the emphasis on community-level analysis. This perspective is crucial because it enables scientists to anticipate the impact of species loss or introduction on ecosystems. Ecological interactions are beneficial for conservation efforts but come with the challenge of defining and isolating interactions that may be influenced by various external factors, like climate change.
Causation in Physics
Causative Laws
Causative laws play an essential role in physics, outlining the principles governing the natural world. These laws allow scientists to predict outcomes based on known variables, which aids in various fields, from engineering to environmental science. A significant key feature is their deterministic nature, often allowing for precise predictions under controlled conditions.
This feature makes causative laws a reliable foundation for scientific experimentation. However, while they provide consistency, they can also restrict understanding in chaotic or complex systems where outcomes are less predictable. In such cases, the applicability of these laws may diminish, leading to a need for alternative approaches.
Thermodynamics
Thermodynamics explores the relationships between heat, energy, and work. Key aspects of thermodynamics include its laws governing energy conservation and entropy. The implications of these principles are foundational in engineering disciplines, influencing the design of engines and refrigerators, among other systems.
Thermodynamics is a beneficial aspect of physics since it has widespread application in both theoretical and practical realms. Its unique feature lies in its ability to apply predictive models to a variety of physical systems. However, the complex mathematical formulations can pose a challenge for those not deeply versed in physics.
Causation in Social Sciences
Behavioral Causation
Behavioral causation examines the factors that influence human actions and societal trends. This area is crucial for understanding phenomena such as addiction, social behavior, and decision-making. It combines elements from psychology, sociology, and economics to analyze the underlying reasons for behaviors.
A characteristic of behavioral causation is its interdisciplinary approach, allowing researchers to draw from multiple fields to gain a comprehensive understanding. This framework is beneficial as it enables more nuanced insights into societal issues. Nevertheless, it often faces challenges related to the complexity and variability of human behavior, making predictions difficult.
Causation in Economics
Causation in economics addresses how various factors influence economic outcomes, such as inflation, employment, and consumer behavior. Understanding these relationships can assist policymakers in making informed decisions regarding fiscal policies and societal welfare.
The key feature is its reliance on empirical data and statistical analysis to hypothesize and test economic theories. This makes it a pivotal element in economic research. However, the challenge lies in correlating causative factors with economic indicators, as the economy is influenced by numerous interrelated variables, complicating the establishment of clear cause-effect relationships.
Challenges in Causation Research
The exploration of causation relationships is inherently complex. Among the pivotal areas examined, the challenges in causation research stand out for their significant implications for understanding cause and effect. These challenges often obscure the truth behind phenomena in various fields, hence their analysis is crucial. They not only take shape in the form of complex systems but also involve the misinterpretation of data. Acknowledging them can lead to sharper clarity and effectiveness in research methodologies.
Complex Systems
Complex systems can present challenging barriers to establishing clear causative links. Their nature often involves multiple interacting variables, making it hard for researchers to identify true relationships.
Interconnected Variables
Interconnected variables are key components of complex systems. They signify that changes in one variable affect others, creating chains of cause and effect. This interdependency can lead to misleading conclusions if not properly understood.
The key characteristic of interconnected variables is their intrinsic link to the systems they operate in. Researchers often choose to focus on them because they reveal the multilayered and intricate relationships that influence outcomes. A unique feature of this concept is that it encourages a multi-dimensional approach to analysis. It highlights not just direct influences but also indirect ones that can emerge from these relationships. However, the challenge here lies in managing the overwhelming volume of data which can lead to confusion in interpretation.
Feedback Loops
Feedback loops present another layer of complexity in causation research. These loops signify processes where the output is circled back into the system as input. This can amplify certain behaviors or nullify others, complicating the identification of causal pathways.
The key characteristic of feedback loops is their ability to create dynamic interactions among variables. This makes them an attractive focus for researchers seeking to understand delayed effects or recurrent patterns. The unique feature of feedback loops is their potential to modify outcomes based on previously established variables. However, their self-reinforcing nature may obscure the clarity one seeks in causation, often leading to cyclical interpretations that can misrepresent the actual cause-effect relationships.
Misinterpretation of Data
Misinterpretation of data remains a critical hurdle. It often arises from flawed analytical techniques or oversight in the research design. Two notable aspects of this challenge are overgeneralization and the presence of spurious relationships.
Overgeneralization
Overgeneralization occurs when conclusions drawn from a specific dataset are erroneously applied to broader contexts. This can lead to significant misjudgments regarding causative factors in various fields.
The key characteristic of overgeneralization is its rootedness in limited observations. It is often considered a common pitfall in research due to the temptation to simplify findings for wider applicability. A unique feature of this concern is the potential to overlook context-specific variables that may be crucial for comprehension. The major disadvantage here is the risk of promoting erroneous beliefs, thereby skewing future research directions.
Spurious Relationships
Spurious relationships refer to situations where two variables appear to be causally linked but are actually influenced by an outside factor. This phenomenon can severely hinder genuine causal analysis.
The key characteristic of spurious relationships is their deception. They often manifest as statistical correlations, which attract attention without revealing true causation. Spurious relationships commonly arise from poor study design, leading to the incorporation of confounding variables. The unique feature of this issue is how it threatens the integrity of scientific conclusions. Researchers must be vigilant to avoid misinterpretation caused by these misleading associations.
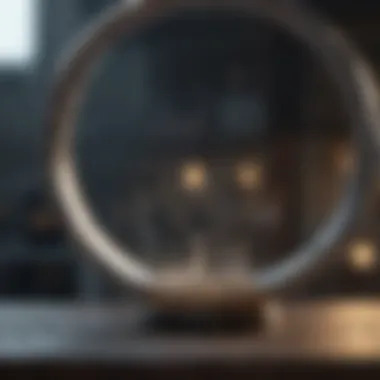
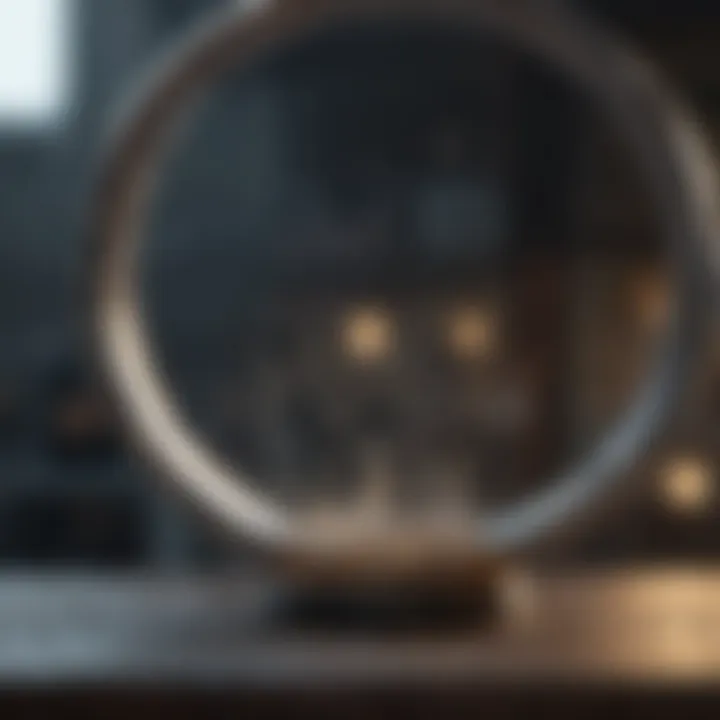
Understanding these challenges is essential for robust causation analysis. The complexities of interconnected variables, feedback loops, and misinterpretation can cloud the path to accurate insights. Only through meticulous scrutiny can researchers uncover the intricacies of causation and enhance the reliability of their conclusions.
Frameworks for Analyzing Causation
Understanding causation necessitates a structured approach. Frameworks for analyzing causation provide essential tools and methodologies that allow researchers to accurately assess and interpret causal relationships. These frameworks can offer insights into how and why certain effects occur, guiding researchers in their inquiry.
The importance of these frameworks extends beyond simple analysis. They facilitate a systematic evaluation of complex relationships, often involving multiple variables. Understanding how these systems interact helps prevent misinterpretation of results, which is a common issue in causation research. Moreover, they serve as a common language across disciplines. This commonality helps in collaboration among scientists, researchers, and educators, all striving to untangle the web of causation.
The frameworks not only highlight connections but also clarify the implications of findings. By delineating causative factors from mere correlations, they establish a more robust understanding of phenomena across various fields. In essence, these analytical structures are foundational to advancing knowledge and fostering informed discussions.
Causal Inference Techniques
Bayesian Approaches
Bayesian approaches are pivotal in causation studies. They employ Bayes’ theorem to update the probability of a hypothesis as more evidence becomes available. This technique's key characteristic is its use of prior knowledge and current evidence, which allows researchers to refine their conclusions continuously. This iterative process makes Bayesian methods especially beneficial for dynamic research environments where new information frequently alters the context.
A unique feature of Bayesian approaches is their flexibility in handling uncertainties. Unlike traditional methods, they enable researchers to incorporate various sources of information and beliefs. This adaptability results in more precise estimations of causal relationships, although it requires careful consideration of prior distributions.
Advantages include a comprehensive view of uncertainty, making them suitable for complex models. However, the need for precise prior information can also be a disadvantage, as poor choices can skew results significantly, leading to incorrect interpretations.
Structural Equation Modeling
Structural Equation Modeling (SEM) is another powerful tool in analyzing causation. This technique allows for the testing of complex relationships among observed and latent variables. A prominent characteristic is its ability to represent intricate theoretical models that involve multiple relationships simultaneously. This complexity is often why SEM is a favored choice for researchers in various disciplines, including social sciences and psychology.
SEM's unique advantage lies in its capacity to assess both direct and indirect effects, making it valuable for disentangling intricate relationships within data. However, it requires large sample sizes for reliable results, making implementation potentially burdensome in studies with limited participants.
The advantages of SEM include its detailed representation of theoretical constructs. Meanwhile, its disadvantages include the high demands on data quality and sample size, which can be challenging in real-world applications.
Counterfactual Reasoning
Counterfactual reasoning is an essential aspect of causal analysis. This technique involves asking what-if questions to explore alternative outcomes had different conditions been present. By analyzing what could have happened, researchers can infer possible causal relationships more effectively.
This reasoning guides experimental design in various fields, especially in social sciences. It emphasizes understanding the causal mechanisms at play rather than merely observing outcomes. Counterfactuals aid in constructing robust causal narratives, allowing scholars to navigate the complexities of causation with greater clarity.
By integrating frameworks like Bayesian approaches and Structural Equation Modeling, researchers enhance their capacity to analyze causation deeply and accurately. These methodologies not only improve scientific rigor but also enrich interdisciplinary collaboration, ultimately contributing to a finer understanding of causation relationships.
Future Directions in Causation Studies
The study of causation is evolving continually, influenced by advances in technology and our understanding of complex systems. This section elaborates on potential advancements that can shape the future of causation studies, emphasizing technological integration and interdisciplinary collaboration. As researchers seek more precise methods of understanding causal relationships, recognizing emerging tools and collaborative efforts will be crucial in refining our methodologies.
Technological Advancements
Machine Learning Applications
Machine learning is making significant strides in causation studies. A specific aspect worth noting is its ability to process vast amounts of data efficiently. This characteristic enhances the analysis of causative relationships across domains like biology and social sciences. Machine learning algorithms can identify patterns that traditional statistical methods may overlook.
Key Feature: The adaptability of machine learning algorithms allows them to integrate new data continuously, improving their predictive accuracy.
This adaptability makes machine learning a powerful tool for researchers. However, it does come with challenges. One disadvantage is the potential for overfitting, where a model performs well on training data but poorly on unseen data. Understanding these limitations is essential for researchers aiming to leverage this technology effectively.
Data Mining Techniques
Data mining techniques are pivotal in discovering hidden patterns in extensive datasets relevant to causation studies. They help reveal correlations that may suggest underlying causal relationships. A critical aspect of data mining is its ability to summarize and analyze unstructured data, which is prevalent in today’s research.
Key Characteristic: The versatility of data mining tools allows for applications across various fields, making them a popular choice for analysts and researchers.
Data mining supports researchers by providing comprehensive insights into complex datasets. Yet, one must be cautious of the false positives that can arise from improperly cleaning or interpreting the data. A balanced approach is needed to maximize the benefits while mitigating the risks.
Interdisciplinary Collaboration
Collaborative efforts across different disciplines can amplify the study of causation. Interdisciplinary collaboration encourages the sharing of methodologies and ideas, enabling researchers to approach complex problems from multiple perspectives. This synergy not only fosters innovation but also enhances the robustness of findings in causation studies.
The blending of disciplines, such as combining insights from biology, psychology, and computer science, can yield richer and more insightful outputs. This diversity in approach allows researchers to tap into various expertise and foster solutions that may not emerge in isolated study.
Closure
In the realm of Causation Relationships, the conclusion serves as a crucial summation that emphasizes the key implications of this analysis. It highlights the intricate ways in which cause and effect interplay across various disciplines, enhancing our understanding of scientific inquiry. Recognizing the nuances of causation allows researchers and scholars to avoid common pitfalls associated with misinterpretations, particularly the confusion between correlation and actual causative factors.
Summary of Key Insights
This article has explored fundamental aspects of causation, including:
- Defining Causation: Establishing a clear differentiation between what constitutes a cause and what represents an effect.
- Types of Causation: Identifying various forms, such as direct, indirect, and conditional causation, which are vital for contextualizing different scenarios.
- Methodologies: Discussing both experimental and observational approaches to establish causative links effectively.
- Applications Across Disciplines: Illustrating how causation manifests in fields like biology, physics, and social sciences, enhancing cross-disciplinary understanding.
- Challenges: Addressing potential obstacles in causation research and the strategies to mitigate misinterpretations of data.
- Future Directions: Highlighting the role of advancing technologies and interdisciplinary collaboration in shaping the future of causation studies.
These insights underscore the importance of a meticulous approach towards studying causation, encouraging critical thinking and rigorous exploration.
Final Thoughts on Causation Relationships
In final reflection, the concept of causation is not merely an academic exercise but a necessary framework for understanding the world around us. Its importance transcends theoretical discussions and resonates strongly across practical applications.
Understanding causation relationships equips individuals with analytical skills that can be applied to real-world problems. This establishes stronger foundations for developing informed policies in health, science, and economics, fostering nuanced discussions among researchers, educators, and practitioners alike.
Thus, as we move forward, the continued exploration of causation will prove essential in illuminating the complex interactions that define our reality. The consideration given to causative links can pave the way for future discoveries and stimulate innovative solutions to existing challenges.