CRISPR Off-Target Prediction Tools for Gene Editing
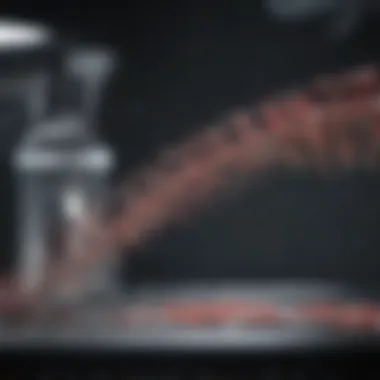
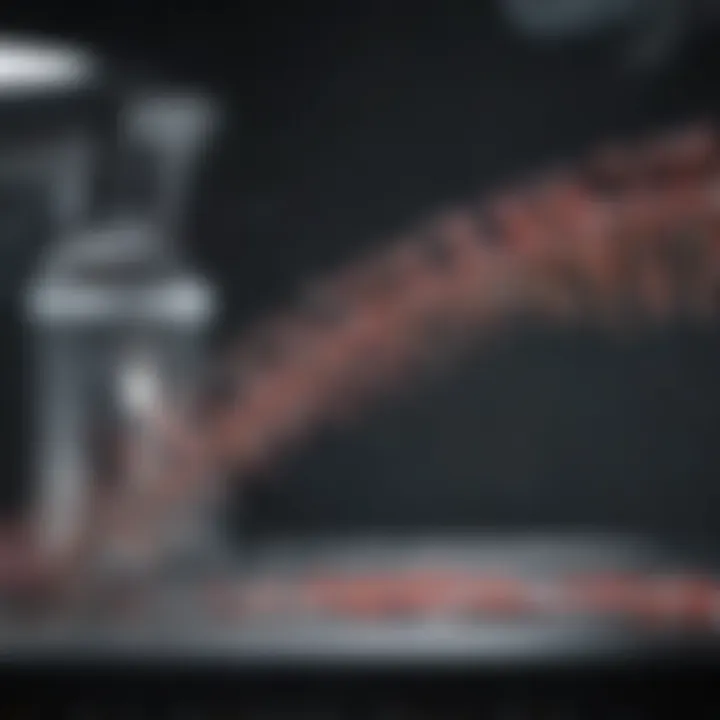
Intro
The emergence of CRISPR technology has marked a pivotal shift in genetic engineering. This innovative tool allows for precise modifications to DNA, enabling researchers to explore genetic functions and develop potential therapies for various diseases. However, as with any powerful tool, the potential for unintended consequences exists. Off-target effects, where CRISPR inadvertently alters unintended locations in the genome, pose significant risks. Thus, the development of off-target prediction tools is crucial in ensuring the precision of gene editing.
Understanding how these tools function illuminates the intricacies of gene editing and establishes a foundation for safer and more reliable applications. The scope of CRISPR off-target prediction extends from academic research to clinical therapies, highlighting the importance for researchers and practitioners alike.
Intro to CRISPR Technology
CRISPR technology has emerged as a groundbreaking advancement in the field of genetics. Its importance cannot be overstated, as it has transformed the landscape of gene editing. Understanding CRISPR is crucial for researchers and practitioners involved in genetic engineering. The specific elements of CRISPR technology, its benefits, and relevant considerations shape the foundation on which gene editing operates.
Gene editing has a rich history that led to the development of CRISPR. With traditional methods often being tedious and imprecise, there was a clear need for innovation. CRISPR stands out not only for its effectiveness but also for its relative simplicity compared to earlier technologies like zinc-finger nucleases and transcription activator-like effector nucleases (TALENs). This advancement marks a notable shift towards more precise and targeted genetic modifications.
Historical Context of Gene Editing
The journey of gene editing dates back several decades. Early attempts at altering genetic material involved rudimentary methods that lacked precision. However, the introduction of techniques like recombinant DNA technology set the stage for significant breakthroughs. This paved the way for the development of CRISPR in the early 21st century, inspired by the natural defense mechanisms in bacteria. Understanding this historical context helps to appreciate the problem-solving nature of scientific progress.
CRISPR, short for Clustered Regularly Interspaced Short Palindromic Repeats, was first characterized in 1987 as a component of bacterial immune systems. Researchers uncovered its potential for gene editing in the 2000s, especially after the discovery of the Cas9 enzyme, which can cut DNA at targeted locations. This mechanism revolutionized genetic research and applications.
Understanding CRISPR Mechanism
At its core, CRISPR technology operates through a straightforward yet highly effective mechanism. It utilizes RNA molecules to guide the Cas9 enzyme to specific DNA sequences for modification. The process begins with the design of a guide RNA that matches the target DNA sequence. This specificity is what grants CRISPR its remarkable precision compared to previous gene editing techniques.
When the guide RNA binds to the target DNA, the Cas9 enzyme introduces a cut in the DNA strand. This targeted cleavage can result in either disabling a gene or introducing new genetic material. This dual capability is a significant aspect of CRISPR's appeal in research and therapeutic applications.
In summary, CRISPR technology represents a substantial evolution in gene editing capabilities. Its historical development and operational mechanisms are fundamental to comprehend the technology's implications and applications. As the field continues to evolve, the understanding of these elements provides a solid groundwork for discussions about off-target prediction tools and the precision they aim to ensure.
Significance of Off-Target Effects
Understanding off-target effects is essential in the landscape of CRISPR technology. These unintended alterations can have profound implications for both basic research and therapeutic applications. Recognizing how these effects influence gene editing outcomes is pivotal for advancing the field.
Defining Off-Target Effects
Off-target effects refer to unintended modifications made by CRISPR systems in genes other than the intended target. Often, this occurs due to sequence similarities between the target DNA and other regions within the genome. Such effects can compromise the safety and efficacy of genetic interventions. This is particularly concerning in therapeutic contexts, where precision is not just desired but required. Scientists must account for these possibilities to ensure that the desired genetic modification achieves its purpose without causing additional, possibly harmful, changes elsewhere in the genome.
Impact on Gene Editing Outcomes
The impact of off-target effects on gene editing can be quite significant. These alterations can lead to unexpected phenotypic outcomes, which may vary from benign to severely detrimental. In research, this variability can obscure results or mislead conclusions about gene function. In therapeutic settings, the consequences can be more severe. If off-target effects occur in critical genes, they can cause diseases or disrupt normal biological functions. Thus, prediction tools become crucial in identifying potential off-target sites before experimental applications.
"Addressing off-target effects is not simply an academic exercise; it is a necessity for the responsible application of gene editing technologies."
In addition, understanding off-target effects allows for the refinement of CRISPR techniques. Identifying and mitigating these effects can enhance the overall precision and reliability of gene editing. Improved methods can lead to higher success rates in therapeutic applications and pave the way for more robust genetic research, potentially unlocking new avenues for treatment across various diseases.
Overview of Off-Target Prediction Tools
Off-target prediction tools play a crucial role in the landscape of CRISPR technology. As gene editing progresses, understanding how to minimize off-target effects is essential. These tools assist researchers in predicting where unintended edits may occur in the genome. The implications of accurate prediction are significant. They can enhance safety and efficacy in both research and therapeutic applications. Therefore, the overview of such tools is not just informative but necessary for responsible gene editing.
Types of Prediction Tools
There are several types of prediction tools available, each offering unique methodologies and applications. They vary in complexity and the specific algorithms used. Common categories include:
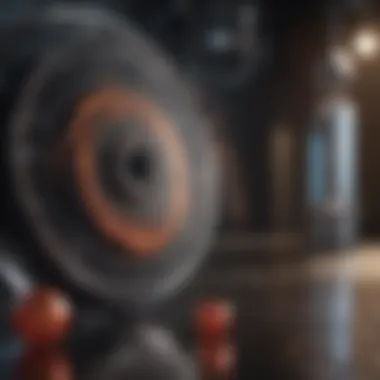
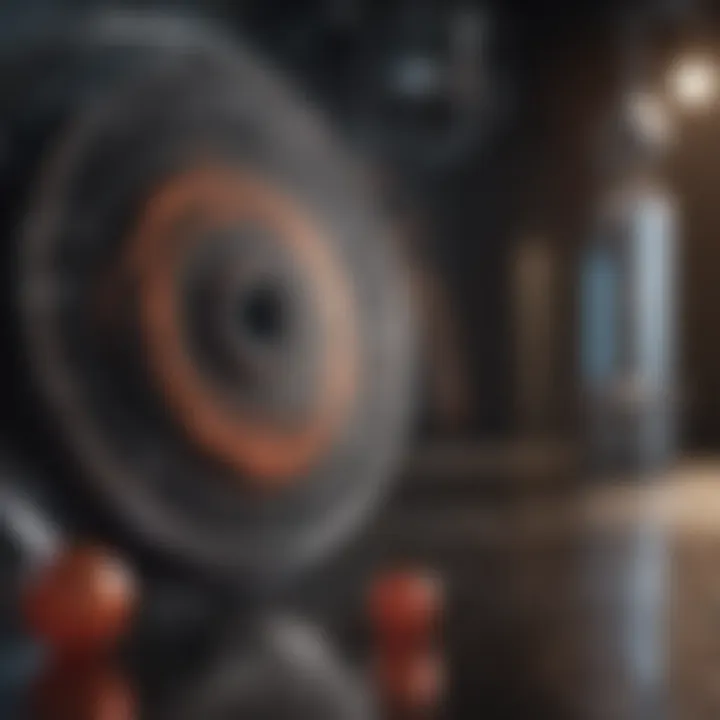
- Sequence-based tools: These consider the genomic sequence of interests. They evaluate the potential binding sites of the CRISPR guide RNA and predict off-target locations based on sequence similarity.
- Structure-based tools: These focus on the three-dimensional structure of the CRISPR-Cas complex. They analyze interactions at the molecular level, providing insights into how changes in structure may influence binding.
Some well-known tools include CRISPRoff, Cas-OFFinder, Off-Spotter, and CCTop. Each tool employs different approaches, which can lead to varying levels of accuracy. Understanding these types helps researchers choose the most suitable tool for their needs.
Key Features to Consider
When selecting an off-target prediction tool, several important features warrant consideration:
- Accuracy and sensitivity: A tool should strike a balance between predicting actual off-target effects while minimizing false positives. Higher accuracy leads to better-informed decision-making in experimental design.
- User-friendliness: Some tools require complex computations, making them less accessible for less experienced users. A simple and intuitive interface can significantly impact usability.
- Database integration: Tools that access extensive genomic databases increase their predictive capabilities. A comprehensive database provides context and enhances the chance to identify off-target sites effectively.
- Output formats: The way results are presented matters. Clear and organized outputs facilitate better interpretation of the data.
"The choice of a prediction tool can influence the success and safety of CRISPR applications greatly."
Incorporating these considerations when using off-target prediction tools ensures more reliable results. A thorough understanding leads to better implementation of gene-editing strategies, ultimately improving the outcomes of research and therapeutic interventions.
Popular CRISPR Off-Target Prediction Tools
The importance of off-target prediction tools cannot be overstated in the context of CRISPR technology. These tools provide researchers with the means to assess potential unintended effects when editing genes. The primary goal is to improve precision in gene editing, reducing the chances of unintended modifications that can lead to unforeseen consequences in biological systems. Identifying off-target sites ensures that interventions remain effective and safe, especially in clinical applications.
As researchers continue to explore the potential of CRISPR, understanding the most popular off-target prediction tools is essential. Each tool comes with unique features that cater to different needs, offering various benefits and considerations. Here are some of the most commonly used CRISPR off-target prediction tools:
CRISPRoff
CRISPRoff is a significant player in the landscape of off-target prediction tools. This tool is designed to provide detailed information about potential off-target effects by analyzing sequence similarities. Its ability to predict off-targets based on genomic context adds depth to the analysis, making it particularly useful for researchers focused on detailed genotyping. The interface is fairly user-friendly, which facilitates ease of use, even for those who might not be bioinformatics specialists.
One of its standout features is the incorporation of a database of known off-target sites, allowing users to quickly compare against previously documented effects. This feature helps streamline the validation process, saving time and resources for researchers. However, its predictive accuracy can vary based on the target and the complexity of the genomic environment.
Cas-OFFinder
Cas-OFFinder is another robust tool that researchers frequently utilize. It operates by analyzing sequences to identify potential off-target sites based on the sequence of the gRNA. The tool is known for its speed and efficiency, providing quick results, which is advantageous when working in time-sensitive projects.
Key functionalities include the ability to customize search parameters and the option to incorporate mismatches in the gRNA sequence. This allows researchers to model various scenarios that might arise during the gene-editing process. Cas-OFFinder's accuracy is well-regarded, though users should remain aware that predictions may not always align perfectly with empirical validation. Accurate understanding of its limitations is crucial when interpreting its results.
Off-Spotter
Off-Spotter is a lesser-known but effective prediction tool that approaches off-target analysis differently. It employs a combinatorial algorithm to evaluate potential off-target sites by analyzing the gRNA sequence and target DNA. The tool emphasizes precision and is tailored for researchers who seek an in-depth analysis of their CRISPR designs.
One of the notable advantages of Off-Spotter is its flexible design, allowing users to input varying conditions for their CRISPR setups. This can be particularly beneficial in complex experimental designs. However, this flexibility might come with a steeper learning curve, which can deter some users. Evaluating its usability in practical applications is necessary for optimizing its integration into gene editing workflows.
CCTop
CCTop, or the CRISPR-Cas9 Target online predictor, stands out for its comprehensive features aimed at providing a holistic analysis of CRISPR efficacy. It allows for the assessment of both on-target and off-target activity, giving researchers a broader view of potential impacts.
The tool includes functionality for assessing multiple target sites, making it useful for projects involving multiple edits. By integrating off-target prediction with a variety of available experimental data, CCTop allows researchers to refine their strategies effectively. While it offers advanced features, it may require more experience to navigate effectively compared to simpler prediction tools. This balance between functionality and usability is a critical factor to consider when selecting the right tool for specific research needs.
Methodologies in Off-Target Prediction
Understanding the methodologies in off-target prediction is vital for advancing CRISPR technology. These methodologies integrate computational and experimental approaches. Enhancing the precision of gene editing techniques necessitates an organized framework to evaluate off-target effects. This section will delve into both computational and experimental methodologies, providing insights into their importance and applications.
Computational Approaches
Computational approaches in off-target prediction rely on algorithms and bioinformatics tools. These tools analyze genomic sequences to identify potential off-target sites. Such methods can effectively reduce the scope of experimental validation needed, saving both time and resources.
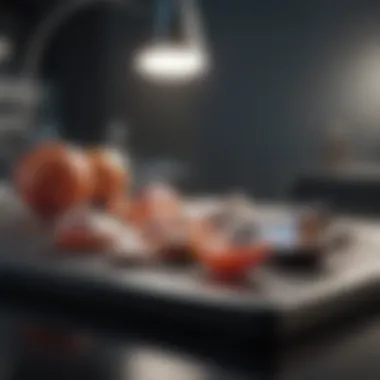
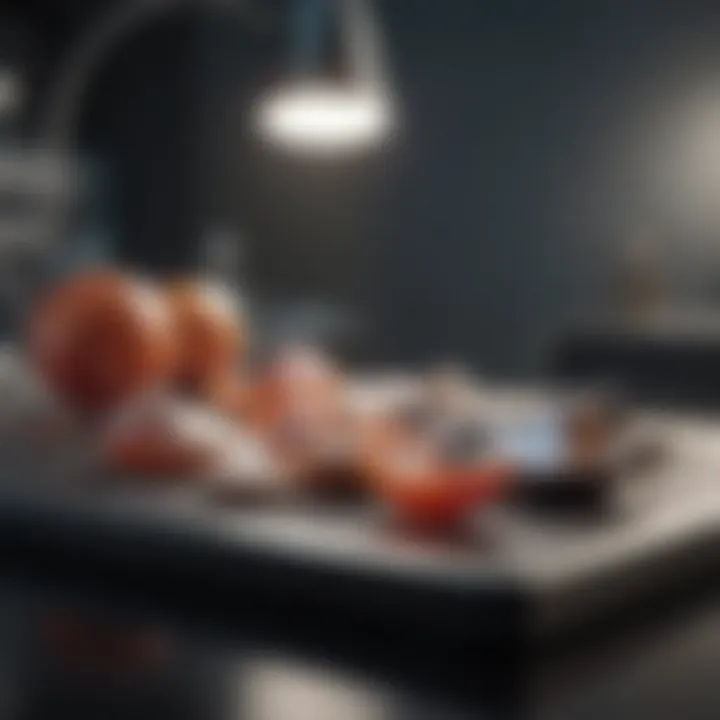
Common computational tools include CRISPRoff, Cas-OFFinder, and Off-Spotter. Each tool employs distinct algorithms to predict off-target matches based on sequence similarity. This accuracy is influenced by several factors:
- Seed Sequence: The initial portion of the guide RNA determines binding efficiency. A strong match in the seed region usually indicates higher probability for off-target activity.
- PAM Sequence: The presence of the protospacer adjacent motif is crucial. If the PAM sequence is not present near a potential off-target, editing may not occur.
- Mismatches: Some mismatches in the target sequence can still allow for successful cleavage. Computational tools often quantify these mismatches to derive an off-target likelihood.
These approaches not only cover the main genomic targets but also encompass an extensive range of potential off-targets. It is essential to manage the complexities involved in these predictions to ensure reliable outcome assessment.
Experimental Validation Techniques
While computational predictions serve as initial guides, experimental validation is essential for confirming off-target activity. This stage of evaluation provides concrete evidence of any unintended effects from CRISPR applications. Various experimental techniques are employed to validate computational predictions. These include:
- Deep Sequencing: NGS or next-generation sequencing is utilized to assess off-target effects across the genome. It allows for comprehensive analysis, often detecting low-frequency off-target events that other methods may miss.
- T7 Endonuclease I Assay: This assay detects mismatched heteroduplexes resulting from CRISPR editing. It is a straightforward method to identify off-target modifications.
- Sanger Sequencing: A traditional method used for validating precise off-target sites. It allows for targeted assessment of predicted off-target sites, confirming or dismissing potential editing events.
The combination of computational tools and experimental validation facilitates a thorough investigation into off-target risks. This two-pronged strategy is crucial for achieving precision in gene editing, ultimately enhancing the reliability of CRISPR applications across various fields of research and therapeutic interventions.
"The integration of computational methods with experimental validation is essential for understanding the full landscape of gene editing precision."
Thus, the methodologies in off-target prediction form the backbone of effective CRISPR technology, ensuring that gene editing will become more reliable and safe in its applications.
Evaluating Prediction Accuracy
Evaluating prediction accuracy is fundamental when discussing CRISPR off-target prediction tools. The advent of gene editing technologies brings a significant potential for breakthroughs in various fields such as medicine, agriculture, and biotechnology. However, the reliability of such technologies hinges on their ability to minimize off-target effects. Thus, ensuring accurate predictions of these potential unintended modifications in the genome is essential to both the efficacy and safety of gene editing applications.
A precise evaluation of prediction accuracy informs researchers and practitioners about the performance of different prediction tools. Understanding this accuracy helps in selecting the right tool for specific experiments. Furthermore, it guides the interpretation of results derived from gene editing endeavors. As these tools evolve, continuous assessment of their predictive capabilities becomes indispensable for scientists aiming to push the boundaries of genetic innovation.
This section articulates key metrics used to assess prediction accuracy, as well as case studies that exemplify these assessments in real-world scenarios.
Limitations of Current Tools
Off-target prediction tools are fundamental in the field of CRISPR technology. While they enhance precision in gene editing, it is important to recognize their limitations. Understanding these limitations can aid researchers in making informed decisions regarding their use and further development. This section will discuss the issues of false positives and negatives as well as challenges encountered in complex genomes.
False Positives and Negatives
False positives and negatives are significant challenges in predicting off-target effects. A false positive occurs when a prediction tool indicates potential off-target activity where none exists. This can lead to unnecessary caution in experimental design and wasted resources. On the other hand, false negatives happen when the tool fails to identify actual off-target sites. This discrepancy can result in unintended genetic modifications, potentially leading to harmful outcomes.
Tools often employ various algorithms and databases to predict off-target sites based on genetic sequences. However, these predictions rely on specific parameters and may not account for all variables influencing gene editing outcomes. Molecular mechanisms can be complex and may not always conform to expected patterns.
"The accuracy of off-target prediction tools directly influences the outcomes of CRISPR applications in practical settings."
Consequently, users must validate predictions through experimental methods to confirm actual activity. This validation process, however, can be resource-intensive and time-consuming. Researchers are advised to use multiple prediction tools where possible and remain cautious of the interpretation of results.
Challenges in Complex Genomes
The analysis of complex genomes presents additional obstacles. Many organisms, such as humans, exhibit highly intricate genetic architectures that pose unique challenges for off-target prediction tools. These genomes may contain repetitive sequences, paralogs, or other features that complicate accurate predictions.
In such cases, tools may struggle to differentiate between similar sequences, leading to an increased likelihood of false predictions. Moreover, variations in gene expression and chromatin accessibility can further impact the activity of CRISPR systems, thus obscuring the true off-target landscape.
As the field progresses, addressing these challenges becomes imperative. Ongoing enhancements in predictive algorithms and experimental techniques may help improve the accuracy of off-target predictions in complex genomic scenarios. Collaboration between computational biologists and experimentalists will also be critical in refining these tools to ensure they meet the evolving needs of the research community.
Future Directions in Off-Target Prediction
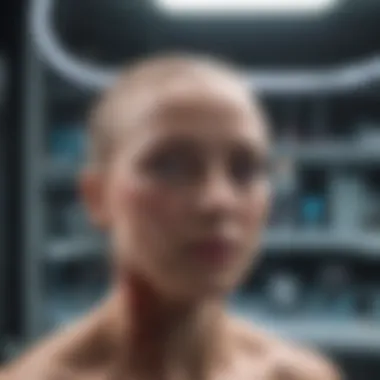
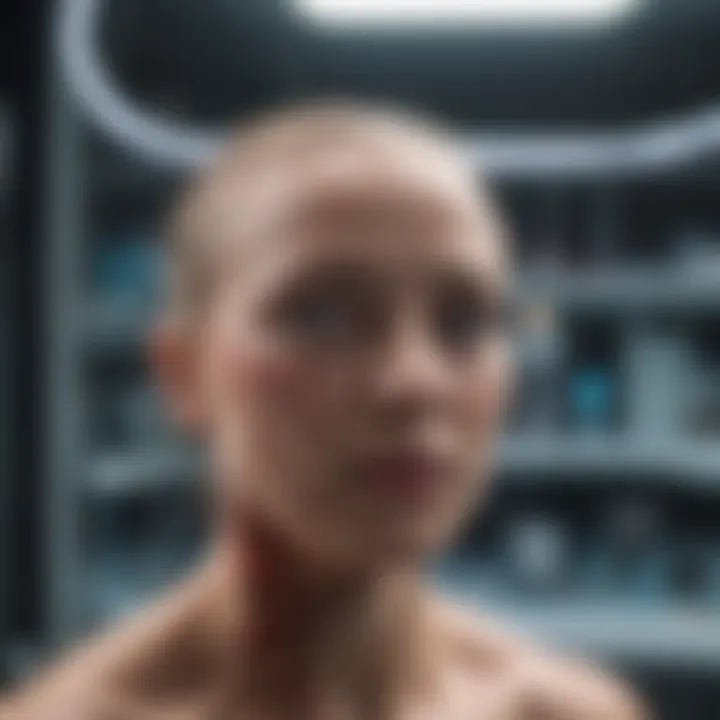
In the evolving landscape of gene editing, understanding off-target effects is essential for advancing the precision of CRISPR technology. Future directions in off-target prediction aim to enhance accuracy and reliability, greatly benefiting both research and therapeutic applications. Developing more advanced tools can facilitate better gene editing practices, leading to safer interventions and more effective outcomes. This section will focus on emerging techniques and their integration with machine learning—elements that are vital for improving prediction capabilities.
Emerging Techniques
Research is constantly unveiling new methodologies in off-target prediction. One significant trend is the development of high-throughput sequencing methods. These methods allow researchers to comprehensively analyze potential off-target sites, yielding more accurate assessments. Moreover, CRISPR-based screens are gaining traction. They utilize libraries of gRNAs to identify specific off-target binding events in various genomic contexts.
Incorporating single-cell sequencing technologies also holds promise. This approach can help distinguish between on-target and off-target effects at a granular level, providing insights into the variability of gene editing outcomes. By utilizing these innovative techniques, scientists can refine their approaches, enhancing the predictability of off-target effects.
- High-throughput sequencing techniques enable comprehensive assessments.
- CRISPR-based screens facilitate identification of binding events.
- Single-cell sequencing allows for detailed analysis.
Integration with Machine Learning
The intersection of machine learning and off-target prediction tools is an area rich with opportunities. Machine learning algorithms can process vast datasets, identifying patterns that may not be evident through traditional analysis. By training models on existing data about known off-target sites, predictive tools can provide more accurate forecasts of potential off-target effects for new CRISPR applications.
Furthermore, workflows that integrate machine learning with existing prediction tools can optimize target site selection. These algorithms can evaluate factors such as sequence similarity and structural characteristics to ascertain risk levels associated with each candidate target site. The adoption of such technologies not only increases the efficiency of the prediction process but also enhances the overall safety of gene editing initiatives.
- Machine learning optimizes existing prediction workflows.
- Algorithms analyze data to identify new insights.
- Increased efficiency leads to safer gene editing outcomes.
"The future of gene editing lies in harnessing the power of advanced tools and methodologies to ensure precision and efficacy throughout the research process."
In summary, the future directions in off-target prediction are geared towards leveraging emerging techniques and machine learning. These strategies will offer sophisticated methods to predict off-target effects with greater accuracy. Ultimately, enhancing CRISPR technology's precision is critical as it paves the way for innovative therapeutic solutions.
Ethical Considerations in Gene Editing
The integration of CRISPR technology into gene editing holds profound implications beyond the scientific realm. Ethical considerations surrounding gene editing are paramount as they guide the responsible application of this powerful tool. The potential for unintended consequences, especially through off-target effects, raises critical questions about the morality of manipulating genetic material.
Implications of Off-Target Effects
Off-target effects can result in unanticipated changes to the genome, leading to possible adverse health outcomes. This unpredictability can impact organisms significantly, most notably in therapeutic applications where precision is crucial. For instance, if a treatment designed to cure a genetic disorder inadvertently modifies other genes, it might create new health issues instead of resolving existing ones. Thus, understanding and mitigating off-target effects is not just a scientific challenge; it is an ethical obligation that researchers must navigate carefully.
"The crux of ethical gene editing lies in balancing innovation against potential risk to human life and biodiversity."
Furthermore, there is concern over equity in access to these technologies. Disparities in availability may widen the gap between those who can afford gene editing and those who cannot. This raises moral questions about who benefits from advancements in gene editing technologies. The society must ensure that gene editing is developed and implemented in a manner that prioritizes public good over profit.
Regulatory Frameworks
Establishing robust regulatory frameworks is crucial to address the ethical concerns in gene editing. Regulatory bodies worldwide must endeavor to create guidelines that ensure gene editing practices are safe and ethical. These frameworks should include provisions for transparency in research and the publication of findings, which would bolster public trust.
Critical areas for regulation include:
- Preclinical Testing Requirements: Mandatory testing for off-target effects before clinical trials can help ensure that potential risks are evaluated thoroughly.
- Public Engagement: Initiatives that facilitate discussions about gene editing's ethical implications can help demystify the technology and engage diverse voices in shaping policy.
- Accountability: Holding researchers and institutions accountable for their work is essential to maintain high ethical standards in gene editing practices.
In summary, ethical considerations form the backbone of responsible gene editing. The discourse surrounding off-target effects and the establishment of effective regulatory frameworks are central to this conversation. By prioritizing ethics, the scientific community can better navigate the complexities of gene editing and ensure its benefits are realized equitably.
End
The exploration of CRISPR off-target prediction tools highlights their vital role in the continuous advancement of gene editing technologies. These tools are not merely secondary components in the gene editing process; they are fundamental in managing precision and safety. The implications of off-target effects can be profound, influencing not only the efficacy of gene therapies but also the ethical landscape of genetic research.
In summary, these prediction tools provide researchers with essential insights to foresee and mitigate potential unintended modifications in the genome. By employing sophisticated algorithms and methodologies, scientists can enhance their ability to identify and reduce off-target interactions, thus improving the reliability of CRISPR applications.
Summary of Findings
This article has articulated several key points regarding CRISPR off-target prediction tools:
- Understanding the significance of off-target effects is crucial for safe and effective gene editing.
- A variety of prediction tools exist, each with unique features and capabilities.
- Methodologies for off-target prediction consist of both computational and experimental approaches.
- Evaluating the accuracy of these tools allows researchers to select the most suitable options for their specific needs.
- Current limitations highlight areas for improvement in future research.
- Ethical considerations should influence the development and application of gene editing technologies.
By synthesizing these findings, it becomes clear that while CRISPR technology offers transformative potential, the commitment to accuracy and ethical integrity remains paramount. The journey forward demands not only innovation in predictive tools but also a rigorous approach to their application and evaluation.