Datasets in Medical Diagnosis: Structure and Impact
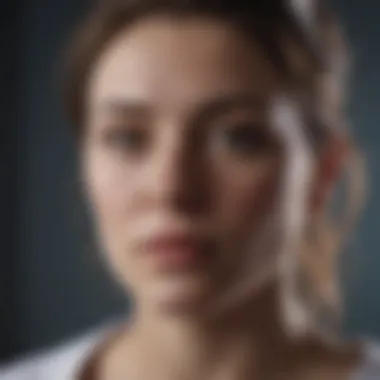
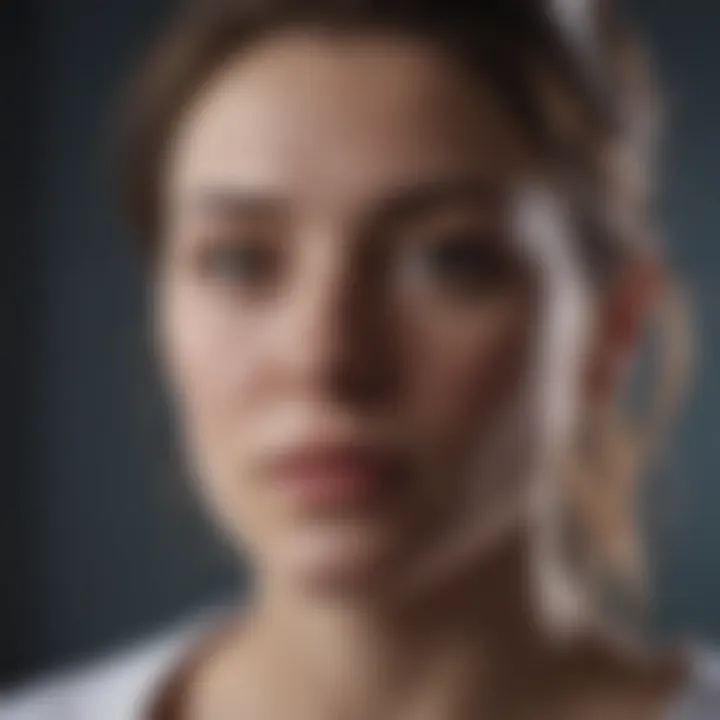
Research Background
Medical diagnosis has evolved significantly over the years, driven largely by the availability and utilization of datasets. The process of identifying diseases and conditions through clinical signs and symptoms has always been a cornerstone of healthcare. However, as technology has progressed, the way healthcare professionals approach diagnosis has transformed, necessitating a deeper understanding of the datasets that underpin these processes.
When talking about the scientific problem at hand, it is essential to recognize that diagnosing medical conditions accurately is often complicated. Factors such as variability in patient presentations, comorbidities, and the sheer volume of information that healthcare workers must process can lead to inconsistencies in diagnosis. For instance, misleading information in records or diagnostic tools can further muddle decision-making, making reliable datasets crucial.
The historical context reveals that the journey toward data-driven medical diagnoses took many turns. Early efforts date back to the development of simple databases in the 1960s and 1970s, focusing mainly on patient records. With the advent of electronic health records, the massive influx of data made it feasible to implement more sophisticated analytics. A notable breakthrough in this area was the introduction of a dataset known as MIMIC (Medical Information Mart for Intensive Care), available to researchers looking to combine clinical data with machine learning models for better diagnostic clarity.
Previous studies have highlighted how specific datasets correlate with diagnostic precision. The Framingham Heart Study, for example, underscored the significance of epidemiological data in predicting heart disease. Moreover, recent analyses have demonstrated that higher-quality datasets are linked to increased diagnostic accuracy, underscoring the critical relationship between data integrity and patient outcomes.
Findings and Discussion
The intersection of datasets and medical diagnosis yields significant findings, particularly related to diagnostic accuracy and the capacity to predict patient outcomes. A major advantage of leveraging datasets is their ability to provide a circumstantial backdrop for clinical decisions, allowing professionals to examine patterns and trends that might otherwise go unnoticed.
Key Results of Research
- A comprehensive study of over 1,000 diagnostic outcomes revealed that machine learning algorithms, when applied to well-curated datasets, improved diagnostic accuracy by approximately 15% compared to traditional methods.
- Datasets like NIH Clinical Center Data Repository have enabled longitudinal studies that illuminate the etiology of various diseases, fostering improved strategies in both predictive diagnostics and preventative care.
Interpretation of Findings
The implication of these findings is twofold: first, incorporating diverse and expansive datasets into diagnostic processes not only enhances accuracy but may also reduce the time spent arriving at a diagnosis. Secondly, as ethical dilemmas regarding patient data privacy persist, stakeholders must balance the fine line between data accessibility and the safeguarding of personal information.
"Datasets act as the bedrock upon which the future of medical diagnostics rests. Their accurate interpretation and application have the potential to redefine how healthcare is delivered."
Preamble to Medical Diagnosis Datasets
In recent years, the healthcare landscape has been undergoing a significant transformation, primarily driven by the advent of advanced technologies and data analytics. The role of medical diagnosis datasets has emerged as not just a support system for healthcare professionals but as a cornerstone in revolutionizing patient care. Understanding how these datasets function, where they come from, and their implications is crucial for anyone involved in the sphere of health research and clinical practice.
Understanding the Role of Datasets
Medical datasets can be thought of as repositories of valuable information that capture various aspects of health-related data. This includes symptoms, diagnoses, treatments, and patient demographics. They serve as a canvas on which patterns and insights can be drawn, enabling clinicians and researchers to make informed decisions.
The increasing sophistication of diagnostic tools and machine learning applications hinges substantially on the quality and comprehensiveness of these datasets. For instance, a dataset that includes diverse patient profiles enhances the predictive accuracy of diagnostic algorithms. It’s like having a multi-faceted view of a problem, giving practitioners the ability to predict outcomes more reliably.
"The blood, sweat, and tears of healthcare data is what fuels the engines of accuracy and innovation in diagnosis."
With modern medical practices leaning heavily on evidence-based approaches, the pivotal role of datasets can scarcely be overstated. From comparative effectiveness research to personalized medicine, these datasets are essential. They help identify correlations that were previously hidden, offering a new lens through which we can understand diseases and their treatments. Therefore, grasping the fundamental nature of these datasets sets the stage for improving diagnostic methods and patient outcomes.
Importance in Clinical Decision Making
When it comes to clinical decision-making, the relevance of medical datasets cannot be ignored. They empower healthcare professionals to tailor treatment plans based on empirical evidence rather than relying solely on intuition or outdated guidelines. This is especially vital in a world where each patient’s individual health profile plays a significant role in the effectiveness of treatments.
Clinical decision support systems often leverage these datasets to provide recommendations. For example, data drawn from historical patient outcomes can guide a doctor in selecting the most effective course of treatment for a new patient. In essence, medical datasets are not just numbers and records, but vital components that ensure the health decisions made today lead to better healthcare systems tomorrow.
Moreover, the ability to track outcomes over time through datasets means that healthcare providers can refine their guidelines regularly. This continuous learning cycle places an emphasis on adaptability and improvement in the healthcare process. Ultimately, understanding the value of these datasets underscores a broader recognition of their influence in clinical judgment, paving the way for enhanced patient care and outcomes.
Types of Medical Datasets
Understanding the different types of medical datasets is crucial for anyone involved in healthcare research or practice. The quality and kind of data can significantly influence diagnostic processes and patient outcomes. Each type of dataset has its own strengths, weaknesses, and specific applications in medical diagnosis. By discerning these nuances, healthcare professionals can tailor their approaches, improving both care delivery and research efficiency.
Structured vs Unstructured Datasets
When categorizing medical datasets, one of the most fundamental distinctions lies between structured and unstructured data. Structured data conforms to a predefined format, making it easily searchable and analyzable. For instance, databases such as electronic health records (EHRs) typically follow a standardized structure, which allows for rapid data retrieval and efficient analysis. In contrast, unstructured data encompasses a vast array of formats that do not adhere to a specific structure, such as clinical notes, radiology images, and audio recordings of physician-patient interactions. This variety may impede immediate analysis but can provide a wealth of information for nuanced understanding of patient conditions.
"Structured data might be likened to a neatly organized file cabinet, whereas unstructured data resembles a sprawling attic filled with assorted treasures. Each has its own value, depending on the context."
Choosing between these types hinges on the objective. For instance, structured datasets can be advantageous for statistical analysis and trend tracking, whereas unstructured datasets offer richer insights into patient experiences and clinician observations.
Clinical Trial Data
Clinical trials generate a treasure trove of data that is essential for evaluating the effectiveness and safety of new therapies or interventions. Clinicians and researchers utilize this data not just during the trials but also for future analysis. This dataset typically includes demographic information, intervention details, and outcome measures. By combining clinical trial data with real-world datasets, health professionals can gain a comprehensive view of treatment impacts over time.
Moreover, clinical trial data undergoes rigorous standards to ensure quality and reliability. This can involve maintaining documentation throughout the trial period. The integration of this data into everyday practice can vastly enhance decision-making processes, streamlining the pathway from research to clinical application.
Observational Studies and Cohorts
Observational studies represent another pivotal component of medical datasets. Unlike clinical trials that often involve strict protocols, observational studies focus on capturing data from real-world settings without intervention by researchers. This approach gathers groups of patients – termed cohorts – and collects data over time. This dataset may encompass a range of variables such as lifestyle factors, demographics, and comorbidities, offering a broad view of patient health outside structured trial conditions.
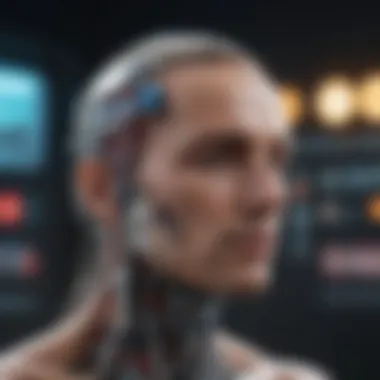
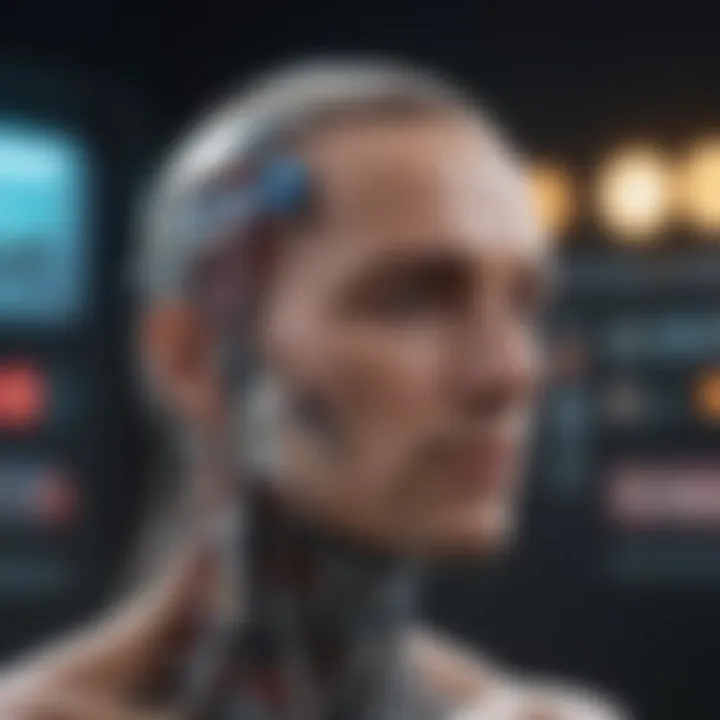
The insights derived from these studies are invaluable. By examining trends and patterns naturally occurring in populations, clinicians can develop a better understanding of disease prevalence, progression, and potential risk factors. These datasets help establish causal relationships that are critical in informing public health strategies and preventative measures.
In summary, the variety in types of medical datasets teaches us that no single category holds the key. Instead, the interplay between structured and unstructured data, coupled with the richness of clinical trial and observational study datasets, creates a holistic environment for improved medical diagnoses and healthcare interventions.
Sources of Medical Datasets
The exploration of medical datasets is incomplete without a look into their sources. The integrity of conclusions drawn from medical research depends heavily on where and how the data is gathered. Understanding the origins of these datasets is crucial for researchers, healthcare professionals, and educators alike. This discussion sheds light on the unique characteristics and advantages inherent to various sources, providing insights into how they play a pivotal role in the field of medical diagnosis.
Public Databases and Repositories
Public databases and repositories are a goldmine for researchers and practitioners aiming to harness large datasets that can inform medical diagnoses. These platforms, such as the National Institutes of Health’s (NIH) database or the UCI Machine Learning Repository, serve as communal vaults, making data freely accessible. The importance of such repositories can’t be overstated; they not only democratize access to critical information but also promote collaboration among researchers across the globe.
Using these databases allows for extensive data analysis without the constraints of financial barriers. Moreover, they provide curated data, often validated through peer-review processes, ensuring a degree of reliability. However, it's often a mixed bag; while some datasets are rich in information, others may lack comprehensiveness or may have undergone minimal scrutiny. To get to the good stuff, thorough knowledge of metadata and understanding the limitations of each database become essential.
Hospital and Healthcare Systems
Hospitals and healthcare systems are another indispensable source of medical datasets. The data generated through clinical practices records a vast array of patient encounters, treatments, and outcomes. This raw, real-world information can provide insights into population health trends and contribute significantly to predictive modeling.
A notable advantage of hospital data lies in its immediate applicability; it reflects current practices and challenges faced in real-time settings. However, accessing this data often comes with its own set of hurdles. Privacy regulations, such as the Health Insurance Portability and Accountability Act (HIPAA), impose strict limitations on data sharing to protect patient confidentiality. Therefore, clinical professionals must balance the pursuit of knowledge with ethical standards.
Collaboration with Research Institutions
Finally, collaboration with research institutions offers fertile ground for sourcing medical datasets. Such partnerships enable the entanglement of institutional resources, expertise, and archived data. These collaborations often yield high-quality, intricate datasets that benefit from rigorous methodological frameworks. Institutions such as universities frequently engage in longitudinal studies or clinical trials that produce valuable datasets.
Working in tandem with researchers not only expands the scope of available data but also enhances the credibility of the findings. So, when a student or a professional seeks to utilize medical datasets, establishing connections with academic institutions can provide access to specialized datasets that might otherwise remain out of reach.
"The source of the dataset significantly influences the robustness of the research findings. Careful consideration of these sources is fundamental in determining the dataset's reliability and ethical standing."
In essence, each source of medical datasets presents distinct advantages and challenges, making it essential for healthcare professionals and researchers to navigate this landscape thoughtfully. From public repositories to direct ties with hospitals and academic institutions, the journey to harness valuable data is rife with opportunities for impactful discoveries.
Data Privacy and Ethical Considerations
Data privacy in medical datasets is not just a regulatory obstacle but a fundamental tenet ensuring the protection of patient rights. When various datasets coalesce into clinical decision models, the ethical considerations surrounding these data's collection, storage, and use become paramount. Breaching such ethics can lead to privacy scandals, eroding trust in healthcare systems and researchers alike. Every dataset begins its life by collecting personal health information, inherently rich in detail, and potentially sensitive. Thus, balancing data utility for diagnosis and treatment with stringent privacy safeguards is crucial.
Regulatory Frameworks and Compliance
In recent years, a raft of regulations has emerged aimed at safeguarding patient information while enabling medical research. Key regulations such as the Health Insurance Portability and Accountability Act (HIPAA) in the United States and GDPR in Europe enforce strict guidelines on how patient data should be managed. These frameworks not only dictate data usage but also facilitate accountability amongst healthcare entities. The essence of compliance raises several questions:
- How do we ensure data collection adheres to ethical standards?
- Are datasets anonymized sufficiently to protect patient identities while still providing meaningful insight?
- What processes are in place to handle breaches, should they occur?
All these points emphasize the necessity for healthcare institutions to remain vigilant and educate their staff on regulatory updates.
Informed Consent Procedures
Informed consent is a cornerstone of ethical data handling. When healthcare professionals collect data for diagnosis or research, patients must be made fully aware of how their information will be used. This means explaining the purpose of the data collection in clear, accessible language, ideally free from medical jargon. If, for instance, a patient’s data will be shared with machine learning models to enhance diagnostic tools, they should understand this contribution enables advancements in healthcare that may eventually benefit many. But while patients must be informed, they also retain the right to withdraw their consent at any point. This fluidity ensures respect for personal autonomy, reinforcing that the patients remain in control of their information.
Challenges in Anonymization
Anonymization serves as a critical step in protecting privacy, allowing data utility while safeguarding identities. However, it's not always as simple as it sounds. Several challenges lurk in the shadows:
- Re-identification Risks: Emerging techniques in data analytics can piece together anonymized data. A well-intentioned dataset can unintentionally expose patient identities, especially when combined with other data sources, making strong anonymization protocols necessary.
- Data Utility vs. Privacy: Striking a balance can be tricky. Over-anonymization may render the dataset virtually useless, thus leading to suboptimal outcomes in patient diagnostics. The same dataset processed under overly stringent conditions might not produce meaningful patterns critical for medical breakthroughs.
- Technical Expertise and Resources: Not all healthcare institutions have equal access to resources to implement recommended anonymization techniques. This can lead to discrepancies in data quality across different establishments, impacting the overall trustworthiness of findings derived from such datasets.
"The intersection of ethics and data privacy is not just a legal responsibility but a moral obligation to build trust between healthcare providers and patients."
Navigating the landscape of data privacy and ethics doesn't just protect patient interests; it fosters a culture of trust and respect in the medical community. Those engaged in the handling of medical datasets must continually be aware of regulatory changes, ethical standards, and the potential pitfalls of data anonymization.
Data Quality and Reliability
Data quality and reliability stand as essential pillars in the realm of medical datasets. When dealing with medical diagnoses, the stakes are high; inaccuracies can spell dire consequences for patient care. A robust dataset can inform clinical decisions, guide treatment plans, and ultimately improve patient outcomes. Nonetheless, if the data feeding these systems is of poor quality or unreliable, the entire framework can crumble.
Assessing Data Validity
Determining the validity of data is pivotal. This involves evaluating whether the data is both accurate and relevant to the clinical questions at hand. For instance, a dataset denoting heart rates may be rendered useless if it includes erroneous entries or data from unrelated patient cohorts. The accuracy of these datasets often depends on the procedures employed during data collection and entry. Employing standardized protocols and checks can significantly enhance data quality.
Key considerations for assessing data validity include:
- Completeness: Are all relevant data points captured without omissions?
- Accuracy: Is the data free from error, particularly regarding measurements and observations?
- Relevance: Does the data pertain to the outcome measures being investigated?

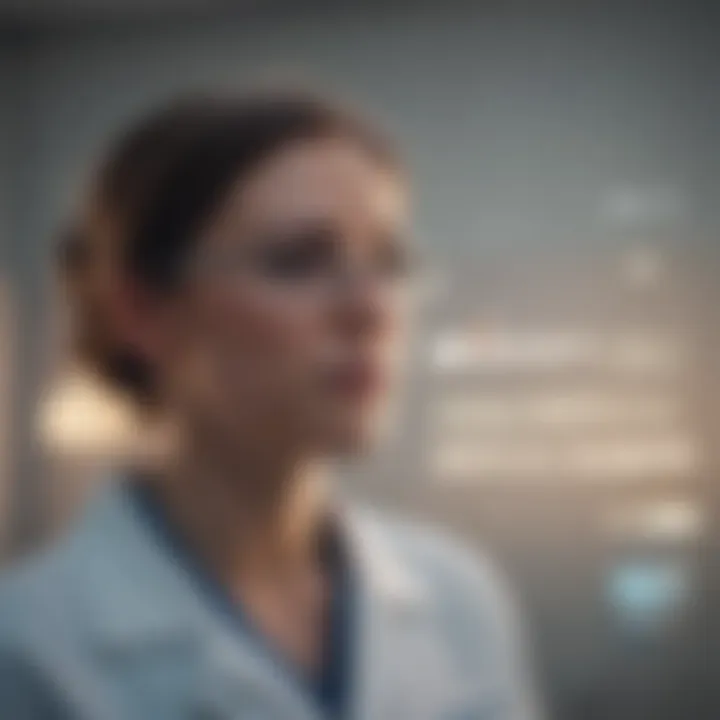
A comprehensive validation strategy should include continuous monitoring of data input methods to ensure they align with established standards. Regular audits can also spotlight inconsistencies early, preventing issues before they escalate.
Handling Missing and Incomplete Data
Missing and incomplete data can present significant roadblocks. Imagine being presented with a patient’s medical history, but crucial test results are absent. This gap could lead to misdiagnosis or inappropriate treatment recommendations. It's vital for healthcare professionals to devise strategies for addressing these deficiencies.
There are various approaches to handling missing data:
- Imputation Techniques: These statistical methods help estimate missing values based on existing data. For instance, one might use the mean or median values from related observations to fill gaps.
- Data Interpolation: This technique estimates intermediate values, particularly useful in time-series data, allowing the reconstruction of potentially lost information.
- Complete Case Analysis: In certain situations, it may simpler to exclude any records with missing data, though this carries the risk of introducing bias.
By adopting these methods, healthcare systems can mitigate the impact of missing information, ensuring that diagnosis and treatment remain sound.
Ensuring Data Consistency
Data consistency deals with maintaining uniformity across datasets, which is particularly crucial in healthcare settings where multiple sources contribute to patient information. Any inconsistencies can lead to confusion and misinterpretation of clinical data, which could adversely affect patient care. For instance, discrepancies in patient identification numbers across different databases can lead to mix-ups in records and treatment histories.
To ensure consistent data, it is essential to:
- Standardize Data Entry Processes: By following a uniform approach to how data is recorded, organizations can eliminate variations that could distort analysis.
- Implement Data Validation Rules: Setting rules and checks that automatically flag inconsistent entries can be beneficial. This might involve using algorithms that validate entries against established baselines.
- Regular Data Reconciliation: Conducting periodic reviews of datasets ensures any inconsistencies are addressed and corrected, ideally before the data informs clinical decisions.
Involving professionals who specialize in data quality within healthcare teams can also help steer initiatives toward achieving greater consistency.
Consistent, reliable data is the lifeblood of effective healthcare delivery. Without it, decision-making processes become guesswork, risking the integrity of patient care.
Machine Learning and Medical Datasets
Machine learning has become a beacon of innovation in the realm of medical diagnostics. It empowers professionals to untangle complex datasets, revealing insights that can lead to more accurate patient evaluations and treatment plans. In simpler terms, when you mix medical datasets with machine learning, you get a powerful cocktail of accuracy and efficiency that could potentially revolutionize the way healthcare operates.
One of the standout advantages of using machine learning is its capability to analyze vast amounts of data at unprecedented speeds. Imagine a physician sifting through thousands of test results a day. It’s a daunting task, but with machine learning algorithms, those datasets can be processed rapidly, and pertinent information can be extracted in a jiffy. This not only saves time but also enhances diagnostic precision.
Machine learning can also adapt and learn from new data. Picture a scenario: a particular treatment protocol shows improved patient outcomes. A machine learning model can integrate this new information and adjust its recommendations accordingly. This adaptive ability ensures that healthcare practices are continually evolving based on the latest evidence, potentially leading to better patient care.
However, it’s not all roses; there are considerations worth noting. As algorithms learn from historical data, any biases present in that data can become magnified. A trained model could inadvertently propagate these biases, leading to unequal treatment across different patient demographics.
In summary, the intersection of machine learning and medical datasets carries immense potential. While it can usher in a new era of diagnostic capability, it's crucial to remain vigilant about the ethical implications and strive for fairness in data application.
Applications of Machine Learning in Diagnosis
The applications of machine learning in medical diagnosis are as diverse as they are profound. From detecting anomalies in medical images to predicting patient deterioration, these methodologies have found their footing across various domains within healthcare.
- Medical Imaging: Algorithms can analyze images like X-rays and MRI scans, often identifying patterns that may escape the human eye. For instance, organizations like Google Health have developed successful models aimed at breast cancer detection in mammograms, achieving accuracy rates that compare favorably with expert radiologists.
- Predictive Analytics: By analyzing billions of data points from medical records, machine learning can help forecast patient outcomes. Say a hospital wants to predict which patients are at risk of readmission. By studying historical data, models can be suited to recognize patterns and alert clinicians before a patient faces complications.
- Natural Language Processing (NLP): This entails analyzing clinical notes and other unstructured data sources. The beauty of NLP lies in its ability to distill insights from vast troves of textual data, enabling healthcare professionals to draw actionable conclusions from routine doctor-patient interactions.
These applications are not just theoretical; they're making a substantial difference on the ground, transforming how healthcare professionals approach diagnosis and patient management.
Supervised vs Unsupervised Learning Techniques
To appreciate the significance of machine learning in medical datasets, one needs to distinguish between supervised and unsupervised learning techniques.
- Supervised Learning: This method requires labeled data. Think of it like teaching a child with flashcards where each card has the answer on the back. In a medical setting, if we want to train a model to identify diabetic patients, we’d need a dataset that includes various patient attributes alongside their diabetes status.Common algorithms used include:
- Unsupervised Learning: On the flip side, unsupervised learning seeks patterns without pre-existing labels. It’s akin to looking for patterns in a crowd without any prior knowledge of who’s who. This type of learning can be instrumental in clustering patients with similar health attributes, aiding in identifying potential risk groups for further study.Techniques often employed include:
- Decision Trees
- Support Vector Machines
- Neural Networks
- K-means Clustering
- Hierarchical Clustering
- Principal Component Analysis (PCA)
Both techniques serve distinct roles within the medical diagnostics landscape, enriching the actual evaluation process based on the nature of the data in hand.
Overfitting and Generalization Challenges
A crucial aspect to consider in machine learning is the delicate balance between accuracy on training data and performance on unseen data. This brings us to the concepts of overfitting and generalization.
Overfitting occurs when a model learns not only the underlying patterns in the data but also the noise. Imagine a student who memorizes answers for an exam instead of truly understanding the material; they might ace the test but fail to apply that knowledge in real-world situations. In medical terms, an overfitted model could misinterpret patient data, leading to misleading diagnostics.
On the flip side, generalization focuses on the model's application to new, unseen data. Striking that balance requires a combination of regularization techniques and validation strategies. Only through rigorous testing can we tune models to potential real-world applicability.
Combating these challenges isn’t just about refining algorithms; it often entails a deeper understanding of the datasets used, emphasizing the need for diverse and high-quality data to train these models effectively.
"Machine learning doesn’t eliminate the role of human expertise; instead, it augments it, ensuring that medical practitioners can make informed decisions supported by data-driven insights."
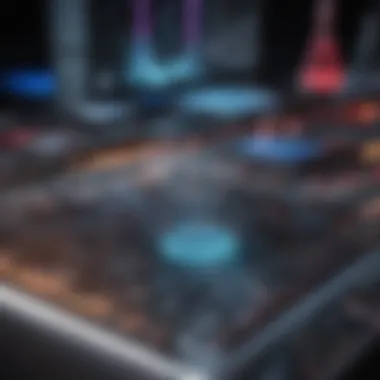
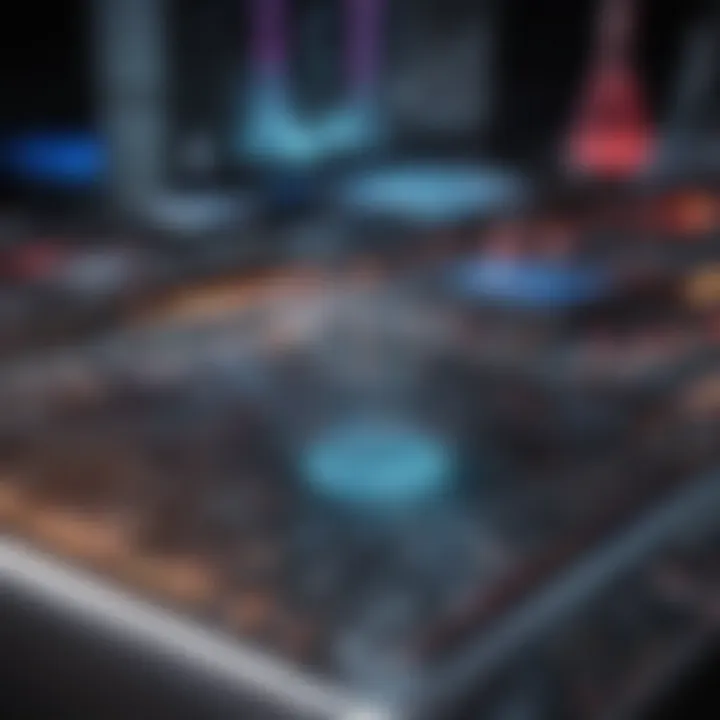
The Future of Medical Datasets
The landscape of medical diagnosis is evolving rapidly, driven by technological advances and the increasing availability of diverse datasets. This section explores the future of medical datasets, focusing on specific elements, benefits, and considerations that shape this vital aspect of healthcare. As we look ahead, understanding these dynamics is crucial for stakeholders ranging from healthcare providers to patients. The ability to harness vast amounts of data holds the promise of better diagnoses, improved patient management, and enhanced health outcomes.
Emerging Trends in Health Data Management
The field of health data management is witnessing several emerging trends that are set to reshape how medical datasets are utilized. One prominent trend is the shift towards interoperability, where different systems can communicate effectively. This paves the way for seamless data sharing among healthcare entities, facilitating a more holistic view of patient health.
Additionally, there’s a growing emphasis on decentralized data management. Unlike traditional methods that often centralize data in one location, decentralization allows fragmented data sources to be utilized more effectively. This enables researchers and clinicians to access a wider array of information, increasing the chances of uncovering deeper insights into health conditions.
Moreover, the focus on patient-generated data is on the rise. Increasingly, patients are encouraged to take an active role in managing their health, contributing their personal health data through various applications and devices, which can then be analyzed to improve diagnoses and treatments.
Integration of Wearable Technology Data
Wearable technology is becoming an integral part of modern health monitoring. Devices like smartwatches and fitness trackers now come equipped with health tracking capabilities such as heart rate monitoring, sleep patterns, and physical activity levels. This data is not just valuable for personal health management; it has far-reaching implications for medical diagnosis.
By integrating data from wearables, healthcare providers can obtain a continuous stream of patient information. This real-time data offers insights that static datasets simply cannot match. For example, fluctuating heart rates during sleep could signal underlying health issues that need immediate attention.
Furthermore, wearable data can enhance the precision of clinical trials. Researchers can remotely monitor a larger group of participants over extended periods, providing more robust data that reflects the day-to-day realities of living with a condition. This can lead to faster discoveries in treatment efficacy and safety.
Real-Time Data Analysis and Diagnostic Tools
The advent of real-time data analysis is revolutionizing how diagnostic tools are utilized in clinical settings. With the processing power of modern computing, healthcare professionals can analyze vast datasets almost instantaneously, leading to rapid decision-making.
Innovative diagnostic tools are emerging, utilizing machine learning algorithms that analyze patient data in real-time. These tools can identify patterns and anomalies that human eyes might overlook, enhancing the diagnostic process. For instance, a patient presenting with vague symptoms can be evaluated against a database of historical cases to identify potential diagnoses more quickly.
Moreover, real-time analytics allow for immediate feedback protocols. If a discrepancy is found between expected and actual patient responses, clinicians can adjust their treatment plans on the fly, improving patient outcomes. This fluidity is reshaping medicine into a more adaptable practice.
In summary, as the healthcare sector continues to embrace new technologies and methodologies, the promise of enhanced diagnoses, patient engagement, and efficient health management becomes more tangible. The journey ahead will be marked by both challenges and opportunities, demanding a concerted effort from all stakeholders to truly capitalize on the potential that medical datasets hold.
Case Studies and Practical Applications
The incorporation of medical datasets into healthcare practices serves as a linchpin for advancements in diagnosis and treatment. Case studies and practical applications provide tangible evidence of how data-driven strategies can lead to significant enhancements in clinical outcomes. These real-world examples not only demonstrate the efficacy and feasibility of leveraging medical datasets but also illuminate the path forward for researchers and practitioners alike.
Successful Implementation in Hospitals
When we look at hospitals that have embraced data-driven practices, we begin to see the transformative power of datasets. A noteworthy example is the implementation of electronic health record (EHR) systems in various healthcare settings. These systems, which rely on structured datasets to streamline patient information management, enable hospitals to access real-time data across departments. This integration fosters collaborative decision-making among healthcare professionals, ultimately enhancing patient care.
Another compelling instance comes from the case of The Mayo Clinic. By employing advanced data analytics on extensive datasets, the clinic has improved its ability to predict disease outbreaks and patient admission rates. Such predictions not only assist in resource allocation but also bolster preventative care measures. Staff can proactively address potential patient surges, thus optimizing workflow and improving service delivery.
Furthermore, hospitals that utilize predictive modeling for patient risk stratification exemplify practical applications of medical datasets. Such models help identify patients at high risk of complications post-surgery, prompting early interventions that significantly reduce readmission rates. This application is a clear testament to how data can bridge gaps in traditional healthcare approaches, yielding both economic and health benefits.
Impact on Patient Outcomes
The influence of incorporating medical datasets into healthcare cannot be overstated. The practical applications we’ve discussed showcase a notable trend: improved patient outcomes. By analyzing aggregated data on treatment methods and resultant health metrics, healthcare providers can refine their approaches and tailor them to specific patient needs.
For instance, a study in a large healthcare network noted a significant drop in medication errors after implementing a comprehensive data management system. This advancement illustrated how data analytics could bolster adherence to clinical guidelines and enhance medication safety.
Moreover, the longitudinal studies facilitated by robust datasets enable ongoing monitoring of patient progress over time. Hospitals and clinics that embrace this method have reported higher patient satisfaction and better health outcomes. This can be linked to tailored treatment plans that evolve with patient data rather than relying on outdated protocols.
"Data-driven healthcare still has ground to cover, but the strides made thus far indicate a promising horizon for patient care."
In summary, the evidence gathered from case studies paints a clear picture of the advantages of integrating medical datasets in clinical settings. With each successful implementation, the healthcare industry moves closer to realizing the full potential of data-driven decision-making, ultimately leading to enhanced patient outcomes and more efficient healthcare delivery.
End
In this discussion, we wrap up the comprehensive exploration of medical datasets essential for accurate diagnosis in the healthcare sector. The significance of this topic can't be overstated; it serves as the backbone for clinical decision-making, directly impacting patient care and outcomes. Particularly, understanding how different types of datasets interact and inform practices is crucial for all stakeholders involved in medical diagnoses.
Summarizing Key Insights
As we sifted through various facets of medical datasets, several key insights surfaced:
- Diverse Types of Datasets: The exploration of structured and unstructured data highlighted the complexity and richness of information available in the medical field. Clinical trial data and observational cohort studies unveil patterns that are instrumental for diagnostic purposes.
- Sources and Access: The array of sources—from public databases to collaboration with research institutions—underscores the importance of accessibility to quality data, which can ultimately assist medical practitioners.
- Data Ethics and Quality: It’s paramount to navigate the challenging terrain of data privacy and ethical considerations. A firm grasp of the regulatory frameworks and informed consent procedures is essential in maintaining trust and ensuring compliance in the use of medical datasets.
- The Machine Learning Revolution: The conversation around machine learning techniques in healthcare diagnostics points to an exciting frontier. This involves not only understanding supervised and unsupervised learning but also recognizing the pitfalls of overfitting in models that aim to predict patient outcomes.
These insights collectively shed light on how medical datasets revolutionize diagnostic practices and enhance patient care.
Future Directions for Research
Looking forward, several avenues for research emerge from our discussion:
- Integration of Innovative Technologies: The potential for wearable technology to contribute real-time data is promising. Research should explore how such integration can refine diagnosis accuracy and patient monitoring, leading to better long-term health outcomes.
- Advanced Data Mining Techniques: As data grows exponentially, there’s a pressing need for innovative data mining approaches. Techniques that can sift through vast datasets efficiently may significantly enhance diagnosis precision.
- Collaborative Data Sharing Models: The shift towards collaborative frameworks among healthcare providers, researchers, and institutions is essential. Future research might focus on developing models that facilitate secure and efficient sharing of medical data while adhering to ethical guidelines.
- Emphasis on Patient-centric Solutions: Understanding patient experiences through datasets is crucial. Future studies should also consider qualitative data, bolstering quantitative evidence, to create a more comprehensive picture of patient needs and treatment effectiveness.
In sum, as we stand at the crossroads of traditional practices and technological advancements, the journey of medical datasets continues to evolve. The future of medical diagnosis hinges on the effective use of these datasets, promising enhanced care and improved patient outcomes.