Exploring the Role of Collective Data Wisdom in Research
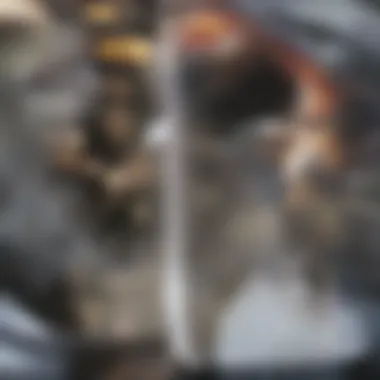
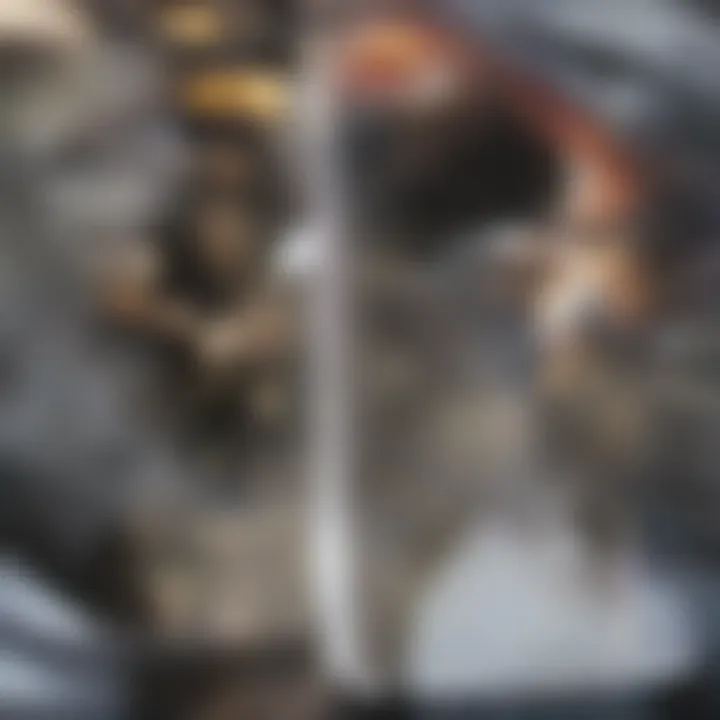
Intro
The notion of Collective Data Wisdom (CDW) is becoming increasingly relevant in today's scientific landscape. As research becomes more complex and diverse, the need to manage and interpret vast amounts of data efficiently has grown. CDW refers to the shared intelligence that arises when individuals collaborate to analyze data. This concept merges insights from various fields, promoting innovation and enhancing the quality of scientific inquiry.
Understanding the implications of CDW goes beyond the mechanics of data analysis. It encompasses philosophical discussions about the nature of knowledge, the ethics of data sharing, and the responsibilities that researchers bear in a collaborative environment. This article aims to unpack these layers while providing practical insights for integrating CDW into modern research practices.
Foreword to Collective Data Wisdom
The concept of Collective Data Wisdom (CDW) holds significant relevance in the context of modern scientific research. CDW emphasizes the synergy that arises when diverse datasets are combined, analyzed, and interpreted collectively. This is not just a technical endeavor but also a fundamental shift in how researchers interact with data across disciplines such as biology, chemistry, and earth sciences.
Understanding CDW fosters a culture of collaboration among researchers, allowing them to extract deeper insights from their collective experiences and knowledge. This collaborative approach enhances the interpretative power of data, leading to more robust conclusions and groundbreaking results. Acknowledging the implications of CDW is essential for recognizing the transformative potential it presents.
Moreover, as data generation continues to escalate, the demand for effective methodologies to harness this data increase. CDW can address this need, offering frameworks for data sharing that are ethical and beneficial. In a landscape where individual research efforts often fall short of holistic understanding, CDW becomes a pivotal mechanism facilitating progress.
Definition and Conceptual Framework of CDW
Collective Data Wisdom refers to the process of leveraging aggregated data from multiple sources to derive insights that are richer than those generated from isolated datasets. At its core, CDW centers around the principles of collaboration, integration, and interpretation. The conceptual framework of CDW prioritizes shared understandings and emphasizes the contextual value of data in relation to other datasets. This means that the relevance of data is not merely derived from its individual worth but from its interplay with additional information.
In practice, this framework encourages the development of collaborative platforms that facilitate the pooling of data resources. Researchers are encouraged to share findings, methodologies, and data insights in an effort to foster a culture of transparency and openness. CDW thus serves not only as a transformative tool for data interpretation but also as an ethos supporting collective inquiry.
Historical Context and Evolution of CDW
The evolution of CDW can be traced back to the increasing need for interdisciplinary research in the face of complex global challenges. Early scientific inquiries were often confined to singular disciplines, resulting in fragmented knowledge pools. However, as science advanced, researchers realized that many challenges could not be solved through isolated efforts alone. This realization has led to the gradual incorporation of collaborative approaches in research practices.
Over the past two decades, the digital revolution has also spurred the evolution of CDW. The rapid proliferation of data and advancements in analytical tools has allowed researchers from various fields to easily access and utilize shared datasets. Initiatives like the Human Genome Project exemplify how combined efforts can lead to groundbreaking scientific discoveries. The historical trajectory of CDW demonstrates its ever-growing importance as a paradigm shift in research, underscoring the need for a collective approach in the current scientific landscape.
"Data wisdom cannot exist in silos. It thrives on shared knowledge and communal insight, reshaping our understanding of what scientific inquiry can achieve."
This foundational understanding of CDW highlights the framework's capacity to facilitate interdisciplinary collaboration and innovation—two central themes that will be explored further in subsequent sections.
The Role of CDW in Scientific Advancement
Exploring the implications of Collective Data Wisdom requires understanding its pivotal role in modern scientific advancement. CDW not only serves as a method for integrating vast amounts of data but also facilitates a more nuanced interpretation of this information. By leveraging collaborative efforts across multiple disciplines, researchers can unlock new insights that would be impossible when working in silos. This interconnectedness is vital as it can directly lead to breakthroughs in various scientific fields.
Enhancing Data Interpretation
One of the primary benefits of Collective Data Wisdom is the enhancement of data interpretation. Traditional methods often struggle with the sheer volume and complexity of contemporary datasets. With CDW, scientists can combine their expertise, allowing for deeper insights. When researchers pool their knowledge, they create a more robust framework for understanding patterns and trends within the data.
For example, in genomics, when biologists share genomic data with data scientists, it enables a more sophisticated analysis of genetic variants. This collaboration can lead to more accurate disease models and treatment options.
Moreover, this shared pool of wisdom encourages diverse perspectives on data analysis. A varied approach leads to the discovery of hidden correlations and can challenge existing hypotheses.
Fostering Interdisciplinary Collaboration
CDW promotes interdisciplinary collaboration, which is necessary for addressing complex scientific challenges. Many real-world problems do not confine themselves to one discipline; thus, researchers need to work together across fields.
For instance, the intersection of biology, chemistry, and computer science is critical in areas like bioinformatics. In this field, biologists work closely with computer scientists to develop algorithms that can analyze biological data efficiently. Such collaboration helps in the creation of innovative solutions that improve understanding and treatment of biological issues.
Furthermore, fostering a culture of teamwork contributes to a more inclusive scientific environment. This inclusivity leads not only to richer research outcomes but also to the development of a new generation of scientists who appreciate diverse methodologies and viewpoints.
Driving Innovation in Research Methodologies
The integration of CDW significantly drives innovation across research methodologies. By creating a shared database of insights and methodologies, researchers can adopt and adapt existing techniques to their projects. This cross-pollination of ideas often results in the development of new experimental techniques or the refinement of old ones.
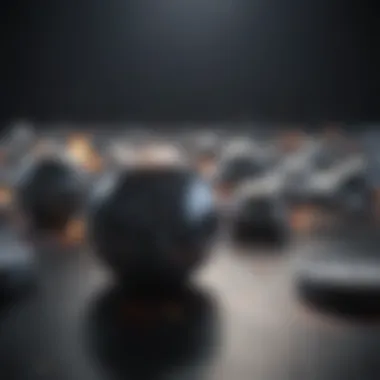
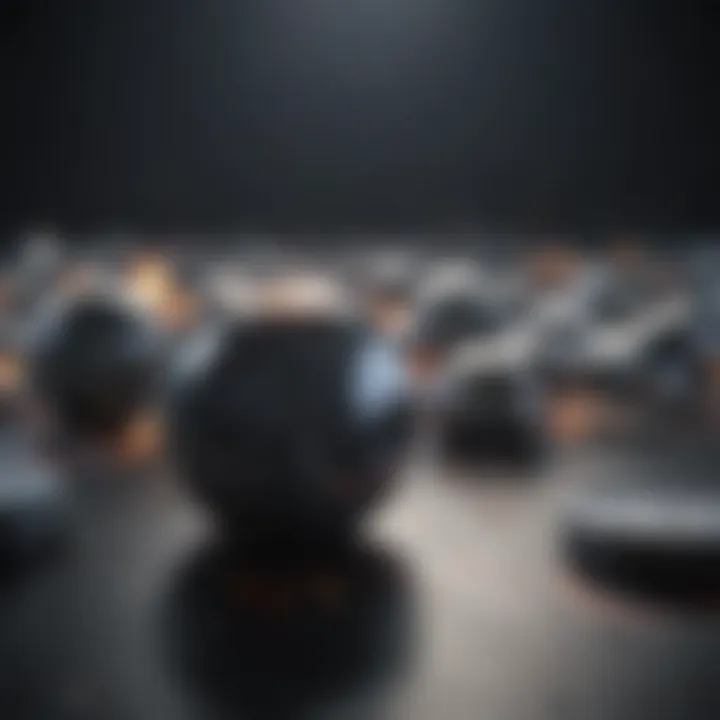
Consider the field of materials science. Researchers utilizing CDW can share findings on material properties and processing methods. This can accelerate advancements in developing novel materials for various applications, such as renewable energy technologies or biocompatible devices.
In addition, CDW encourages the adoption of advanced tools like machine learning for data analysis. The power of these tools can be harnessed more efficiently when there is a collaborative framework in place, leading to innovative strategies that propel research forward.
"The integration of diverse data sources and expertise is crucial for realizing the full potential of scientific discovery."
In summarized form, the role of CDW in scientific advancement cannot be overstated. It enhances data interpretation, encourages interdisciplinary collaboration, and drives innovation in methodologies. These factors collectively contribute to a more dynamic and effective research landscape. As researchers embrace the principles of CDW, they will become better equipped to tackle the increasingly complex issues facing society today.
Challenges in Implementing CDW
The implementation of Collective Data Wisdom (CDW) brings significant advantages to scientific research, yet it also presents certain challenges that must be meticulously navigated. Understanding these challenges is crucial for effectively integrating CDW into research processes. Addressing issues related to data quality, ethical considerations, and technical barriers not only enhances the reliability of research outcomes but also ensures responsible data practices.
Data Quality and Reliability Issues
One of the foremost challenges in implementing CDW is ensuring the quality and reliability of the data being utilized. When harnessing data from various sources, researchers can encounter inconsistencies, inaccuracies, or incomplete datasets. These problems can lead to misguided conclusions or even halt advancements in scientific knowledge.
To tackle this challenge, it is imperative to establish robust data validation protocols. Employing standardized methods for data collection and analysis can enhance the integrity of the findings. Moreover, continuous monitoring and updating of datasets contribute to maintaining their relevance and accuracy.
Ethical Considerations in Data Sharing
Another vital consideration revolves around the ethical dimensions of data sharing. The promise of CDW lies heavily in collaboration and open access; however, this raises questions regarding privacy, informed consent, and ownership of data. Researchers must navigate these ethical waters carefully to avoid potential pitfalls that might lead to reputational damage or legal implications.
An effective approach involves the implementation of clear guidelines on data sharing. Collaborative frameworks that prioritize the rights of individuals and institutions offer a pathway to ethically sound practices. Highlighting the importance of transparency in these processes also fosters trust among research communities and enhances the collective wisdom derived from shared data.
Technical Barriers to Integration
Technical challenges also play a significant role in the successful application of CDW. Data from disparate sources often exists in various formats, making integration complex. Compatibility issues among different data management systems can hinder efficient analysis, resulting in delays and frustration in research activities.
To overcome these technical barriers, researchers can invest in advanced data integration tools. Utilizing application programming interfaces (APIs) can facilitate smoother data sharing and interoperability between systems. Additionally, training for researchers to use these technical tools effectively can promote smoother transitions to collaborative practices and ultimately enrich the overall research environment.
"Understanding the challenges of implementing CDW is essential for researchers aiming to harness its full potential. Addressing data quality, ethical issues, and technical integration fosters a more productive research landscape."
CDW and Data Governance
Data governance is a critical aspect of implementing Collective Data Wisdom (CDW) in modern scientific research. It refers to the overall management of the availability, usability, integrity, and security of data used in the context of research. The establishment of robust data governance frameworks is essential to ensure that the potential of CDW is fully realized while maintaining ethical standards. This balance is vital because it not only enhances research efficiency but also protects the interests of individuals and organizations involved in data sharing.
A well-defined data governance structure can help researchers navigate the complexities of data utilization and collaboration. It provides clear guidelines on how data should be handled, shared, and stored. Effective governance also promotes trust among data providers and users, enabling seamless collaboration across various disciplines. Furthermore, it ensures adherence to legal and regulatory requirements that may vary across regions. As the body of available data grows, the need for structured governance becomes even more pronounced.
Establishing Standards for Data Use
Setting standards for data use is foundational in creating a conducive environment for CDW. These standards define the protocols for data collection, storage, sharing, and usage. By establishing clear standards, researchers can enhance data interoperability, which is crucial for collaborative endeavors. When diverse datasets conform to agreed-upon formats and protocols, it greatly simplifies their integration into collective frameworks.
Some key aspects of standards for data use include:
- Data Ownership and Attribution: Clearly outlining who holds ownership of the data and how it is to be legally attributed when used in research publications.
- Data Privacy and Security: Ensuring protocols are in place to protect sensitive data from unauthorized access.
- Quality Assurance: Implementing measures to verify the reliability and accuracy of data before it is shared.
The successful establishment of these standards fosters an environment where researchers feel confident about sharing their data, knowing it will be handled responsibly.
Roles of Institutions and Organizations
Institutions and organizations play pivotal roles in fostering effective data governance related to CDW. They not only provide guidance on policies and procedures but also serve as enablers of best practices in data management and sharing. Research institutions, universities, and various governmental bodies can help in creating a supportive infrastructure for data governance.
Here are some key roles they play:
- Policy Development: Institutions are tasked with creating comprehensive policies that outline the ethical use of data and the imperative of transparency in data sharing.
- Training and Support: Providing training sessions for researchers on data management practices, helping them navigate the complexities of data sharing and ensuring compliance with established standards.
- Facilitating Collaboration: Institutions can create platforms that encourage collaboration between academic and industry researchers, allowing for broader access to datasets, thus enhancing the scope of research.
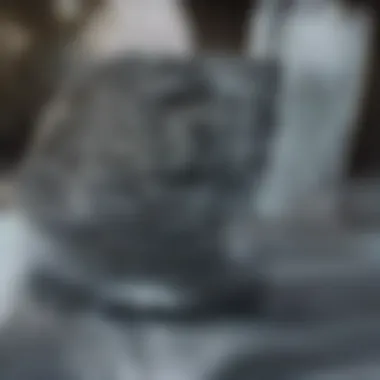
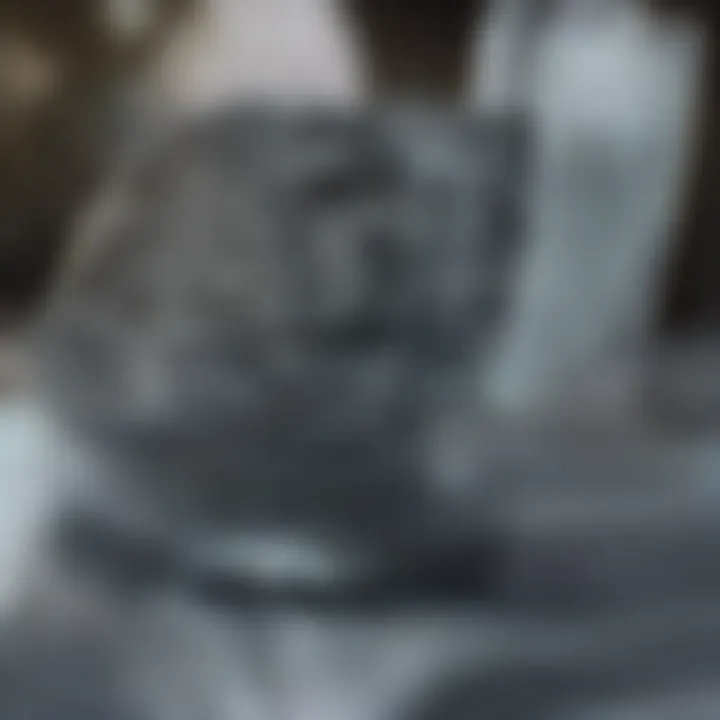
Competitive and collaborative research environments benefit from proactive institutional support that champions the importance of data governance.
In essence, governance serves as the backbone that holds the network of CDW together, allowing for efficient data use while safeguarding ethical responsibilities.
The intersection of CDW and data governance signifies not just a trend but a necessary evolution in how scientific research is conducted in the modern era. Entering a new phase of research collaboration goes hand-in-hand with establishing an environment built on trust, shared standards, and institutional support.
Case Studies of CDW in Action
The concept of Collective Data Wisdom (CDW) becomes significantly concrete when explored through real-world case studies. These examples serve to illustrate its practical implications, demonstrating both the advantages and challenges of implementing CDW across diverse scientific fields. Examining case studies not only highlights successful strategies, but also provides critical analyses that can inform future applications of CDW.
Successful integrations of CDW can lead to breakthroughs in research, optimization in processes, and enhanced collaborative efforts among scientists. In these instances, data acting collectively often yields more insightful results than isolated data streams.
Successful Implementations in Biology
In the biological sciences, numerous successful implementations of CDW exist that underscore its significance. A prime example is the Global Biodiversity Information Facility (GBIF). This initiative consolidates biodiversity data from various sources, allowing researchers worldwide to access extensive datasets.
The GBIF platform utilizes CDW by pooling data from independent researchers, museums, and government agencies. The benefits include:
- Increased accessibility to crucial biological data.
- Enhanced data quality, as diverse sources help identify inaccuracies or gaps.
- Support for widespread research, as any scientist can leverage this comprehensively curated data.
Consequently, researchers can deliver more informed conservation strategies and contribute significantly to understanding biodiversity in ecosystems.
CDW Applications in Chemistry Research
In the realm of chemistry, the Chemical Abstracts Service (CAS) is a compelling case study. This database integrates vast amounts of chemical literature and data, allowing researchers to discover trends and correlations that may not be visible in isolation.
Key aspects of CDW in chemistry research include:
- Comprehensive databases, which provide a holistic view of existing knowledge and discoveries.
- Facilitated collaboration among chemists across different sectors who can access shared data.
- Informed decision making enabled by aggregated insights that stem from collective datasets.
This structured database not only accelerates research but also assists in innovations in drug development and materials science, optimizing the path from concept to application.
Innovative Uses in Earth Sciences
When considering the Earth sciences, the use of CDW can be seen vividly in climate modeling. The Community Earth System Model (CESM) stands out as an example that harnesses CDW effectively. It draws upon extensive datasets from multiple sources, allowing researchers to simulate and predict climate behavior on a global scale.
Through its applications, the key benefits of employing CDW in Earth sciences are clear:
- Improved accuracy of climate predictions through data consolidation.
- Cross-disciplinary collaboration, linking oceanography, meteorology, and ecology.
- Real-time data integration, enabling timely responses to evolving climate patterns.
These applications of CDW lead to rich insights that help researchers develop robust climate policies.
By showcasing specific implementations across various disciplines, we see the transformational power of CDW. It is not merely an abstract concept but a functional framework that can redefine research practices.
Implementing CDW in Research Practices
Implementing Collective Data Wisdom (CDW) in research practices is pivotal for the future of scientific inquiry. CDW allows scientists to leverage a vast pool of data collected from diverse sources. Doing so enhances interpretation, aids collaborative efforts, and drives innovation. As the volume and complexity of data continue to grow, the importance of adopting CDW practices becomes increasingly evident. Each part of this process contributes significantly to the overall efficiency and effectiveness of scientific research.
Developing Collaborative Platforms
Creating collaborative platforms is essential for the successful implementation of CDW. These platforms facilitate sharing of information among researchers, making access to data easier. By doing so, they help overcome the silos often found in disciplinary research. Platforms such as GitHub, Open Science Framework, and ResearchGate are examples of tools that promote collaboration.
When these collaborative platforms are well-designed, they can host discussions, share methodologies, and connect data contributors. Important benefits include:
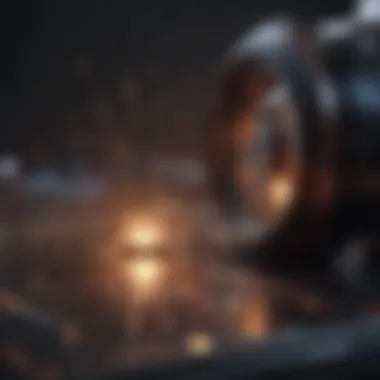
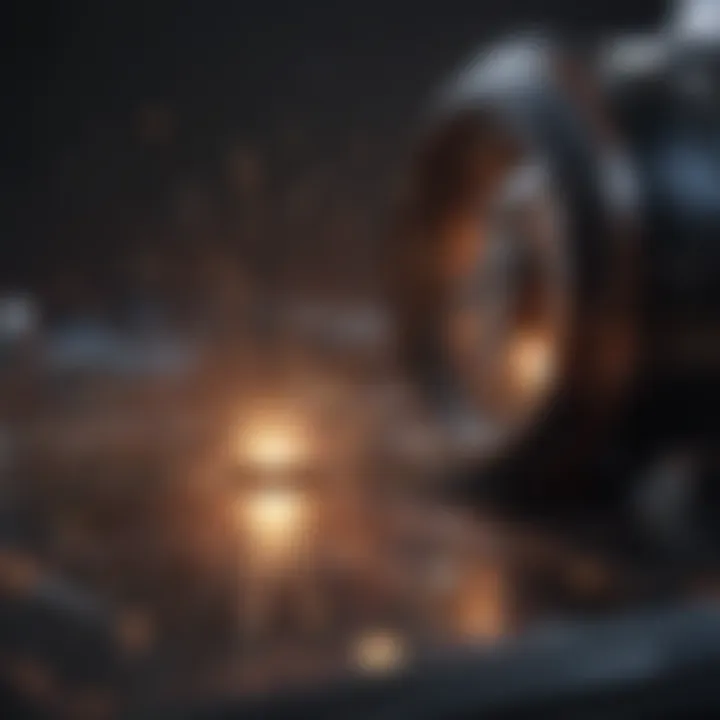
- Ease of Access: Researchers can easily find and download datasets.
- Interactivity: Researchers can exchange ideas and ask questions in real-time.
- Documentation: Properly maintained platforms provide documentation that ensures reproducibility of results.
However, developing these platforms does come with challenges. Considerations must include data security and user privacy, ensuring that sensitive data is not compromised. Additionally, the interface must be user-friendly to encourage greater participation from diverse fields.
Enhancing Data Analytical Tools
To effectively utilize CDW, enhancing data analytical tools is crucial. Current tools must be capable of handling large datasets efficiently. This often means incorporating robust algorithms and utilizing artificial intelligence to draw meaningful conclusions.
Modern analytical tools also benefit from:
- Increased Accuracy: Advanced tools reduce human error in data interpretation.
- Real-Time Analysis: Tools that allow for immediate analysis can provide insights faster.
- Integration Capabilities: The best tools can integrate with various data sources, enhancing versatility.
Some well-known tools in this space include R, Python, and Tableau. Each of these supports different aspects of data analysis, from statistical computations to visualizations.
"The true power of CDW lies in its ability to transform disparate data into cohesive insights that guide research decisions."
Future Prospects of CDW
The future of Collective Data Wisdom (CDW) is pivotal as it shapes how knowledge is formed and shared in scientific research. This section will delve into potential trends and innovations that could redefine collaborative efforts across various fields. These advancements may facilitate deeper connections among researchers and improve the overall quality of data interpretation and application.
Potential Trends in Research Collaboration
In the coming years, research collaboration is likely to evolve significantly. One considerable trend is the increasing integration of cloud-based platforms. These platforms allow researchers from differing geographic locations to share and analyze data effectively. It simplifies the process of collective data usage, making it accessible from various devices and environments. The use of application programming interfaces (APIs) to link various data sources and scientific tools will also improve the synergy of research efforts.
There is also a growing tendency toward interdisciplinary teams. By combining expertise from multiple fields, scientists can generate insights previously thought unattainable. For example, biologists could work alongside data scientists to leverage complex algorithms in biological research. Such collaborations may yield innovative solutions to challenges in fields like climate science or public health.
Moreover, the increasing emphasis on open science is shaping future collaborations. Open data initiatives can enhance transparency and inclusivity in research. As more datasets become publicly available, researchers will have more opportunities to contribute to collective data wisdom. This openness cultivates an environment of trust, encouraging a diverse range of contributors to share their insights and discoveries.
Innovation and Evolution of Data Science
Data science itself will continue to grow and evolve, influenced by advancements in technology and methodology. As machine learning and artificial intelligence technologies develop, researchers will increasingly rely on these tools to analyze massive datasets. The ability to harness machine learning will assist in identifying patterns and correlations that may not be immediately visible through traditional analysis techniques.
Furthermore, the continual improvement of data visualization tools will allow researchers to represent their findings more effectively. Clarity in presentation is key to understanding complex data sets. Enhanced visualization leads to improved decision-making and communication with broader audiences, including policymakers and the public.
Researchers should also prepare for the integration of ethical frameworks into data science practices. As data sharing becomes more common, it is crucial to address privacy and ethical issues surrounding data use. Developing guidelines that respect individual privacy rights while promoting collaboration will be essential.
"The evolution of data science and its innovative applications underscore the significance of collaborative efforts, making CDW a foundational aspect of modern research."
In summary, the future prospects of CDW promise to redefine how research is conducted. With growing trends in collaboration and innovations within data science, the scientific community stands on the brink of a new era—one characterized by enhanced connectivity and collaborative wisdom.
End
The conclusion serves as a vital component of this exploration into Collective Data Wisdom (CDW). It encapsulates the essential elements discussed throughout the article, emphasizing the significance of incorporating CDW into modern scientific research. By summarizing the insights gained, the conclusion reinforces how CDW influences data interpretation, innovation, and collaboration across disciplines. This final section also underscores the pressing need for scientists and researchers to embrace CDW actively.
Summarizing Key Insights
The implementation of Collective Data Wisdom fosters a collaborative environment where data can be shared and utilized effectively. Noteworthy benefits include the enhancement of data analysis through diverse perspectives, which leads to more robust findings. Moreover, CDW encourages interdisciplinary collaboration—bringing together different expertise and experiences to solve complex scientific problems.
Some key insights derived from this exploration include:
- Data Sharing: Efficient sharing of data enhances reproducibility and validity.
- Collaboration: Diverse teams produce innovative solutions that one discipline alone might not discover.
- Ethical Standards: Addressing ethical implications is crucial in fostering trust among researchers.
- Technological Integration: Utilizing advanced analytical tools improves data quality and reliability.
"Collective Data Wisdom will redefine how research is conducted, making collaboration an essential part of the process."
Call to Action for the Scientific Community
In summary, the call to action for the scientific community revolves around the proactive adoption and integration of CDW principles in research practices. Scientists, educators, and institutions should focus on creating more collaborative and transparent research environments. Initiatives could include:
- Establishing Collaborative Platforms: Using tools that promote data sharing and communication among researchers from various fields.
- Training Programs: Providing knowledge on data ethics, sharing protocols, and analytical tools to prepare the next generation of scientists.
- Engagement with Policy Makers: Advocate for policies that support open data initiatives, ensuring ethical standards are maintained.
The integration of CDW is not merely beneficial; it is necessary for advancing modern scientific research. Scientists must collectively harness this power. Together, they can overcome challenges, paving the way for future discoveries that are innovative, ethical, and impactful.