Comprehensive Overview of Omics Data Analysis
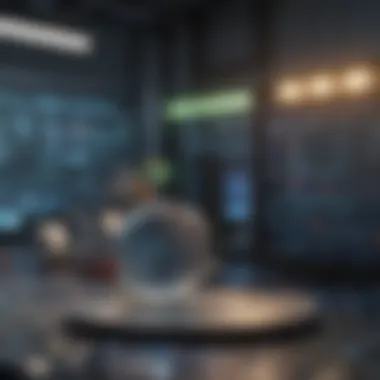
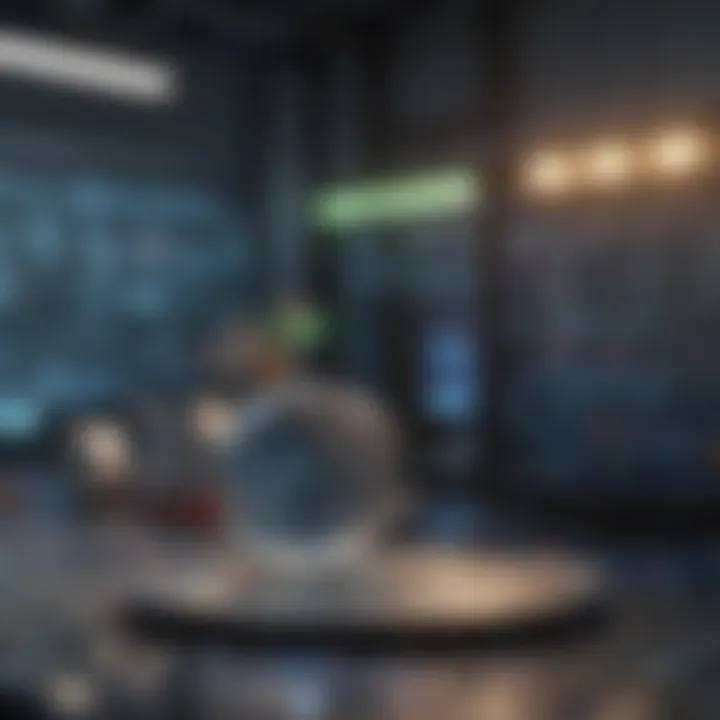
Intro
Omics data analysis stands as a pivotal component within the modern scientific landscape, particularly concerning biology, medicine, and environmental sciences. The sheer volume of data generated from various omics technologies—genomics, proteomics, metabolomics, and others—can feel overwhelming. Yet, understanding this data is where the magic lies. It transforms raw numbers into actionable insights that can revolutionize our understanding of complex biological systems.
As the field of omics continues to evolve, so does the necessity for a comprehensive course that encapsulates both essential techniques and practical applications. This article aims not just to introduce you to the multi-faceted world of omics data analysis, but also to equip you with the knowledge needed to dissect and interpret these complex datasets effectively.
"In a data-driven world, knowledge is power—learning how to analyze and interpret this information is a game changer."
Through this overview, we will explore the fundamental concepts, analytical methods, and tools that walk hand in hand with the ever-expanding realm of omic sciences. You'll find that with a solid foundation, including the latest trends, anyone can grasp the implications of omics data analysis across various research domains.
Preamble to Omics
The world of biology is rapidly evolving, and at the heart of this transformation lies omics – an umbrella term that encompasses various fields of study connected to a specific organism or biological system. Understanding omics is not merely an academic pursuit; it’s a crucial element for anyone wanting to grasp the complexities of biological systems, opening the door to discoveries that can lead to enhanced health, better crops, and environmental sustainability. In essence, omics serves as a bridge between raw data and meaningful insights, driving innovation across several scientific sectors.
Defining Omics and Its Importance
So what exactly is omics? It refers to the comprehensive study of molecules within biological samples. This can break down into several disciplines such as genomics, transcriptomics, proteomics, and metabolomics. Each of these subfields tackles a distinct layer of biological information, piecing together a puzzle that describes life in minute detail.
The importance of omics cannot be overstated. With advancements in sequencing technology and data analytics, researchers can collect and analyze vast amounts of data encompassing entire genomes and complex interactions within cells. This journey reveals not just the inner workings of organisms but could also lead to breakthroughs in personalized medicine, where treatments are tailored based on an individual's omics profile. The ramifications of omics data analysis are profound, providing insights that could reshape theories and practices in medical research, agricultural science, and environmental studies.
The benefits extend beyond healthcare. Consider agriculture; omics can be used to enhance crop yields and resiliency against diseases, which is crucial in a world grappling with food security. Likewise, in environmental contexts, omics can help in understanding biodiversity and its preservation. Clearly, omics is not just a buzzword but a crucial toolkit for addressing some of the most pressing challenges of our time.
Historical Context of Omics Research
To appreciate the significance of omics today, a glance at its historical context is invaluable. The journey began in the early 1990s, with the completion of the Human Genome Project. This landmark project aimed to map all the genes in the human body, laying a solid foundation for genomics as a discipline. Little did researchers know, this was just the tip of the iceberg.
Following the completion of the Human Genome Project, the field expanded rapidly. The advent of powerful sequencing technologies and analytic tools spurred interest in other areas of omics. For instance, proteomics emerged as scientists began to study proteins and their functions in greater detail. Before long, transcriptomics and metabolomics followed suit, leading to a blossoming of integrated approaches.
Today, omics research is interdisciplinary, involving bioinformatics, computational biology, and systems biology. The narrative has shifted towards understanding complex systems rather than isolated components. Researchers now strive for a holistic view, recognizing that biological processes are not merely linear but rather intricate webs of interaction.
This historical tapestry of omics research underscores its evolution from isolated studies into an interconnected web of scientific exploration, each discipline informing the other. With this rich background, it’s evident that delving into omics data analysis is imperative for anyone looking to contribute to the future of biological research.
Types of Omics Disciplines
Understanding the different types of omics disciplines is crucial for anyone pursuing a comprehensive grasp of omics data analysis. Each discipline, whether it be genomics, transcriptomics, proteomics, or metabolomics, unpacks a different layer of biological complexity. This specialized knowledge doesn’t just enhance our understanding of biological systems but also informs the development of targeted strategies in healthcare, agriculture, and environmental science.
Genomics: Exploring Genetic Information
Genomics, the first of our omics disciplines, dives into the DNA blueprint of organisms. There’s a good reason why it often takes the spotlight. With genomic sequencing technology becoming more affordable and accessible, researchers can now analyze vast amounts of genetic data more easily than before. This discipline allows for profound understanding about genetics and inheritance patterns, shedding light on diseases such as cancer, where mutations play a critical role.
Genomics is like cracking a code that has been written over billions of years; understanding it can lead to breakthroughs in personalized medicine and targeted therapies.
A few benefits of genomics include:
- Early Disease Detection: By analyzing genetic predispositions, potential health issues can be flagged early.
- Personalized Treatments: Tailoring medical treatments based on individual genetic makeups can lead to improved outcomes.
- Population Studies: Studying genetic variations across populations can help manage public health better.
Transcriptomics: Analyzing RNA Data
The next discipline is transcriptomics, which shifts the focus from DNA to RNA. While DNA holds the instructions, RNA reflects those instructions in action. By analyzing different RNA profiles, scientists can gauge gene expression levels, helping to identify how genes respond to various stimuli.
In transcriptomics, the science bridges the gap between genetic information and functional biology. It’s invaluable for understanding how genes are regulated during processes like development or disease progression.
Key components in transcriptomics include:
- Quantifying Gene Expression: Explore which genes are actively expressed under different conditions.
- Identifying Splicing Variants: Discover how genes can produce different proteins based on the splicing of RNA.
- Time-Lapse Studies: Understand gene dynamics over time, which is essential in developmental biology.
Proteomics: Understanding Proteins and Their Functions
Proteomics is all about proteins—those hard-working molecules that carry out most of the functions in our cells. Unlike genomics and transcriptomics, proteomics examines the proteome, which includes not just the types of proteins but also their structures, modifications, and interactions.
This discipline is particularly crucial because proteins are often the direct effectors of biological processes. Hence, analyzing proteins provides direct insights into cellular functions and mechanisms. There’s also growing interest in how proteomics intersects with therapy development, especially in understanding disease mechanisms.
Here are some points to consider in proteomics:
- Identifying Protein Interactions: Understanding how proteins communicate can unravel signaling pathways.
- Post-Translational Modifications: Studying how proteins are modified after they’re made sheds light on their functional versatility.
- Biomarker Discovery: Many diseases can be tracked by specific proteins found in bodily fluids, making proteomics crucial for diagnostics.
Metabolomics: Examining Metabolic Profiles
Finally, we arrive at metabolomics, which focuses on the small molecules that are the end products of cellular processes. It's like the icing on the cake—it provides immediate insights on the metabolic state of cells. By analyzing metabolites, scientists can gain a nuanced understanding of biological systems and how they change in response to environmental variations or disease states.
The applications of metabolomics are vast, ranging from clinical diagnostics to plant biology. Here are a few areas of focus:
- Metabolic Pathway Mapping: Helps in understanding disease mechanisms and developing treatment strategies.
- Nutritional Science: Explores how metabolites respond to dietary changes, thus impacting health.
- Toxicology: Monitors how toxins affect metabolic profiles, aiding in risk assessment for chemicals.
In summary, each omics discipline offers unique insights and tools for dissecting biological complexity. As technologies advance, the integration of these disciplines will play a central role in advancing our understanding of life's underlying processes.
Key Components of the Omics Data Analysis Course
Understanding the key components of an omics data analysis course is vital for anyone looking to excel in this rapidly advancing field. These components form the bedrock upon which participants can build their insights and hone their analytical skills. It’s about more than just numbers and data; it’s about putting this complex information into perspective.
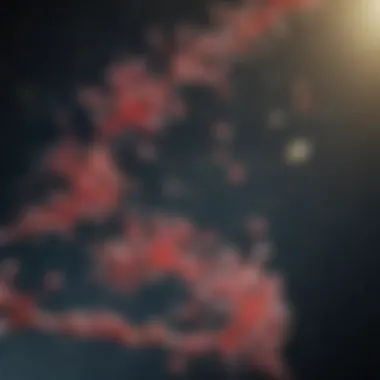
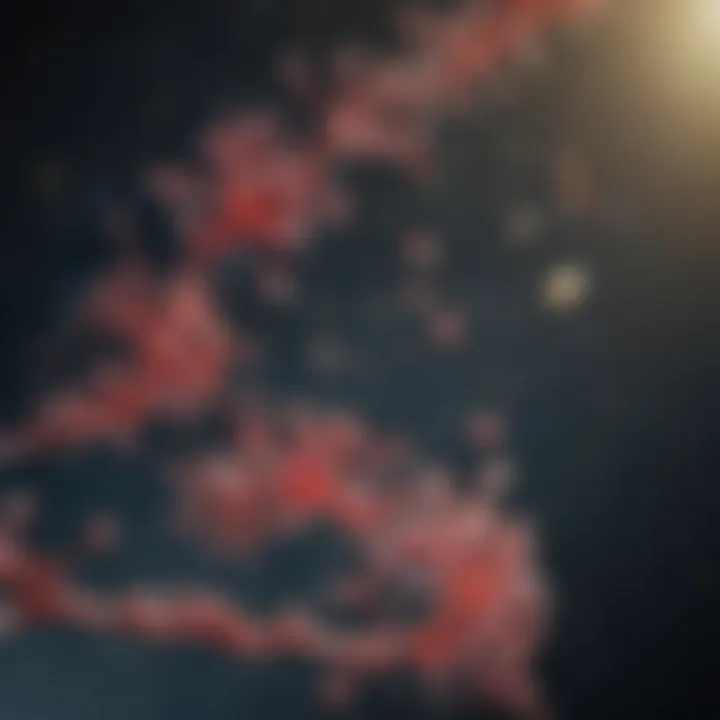
Course Objectives and Learning Outcomes
Every well-structured course needs a clear set of objectives and outcomes. Here, participants can expect to achieve several goals:
- Familiarity with Key Concepts: Grasping the foundational terms and methodologies surrounding omics.
- Hands-On Data Analysis: Engaging with real datasets, analyzing them, and deriving meaningful conclusions. This experiential learning solidifies theoretical knowledge.
- Application of Analytical Techniques: Understanding various statistical and machine learning approaches to process omics data.
- Integration Skills: Learning how to integrate data from different omics disciplines, which is increasingly crucial in multi-omics research.
These objectives ensure that participants not only learn but also apply their knowledge in practical scenarios. The ultimate outcome? Graduates walk away equipped with critical skills needed in today's data-driven biosciences environment.
Prerequisites for Participants
Before diving headfirst into an omics data analysis course, it’s important to lay down some prerequisites. Understanding these can help maintain the quality of discourse during the course. Participants generally should possess:
- Basic Knowledge of Biology: Familiarity with biological concepts, especially genetics, will enable comprehension of the omics landscape.
- Statistical Foundation: A handle on basic statistics is crucial. As the analysis heavily relies on statistical methods, this knowledge base helps in grasping complex concepts quickly.
- Programming Skills: Familiarity with coding, particularly in R or Python, becomes useful. This isn’t just about using software; it's about navigating scripts and understanding custom analyses.
- Curiosity and Engagement: Perhaps less tangible, but the drive to learn and engage actively is essential for a successful experience.
By keeping these prerequisites in mind, participants can maximize their learning experience. They are equipped to tackle the demanding nature of omics data analysis, ultimately enriching their journey through this fascinating domain.
Data Collection and Management Strategies
Data collection and management are cornerstone activities in the realm of omics data analysis. Understanding how to gather data effectively and manage it properly is essential for ensuring the integrity and usability of the information processed. Poor data management can lead to flawed conclusions, wasted resources, and ultimately, hinder progress in scientific discoveries. Therefore, having a solid grasp of data collection and organization strategies helps researchers and students maximize their analytical capabilities.
Ethical Considerations in Data Collection
Collecting omics data isn't just about generating numbers and sequences; it's about respect and responsibility towards the subjects involved. There are multiple ethical dimensions to consider, especially when working with human samples. For instance, obtaining informed consent is not just a formality—it's crucial. Participants need to understand how their genetic or biological materials will be used. Also, safeguarding personal data privacy is paramount. A breach in confidentiality can lead to significant consequences, not just for the individuals involved but also for the credibility of the research.
Here's a rundown of significant ethical factors in omics data collection:
- Informed Consent: Ensure that participants are clearly informed about the study, risks, and data usage.
- Confidentiality: Protect identities and personal data, making sure only authorized personnel have access to sensitive information.
- Bias and Representation: Be vigilant about inclusivity—research should represent diverse populations to avoid biased outcomes.
- Data Misuse: Proactively manage the risks of data being misappropriated in ways that could harm participants or communities.
The ethical dimension should be woven into the very fabric of data collection practices. Establishing trust not only enhances participant engagement but also solidifies the integrity of the research itself.
Best Practices for Data Storage and Organization
Once data is collected, the next challenge is ensuring it is stored and organized effectively. A systematic approach to data management can save time and resources while minimizing errors. Here are some practices to consider:
- Standardized Formats: Adopting uniform naming conventions and data formats simplifies the analysis process. For example, dates should follow the same structure regardless of the dataset.
- Metadata Documentation: Describing data with relevant metadata can clarify data context. It may include information on how it was collected, processed, and its relevance to specific hypotheses.
- Version Control: Utilize version control systems, particularly in collaborative environments, to track changes and maintain data integrity.
- Secure Backups: Regularly back up data in multiple locations to prevent loss. Utilizing cloud services can greatly enhance secure storage practices.
"Proper data organization is like a well-organized toolbox. When you need a specific tool, you don’t want to fumble about looking for it. You want to know exactly where it is."
Following these practices establishes a reliable framework for data management, crucial for robust analysis. With the right strategies in place, researchers can focus their efforts on extracting insights rather than battling with chaotic data sets.
Analytical Techniques in Omics Data Analysis
In the realm of omics data analysis, the ability to apply precise analytical techniques is paramount. These techniques serve as the backbone of understanding complex biological data, allowing researchers to uncover patterns, make predictions, and derive actionable insights. It’s a vast field where attention to detail can make or break the findings. Therefore, exploring and mastering these methodologies enhances the robustness of any research.
Statistical Approaches: Fundamentals and Applications
Statistical approaches lay the groundwork for omics data analysis by offering methods to interpret biological signals amidst noise. In a nutshell, they help researchers to discern meaningful variances within large datasets. Think of it as trying to find a needle in a haystack, where statistical tools are the magnets that attract significant information while pushing aside the irrelevant debris.
Here are some key statistical techniques commonly employed in omics:
- Descriptive Statistics: This helps summarize the data by using metrics like mean, median, and standard deviation. It’s fundamental in providing a snapshot of the datasets.
- Inferential Statistics: This involves hypothesis testing to draw conclusions about the population from which the sampled data is drawn. Methods like t-tests and ANOVA are commonly used here.
- Multivariate Analysis: Techniques such as Principal Component Analysis (PCA) allow the examination of multiple variables simultaneously, revealing intricate relationships within the data.
By employing these statistical methods, researchers can address various biological questions. For example, a study might utilize ANOVA to compare gene expression between different treatment groups, providing insights into the effectiveness of a drug.
As a deeper understanding emerges, particularly the importance of reproducibility and validity, researchers must be wary of pitfalls. Statistical methods must be rightly chosen and correctly applied; an inappropriate technique can lead to misleading conclusions. As the saying goes, "Garbage in, garbage out." In this case, accurate input and meticulous analysis are essential to ensure credible results.
Machine Learning Algorithms in Omics
With the explosion of data, machine learning algorithms have become vital in analyzing omics datasets. These algorithms excel at handling large volumes of data and identifying patterns that traditional methods might overlook. Think about it; with machine learning, researchers can sift through mountains of molecular data faster than you can say "bioinformatics."
Some key algorithms frequently applied in omics analysis include:
- Support Vector Machines (SVM): Useful for classification tasks, SVM can help distinguish between different expressions of genes related to disease.
- Random Forests: This ensemble method improves predictive accuracy. It's particularly beneficial for understanding complex interactions among many biological variables.
- Neural Networks: Particularly beneficial in modeling nonlinear relationships, neural networks can dive deeper into associations between genetic variations and phenotypic outcomes.
Furthermore, machine learning brings a certain agility to the process. Models can be iteratively refined as new data comes in, allowing researchers to adapt and enhance their analysis continuously.
"Machine learning isn’t just a trend; it’s the lifeblood of innovation in omics analysis."
The combination of machine learning with traditional statistical methods creates a more comprehensive framework for analysis. Researchers now have tools that not only analyze data but also learn and evolve with it. It’s crucial to realize, though, that these models require careful tuning and validation to avoid biases and ensure reliability in predictions.
Software Tools for Data Analysis
In the realm of omics data analysis, software tools serve as the backbone, streamlining complex processes and enhancing the ability to extract meaningful insights from vast datasets. The importance of these tools cannot be overstated, as they not only facilitate data management but also support the application of advanced statistical and machine learning techniques. Having the right software applications at your fingertips can mean the difference between comprehending a massive genome dataset or getting lost in a sea of numbers. This section will delve into popular omics analysis software, evaluating their contributions and functionalities.
Overview of Popular Omics Analysis Software
When it comes to analyzing omics data, a variety of software tools cater to different needs and preferences. Some of the well-known applications include:
- R and Bioconductor: A powerful ecosystem for data analysis and visualization, especially in genomics.
- Python Libraries: Such as NumPy and Pandas, offering flexibility, customizable data manipulation, and analysis.
- Dedicated Omics Platforms: There are various platforms that integrate multiple omics disciplines to provide comprehensive analysis capabilities.
These tools cater to users ranging from beginners to seasoned professionals, ensuring a suitable option tailored for diverse applications in omics research.
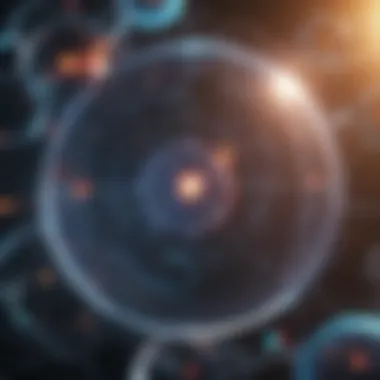
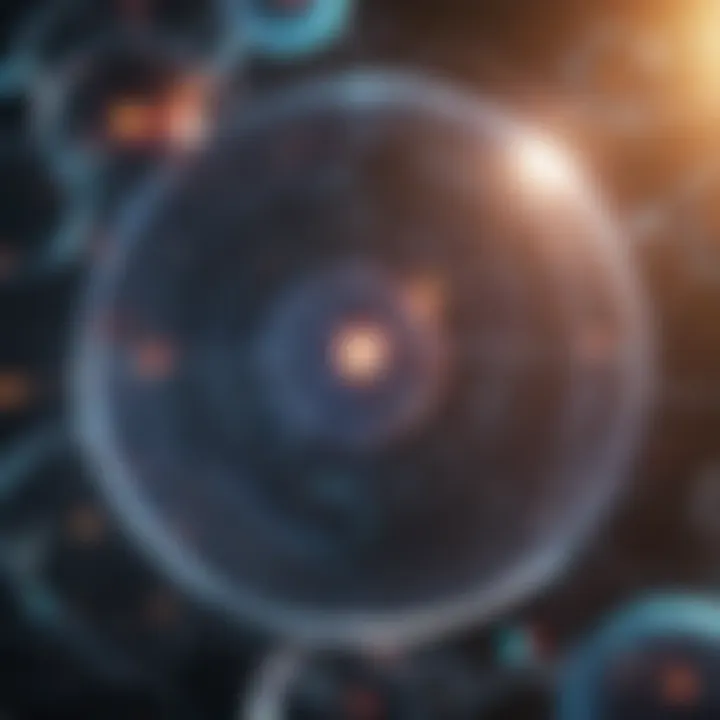
Comparison of Software Platforms
Comparing software platforms is crucial for selecting the ideal tool that aligns with specific research goals. Each platform has its strengths and weaknesses, impacting ease of use, analysis capabilities, and accessibility.
R and Bioconductor
R is a programming language widely recognized for its statistical prowess. Bioconductor is a set of packages built on R that specializes in bioinformatics and computational biology. It offers:
- Key Characteristic: An extensive collection of tools tailored for analyzing genomic data, which makes it a go-to for researchers.
- Unique Feature: The capacity for high-level data visualization. This is beneficial for uncovering patterns that might otherwise remain obscured in raw data.
Advantages:
- Comprehensive tools for rigorous statistical tests.
- Strong community support and documentation.
Disadvantages:
- Steep learning curve for those unfamiliar with programming and R syntax.
Python Libraries for Omics Analysis
Another mainstay in data analysis is Python, known for its simplicity and versatility. Its libraries, like SciPy and scikit-learn, specialize in scientific computation and machine learning:
- Key Characteristic: User-friendly interface that allows for a more straightforward programming experience.
- Unique Feature: Integration of machine learning algorithms within data analysis tasks, allowing for advanced predictive modeling.
Advantages:
- Intuitive syntax caters to those new to programming.
- Strong capabilities for machine learning applications.
Disadvantages:
- May lack certain specialized functions compared to R's Bioconductor.
Dedicated Omics Platforms
Dedicated omics platforms are constructed to facilitate a thorough analysis across the different omics disciplines. These platforms offer a one-stop shop for researchers looking to manage, analyze, and interpret multi-omic data:
- Key Characteristic: They typically provide a user-friendly interface, making it easy to navigate through complex datasets without needing extensive coding skills.
- Unique Feature: Cross-disciplinary integration, which lets researchers utilize data from multiple sources (genomic, proteomic, etc.) in a cohesive analysis framework.
Advantages:
- Highly specialized tools designed specifically for omics data.
- Often come with built-in visualization capabilities.
Disadvantages:
- May be expensive and require subscriptions.
- Limited flexibility compared to programming-based solutions like R and Python.
Overall, the choice of software can significantly influence the analytical approach and ease with which insights can be gleaned from omics data. Each tool brings its own set of strengths, catering to various aspects of data analysis and interpretation.
Applications of Omics Data Analysis
The applications of omics data analysis extend far and wide, carrying significant implications across multiple fields such as medicine, agriculture, and environmental science. Through scientific investigation of biological data, researchers can unlock new potentials and innovations that were previously thought unattainable. This approach not only enhances our comprehension of complex biological systems but also harmonizes empirical research with practical applications.
Clinical Applications and Personalized Medicine
In the realm of healthcare, omics data analysis is transformative, especially in its role in personalized medicine. This field drives the shift from a one-size-fits-all approach to individualized treatment plans. By analyzing genomic and proteomic data, clinicians can tailor therapies based on a patient's unique genetic profile, leading to more effective treatment strategies.
For instance, cancer treatments have seen revolutionary changes. Specific genetic mutations in tumors can be precisely matched with targeted therapies. For example, drugs like trastuzumab are designed for patients with HER2-positive breast cancer. Moreover, understanding a patient's metabolome can predict their response to medications, minimizing adverse effects and enhancing treatment efficacy.
"Personalized medicine is not just about treating the disease; it’s about treating the individual who has the disease."
This tailored approach also fosters earlier diagnoses, which can be crucial for conditions like cardiovascular diseases. Identifying biomarkers through lipidomics can facilitate timely and preventive interventions. As research progresses, integrating proteomics and transcriptomics further enriches our understanding, leading towards a truly holistic healthcare landscape.
Agricultural Biotechnology: Enhancing Crop Yield
The implications of omics data analysis stretch beyond medicine, profoundly impacting agricultural biotechnology. As the global population soars, the need for sustainable agricultural practices is paramount. By leveraging genomics and metabolomics, researchers are discovering ways to enhance crop yield resilience against environmental stresses, pests, and diseases.
By employing these sophisticated techniques, scientists can identify favorable traits in plants. For example, understanding the genetic basis of drought resistance can guide crop breeders to develop varieties that thrive in changing climates. Moreover, innovations in transcriptomics allow researchers to analyze gene expression in real-time, optimizing growth and improving nutrient profiles in crops.
As a result, farmers gain access to crops that are not only more productive but also healthier, which is essential for food security. This holistic view of agricultural practices, integrating biological data, moves us closer to innovative farming solutions.
Environmental Studies: Assessing Biodiversity
Omics data analysis serves as a powerful tool in environmental studies, particularly in assessing and preserving biodiversity. Understanding the complex interactions within ecosystems is critical for conservation efforts. By utilizing metagenomics, researchers can investigate the genetic material present within environmental samples, providing insights into microbial diversity and ecosystem functionality.
For instance, in studies focusing on coral reef ecosystems, scientists have utilized metagenomic approaches to assess the health of coral populations. Identifying the genetic diversity of microbes associated with coral can reveal vital information about the resilience of these ecosystems to climate change and pollution. As such, comprehensive omics analyses drive informed decisions in conservation strategies, promoting an intricate understanding of ecological dynamics.
In summary, the multidisciplinary applications of omics data analysis underscore its significance in contemporary research. It bridges the divide between data and actionable insights, thereby shaping the future of medicine, agriculture, and environmental conservation. By enabling tailored strategies and fostering sustainable practices, the potential of omics data stands to address pivotal challenges facing our planet today.
Emerging Trends in Omics Research
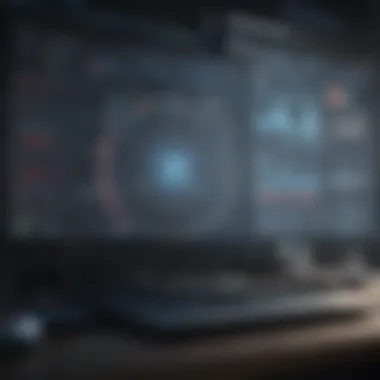
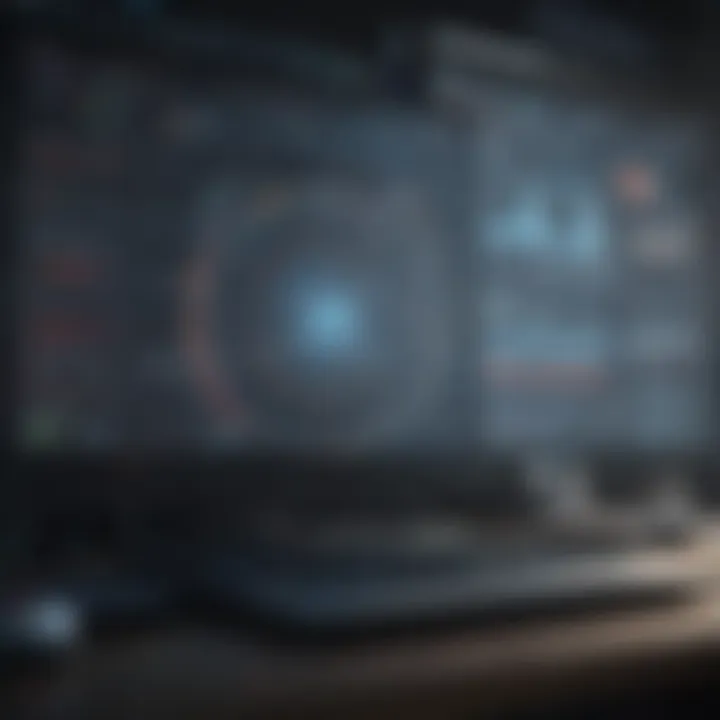
The field of omics research is constantly evolving, ushering in fresh techniques and methodologies that hold great promise for scientific inquiry. As we stand on the precipice of new breakthroughs, it becomes imperative to explore these emerging trends thoroughly. Such an examination is not only beneficial for enhancing our understanding of biological systems but also critical for pushing the boundaries of research applications in areas such as personalized medicine, agricultural innovations, and environmental sustainability.
Integration of Multi-Omics Data
One of the most significant shifts in omics research is the integrated approach. Multi-omics involves combining various omics disciplines—such as genomics, proteomics, and metabolomics—into a single analysis framework. This holistic method can provide deeper insights into biological phenomena than analyzing each layer of data in isolation.
The significance of integrating datasets becomes apparent when you consider the complexity of biological systems. Individual omics approaches can reveal certain aspects, but when woven together, they can give a more complete picture. For instance:
- Interconnected Insights: Genomic variants can influence protein expression, which in turn affects metabolic pathways. Multi-omics analysis captures these connections effectively.
- Enhanced Predictive Models: Integrated data can improve the accuracy of predictive models, especially in personalized medicine. By understanding the interplay between different biological components, researchers can make better-informed decisions about treatment options.
- Comprehensive Biomarker Discovery: The collaboration of different omics data assists in uncovering novel biomarkers, facilitating early diagnosis and targeted therapies.
However, challenges persist. The collection and analysis of multi-layered data necessitate advanced computational methods and algorithms, as well as robust frameworks for data management. The integration of diverse datasets poses substantial technical hurdles and ethical issues regarding data sharing and privacy that researchers must navigate cautiously.
Advancements in Single-Cell Omics
Parallel to the move towards multi-omics, the field of single-cell omics is also experiencing rapid advancements. This approach focuses on the analysis of individual cells rather than bulk populations, allowing for an unprecedented level of detail in biological research. By examining the heterogeneity within tissues, researchers can glean insights that would otherwise remain obscured.
There are several noteworthy advancements reshaping single-cell omics:
- Improved Sequencing Technologies: Newer sequencing techniques are allowing for higher throughput and lower costs, making single-cell RNA sequencing more accessible.
- Spatial Transcriptomics: This innovative technology captures the spatial context of cells, providing information about cellular interactions and microenvironments. Understanding these relationships plays a vital role in developmental biology and cancer research.
- Enhanced Data Analysis Tools: The development of sophisticated software tools is enabling researchers to analyze single-cell data more effectively. These tools help in visualizing complex data sets, allowing for nuanced interpretations of cellular behaviors.
As single-cell omics continues to gain traction, researchers are faced with challenges surrounding data complexity and the need for refined analytical methods. The intricate nature of single-cell data demands not just robust computational resources but also interdisciplinary collaboration to translate findings into actionable scientific breakthroughs.
The future of omics research lies in its ability to evolve and adapt. By embracing integrated and single-cell approaches, we can significantly enhance our understanding of life's complexities.
In summary, the trends of multi-omics integration and single-cell analysis represent pivotal shifts in the realm of omics research. These methodologies provide new avenues to explore and understand biological complexities, which can ultimately lead to impactful advancements in various scientific disciplines.
Future Directions of Omics Data Analysis
In the ever-evolving landscape of scientific research, the future directions of omics data analysis are a Pandora’s box of possibilities awaiting exploration. As the field matures, various new strategies and technologies are emerging, driving both efficiency and effectiveness in data interpretation. These advancements not only promise to enhance our understanding of biological systems but also point towards new applications in medicine, agriculture, and environmental science. Addressing how these changes will unfold is crucial not only for researchers already immersed in this domain but also for students and professionals who aim to navigate the future of omics analysis adeptly.
The Role of Artificial Intelligence
Artificial Intelligence (AI) is quickly becoming the backbone of omics data analysis. Its ability to process vast amounts of data at lightning speed is nothing short of revolutionary. Machine learning algorithms can sift through complex datasets, revealing patterns that may otherwise remain hidden to the human eye. This can be especially useful in genomics, where AI can help in predicting gene functions and interactions with remarkable precision.
- Benefits of AI in Omics:
- Increased Accuracy: AI models can enhance predictive accuracy in genetic data interpretation.
- Simplifying Complexity: They simplify complex data mixtures into more understandable insights.
- Automated Analysis: Robust algorithms can automate repetitive tasks, freeing researchers to focus on hypothesis generation and testing.
However, it is not just about plopping algorithms into place. Careful consideration must be given to the data they train on. Garbage in, garbage out, as they say. Moreover, collaborators in various disciplines, from bioinformatics to clinical research, must work in sync to ensure that AI tools evolve in tandem with biological insights and ethical considerations.
Collaborative Research Opportunities
The essence of advancing omics data analysis lies in the spirit of collaboration. The future points strongly to multidisciplinary partnerships that unite genetics, data science, agriculture, and environmental studies. Such collaborations open doors to innovative methodologies and insights that single-discipline teams may overlook.
- Key Collaboration Areas:
- Health and Medicine: Joining forces with clinical researchers can drive the creation of personalized medicine.
- Agricultural Research: Agricultural scientists can work with omics experts to boost crop resistance and yield, applying insights gleaned from sequencing data.
- Environmental Studies: Collaborating with ecologists enables a more profound grasp of biodiversity and ecosystem health using omics approaches.
It is often said that teamwork makes the dream work. In the case of omics data analysis, collaborative research is not just beneficial; it may be essential for future breakthroughs.
Epilogue
As we draw the curtains on this extensive exploration of omics data analysis, it’s vital to grasp the substantial role that this field plays in contemporary research and its future potential. The conclusion doesn’t just serve as a wrap-up, rather it synthesizes the insights gathered from the various sections discussed throughout the article. The essence of omics data analysis lies in its multifaceted nature—enabling scientists to delve deep into biological questions, harnessing data from genomics, transcriptomics, proteomics, and metabolomics, and orchestrating these streams into insightful narratives.
Summary of Key Points
In revisiting the core themes, several pivotal points emerge:
- The Significance of Omics: Omics analysis unfolds a panorama of biological intricacies, allowing researchers to decipher aspects like gene expression, metabolic pathways, and protein interactions.
- Technological Integration: Advanced software tools facilitate robust data analysis, making the manipulation of vast datasets manageable and insightful.
- Real-World Applications: From clinical innovations to agricultural advancements, the applications of omics are far-reaching, providing tangible benefits that extend to personalized medicine and sustainability efforts.
- Emerging Trends: The recent trends in integrating multi-omics and advances in single-cell techniques underscore the dynamic nature of the field, paving the way for novel insights in various domains.
The understanding of these elements lays the groundwork for effective engagement with omics data, fostering a comprehensive grasp that goes beyond rote memorization.
Implications for Future Studies
Looking ahead, the implications for future studies in omics data analysis are profound. The growing intersection of artificial intelligence with omics is particularly noteworthy. The incorporation of machine learning can automate the preprocessing and analysis of data, opening doors to discoveries that were previously deemed too complex to tackle. Collaborations spanning across disciplines are set to flourish, as biologists and data scientists unite to expand the horizons of what can be achieved.
Key considerations for ongoing studies might include:
- Embracing Cross-disciplinary Collaboration: Expanding partnerships that bridge biology, mathematics, and computer science will enhance the depth and breadth of analyses.
- Investigating Ethical Implications: As data handling becomes more complex, addressing privacy and ethical concerns will be crucial to preserve scientific integrity.
- Promoting Data Transparency: An emphasis on open data formats and sharing will facilitate broader engagement within the scientific community, enhancing reproducibility and trust in findings.
By keeping these aspects in mind, the future of omics data analysis promises not just advancements in knowledge, but also solutions to the pressing challenges facing society today.
Curated List of Key Literature
The curated list of key literature is a treasure trove for anyone keen on delving deeper into omics data analysis. Specifically, these resources often encompass landmark studies that have defined the discipline as well as emerging research that pushes boundaries. Below is a sample compilation that can guide one’s exploratory path:
- Nature Reviews Genetics: Provides comprehensive reviews on genetic research and advancements.
- Bioinformatics journal: Focuses on the application of statistics and information technology in biology.
- PLOS ONE: An inclusive journal publishing a platform for rigorous research across all fields of science.
- Trends in Biotechnology: Offers insights on how omics technologies are being adapted in various fields such as agriculture and medicine.
- Journal of Proteome Research: Specializes in the exploration of protein profiles and functions.
These publications serve as a launch pad for deeper inquiry. Researchers and students are encouraged to engage critically with the material, dissecting methodologies and analyzing results while considering the implications of each study.
Online Resources and Databases
The online landscape provides a vast array of databases and resources that complement traditional literature. They function as practical tools for data retrieval, analysis, and interpretation, which is critical in omics studies. A few notable online resources include:
- NCBI (National Center for Biotechnology Information): A key repository of biomedical and genomic information.
- EMBL-EBI (European Molecular Biology Laboratory - European Bioinformatics Institute): Offers access to databases and services tailored for biological research.
- Ensembl: A genome browser that provides annotations for various species.
- The Gene Expression Omnibus (GEO): An archive of high-throughput gene expression data and other functional genomics datasets.
- ArrayExpress: A database of functional genomics experiments, providing an easy way to access and analyze datasets.
Having these resources at one's disposal enhances the efficiency of the research process. They not only enable the collection and organization of large datasets but also facilitate collaboration across multiple disciplines. As the field of omics continues to evolve, staying connected with online resources ensures that one's insights and methodologies remain current and applicable.