Exploring Sound Technology and AI Innovations
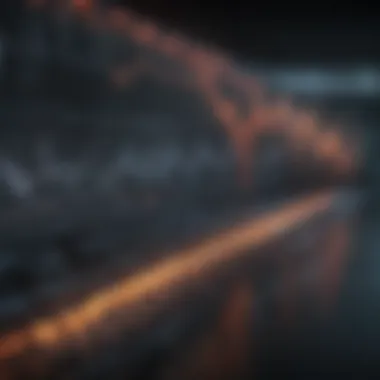
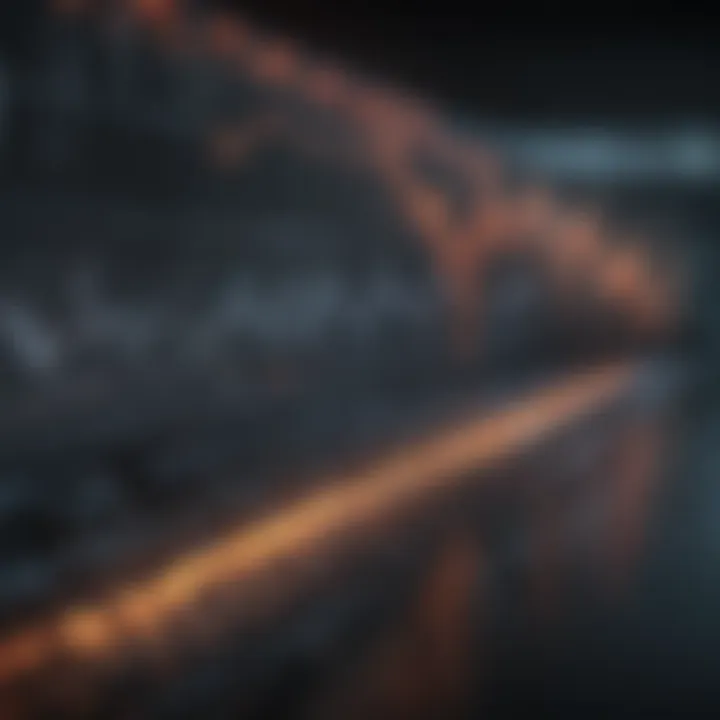
Intro
The intersection of sound technology and artificial intelligence is more than just a buzzword in today’s tech-talk. As we lean into an era defined by rapid innovation, this convergence is starting to change how we perceive, interact with, and utilize sound. Imagine an AI that can not only understand the rhythms of our daily lives but can also manipulate sound for a variety of applications—from enhancing music production to improving communication technologies. This exploration offers a path to understand deeply the intricate relationship between auditory elements and the mechanics of artificial intelligence.
Research Background
Overview of the scientific problem addressed
At its core, the scientific challenge lies in deciphering how machines can manage and interpret sound—a phenomenon that has complexities far beyond human comprehension. The field is not merely about creating algorithms that process sound; it involves developing models that capture the subtleties and nuances inherent within auditory data. Sound is a rich tapestry of vibrations and frequencies, and replicating human understanding of this via machines poses a significant challenge.
Historical context and previous studies
Historically, sound processing began in earnest in the late 20th century with the advent of digital audio technologies. Early pioneers like Max Mathews laid foundational work in computer-generated music. Fast forward a few decades, the advent of machine learning techniques began to scale up possibilities. Tools like deep neural networks have enabled machines to recognize patterns in sound data, leading to breakthroughs in voice recognition and sound classification. Significant studies have illuminated the path forward, but much remains to be discovered.
Findings and Discussion
Key results of the research
Recent research has illuminated various applications of sound AI across diverse sectors. From healthcare, where sound analysis is used to monitor patient vitals, to entertainment, where music composition is being revolutionized, the key results have underscored the broad implications of these technologies. Researchers have found that machine learning algorithms can achieve impressive accuracy in recognizing various sounds, suggesting a growing capability to interpret complex auditory cues.
Interpretation of the findings
The implications of this research extend into ethical realms, posing questions about privacy, consent, and the psychological impact of AI-driven sound technologies. Listening devices that study environmental noise or personal conversations raise significant privacy concerns. Further, as AI systems become more capable, their influence on creative aspects of sound—like music composition—could reshape artistic expressions entirely.
"The development of sound AI transcends mere technological achievements, as it beckons a future where the human experience with sound intertwines with intelligent algorithms."
The findings we explore show a promising yet complex future filled with potential and challenges. While technologies continue to grow, striking a balance between innovation and ethical responsibility is paramount.
Prologue to Sound Artificial Intelligence
The realm of sound and technology is not merely an intersection of two disciplines; it represents a profound transformation in how we engage with the auditory world. Sound Artificial Intelligence, often referred to in short form as Sound AI, encapsulates the methodologies and technologies that harness the power of sound and infuse it with machine learning capabilities. The importance of this topic is underscored by its expanding applications in various sectors—from entertainment to healthcare, and even environmental monitoring.
Understanding Sound AI is not just beneficial; it’s crucial for grasping how modern technology shapes our environments and experiences. Sound AI enables machines to interpret audio information, recognize patterns, and even generate new sounds. This capability opens doors to intuitive interfaces that extend beyond text and visuals, catering to a multidimensional audience.
Moreover, considerations regarding the ethical implications, biases in audio recognition, and the potential for misuse warrant careful discussions as the technology continues to develop.
Defining Sound and Artificial Intelligence
To appreciate the significance of Sound AI, one must first dissect its core components. Sound refers to the vibrational energy that travels through a medium, usually air, which can be perceived through our auditory system. It encompasses everything from the melodic strains of music to the inaudible murmurs of environmental conditions.
Artificial Intelligence, on the other hand, embodies computer systems capable of performing tasks that typically require human intelligence. This includes learning, reasoning, problem-solving, and, particularly relevant to our discussion, understanding sensory data such as sound.
When these ideas coalesce, we see a framework where machines are not only processing sound but also learning from it and making predictions that can be immensely beneficial—even life-saving—in certain contexts.
Historical Context of Sound Technologies
The interplay of sound and technology has roots that stretch back centuries. The early experiments with sound transmission laid the groundwork for modern telecommunication systems. From Alexander Graham Bell's invention of the telephone to Thomas Edison's development of the phonograph, history is replete with milestones that reflect the relentless human pursuit of mastering sound.
As technology advanced, so too did our understanding of auditory phenomena. Developments in digital sound recording in the mid-20th century substantially improved how sound could be manipulated. The introduction of digital signal processing (DSP) in the 1960s marked a turning point, enabling precise analysis and alteration of sound waves, paving the way for what we now know as Sound AI.
Today, Sound AI draws on these historic advancements, using algorithms to enhance sound recognition, enabling things like automatic speech recognition, music analysis, and even noise cancellation technologies. This evolution illustrates the dynamism of the relationship between sound technology and artificial intelligence, peppered with innovations that can sometimes feel almost magical.
"The future of communication transcends existing boundaries, as sound takes center stage in the orchestration of data through technology."
Mechanics of Sound Perception in AI
The essence of sound perception within artificial intelligence is a multifaceted journey that captures the intricacies of audio. As we unravel this fabric, it becomes obvious that understanding how machines interpret sound can reshape our interactions with technology. Sound is not merely a wave; it carries meaning, emotion, and context. AI captures these layers through its mechanics, interpreting soundwaves and converting them into usable data for various applications.
Getting into the nuts and bolts, sound perception isn't just about hearing; it encompasses how AI systems analyze these auditory signals. Consider this: just as humans decode speech by picking up on tone, pitch, and rhythm, artificial systems apply similar principles but with algorithms that dissect these elements with precision. The benefits of mastering sound perception in AI span across various fields, from healthcare to environmental monitoring, forming the backbone of innovative solutions. Understanding these mechanics is essential, propelling AI beyond basic recognition into realms of emotional intelligence and context-aware interactions.
Understanding Waveforms
Waveforms are the graphical representation of sound, visually encapsulating its amplitude and frequency. At their core, they give AI an essential framework to understand audio. Each waveform encodes tonal information, allowing machines to differentiate between sounds, whether it's the subtle hum of a fridge or the vibrant chords of a piano.
- Types of Waveforms: Having knowledge about different waveform types is crucial; for example, sine waves depict pure tones while complex waveforms might represent chords or noise.
- Importance of Sampling: Sampling rate also plays a pivotal role. Higher rates can capture nuances that lower rates might miss, thereby affecting the machine’s learning efficiency.
The study of waveforms is not a simplistic undertaking. This analysis influences machine learning models and their ability to accurately predict and interpret sounds. The waveform’s shape tells a story, with peaks and troughs representing variations in amplitude —each contributing a brushstroke to the canvas of sound.
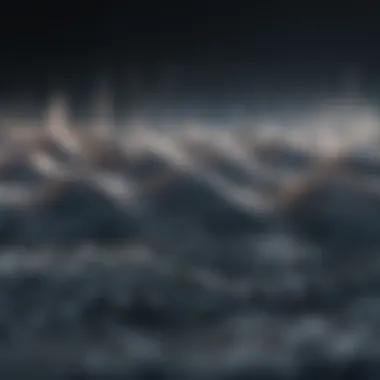
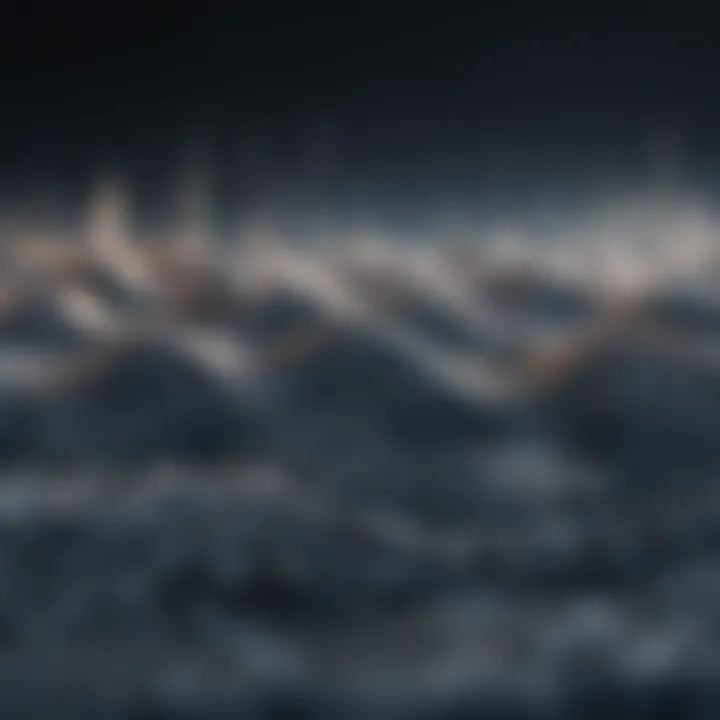
Transduction in Sound Systems
Transduction is the process that converts sound waves into electrical signals, serving as a linchpin in sound systems. It’s the transition from the physical realm of sound pressure to a digital format that machines can understand. Think of a microphone; when it captures a sound, it transforms vibrations in the air into electrical signals, which are then processed by AI algorithms.
The key processes involved include:
- Microphones: These devices utilize various transducer types, like dynamic microphones, which rely on moving coils, or condenser microphones that function through capacitive plates. Each type brings unique characteristics to sound capture.
- Analog to Digital Conversion: The captured analog signal is subsequently converted into a digital signal. This conversion is vital for any AI to process or analyze the audio.
- Importance in AI: The efficiency and accuracy of this transduction influence how well AI can learn from the data it processes. Inadequate transduction leads to loss of data fidelity, ultimately distorting what the AI interprets.
In summary, both understanding waveforms and mastering transduction in sound systems enable AI to carve a path through auditory data landscapes. As this technology develops, so does its potential to create more sophisticated, contextually aware systems that enrich user experiences across various sectors. This endeavor is not only about technology but about enriching human interaction and understanding through sound.
Algorithms Driving Sound AI
The relationship between algorithms and sound artificial intelligence is the backbone of modern auditory technology. Algorithms are not just a collection of mathematical calculations; they are the essential tools that enable machines to analyze, interpret, and generate sound. By integrating these algorithms into sound AI systems, we can achieve significant advancements in fields ranging from music generation to environmental monitoring.
Algorithms enable sound AI systems to recognize patterns in audio data, providing the foundational ability for these systems to understand complexity in sounds—be it speech, music, or ambient noise. With such capability, they do not merely listen; they comprehend. This element of understanding acts like a bridge, transforming raw sound waves into meaningful insights.
Additionally, with advancements in computation speed and data accessibility, algorithms have evolved to process vast amounts of sound data in real time, enhancing responsiveness and refining accuracy. However, it’s not all smooth sailing. The choice in algorithmic design can significantly impact performance, raising considerations regarding efficiency, processing time, and scalability.
Below are some benefits and considerations that come with leveraging algorithms in sound AI:
- Benefits:
- Considerations:
- Increased Efficiency: Algorithms can process sound data faster than human capability, speeding up analysis and decision-making processes.
- Enhanced Accuracy: With machine learning techniques, algorithms can continually improve their performance by learning from past data.
- Customization: Different algorithms can be tailored to specific applications, whether it’s analyzing complex musical compositions or deciphering spoken language.
- Data Bias: If the training data contains inherent biases, the algorithms will perpetuate these biases.
- Computational Limitations: Some algorithms require significant computational power, potentially limiting their application in less-resourced settings.
Understanding the role of algorithms in sound AI is crucial for both developing better systems and ensuring they are used responsibly. Researchers and practitioners must navigate these complexities to harness the true potential of sound AI.
Machine Learning Techniques for Sound Analysis
Machine learning techniques serve as the engine driving sound analysis in AI systems. These methods allow computers not just to process sound but to learn and adapt over time. For instance, supervised learning is frequently employed to train models to recognize various sound patterns. By feeding the system labeled audio samples—like identifying different musical genres or accents in speech—models learn to predict outcomes based on new, unseen data.
Deep learning, a subset of machine learning, has proven particularly effective in sound analysis. The use of convolutional neural networks (CNNs) is noteworthy here; they can dissect audio clips into spectrograms which visually represent sound frequencies over time. This transformation allows the model to analyze sound data in a way that highlights intricate details that could be missed otherwise.
In addition to supervised learning, unsupervised learning has become essential in identifying previously unseen patterns in sound data. For instance, through clustering methods, sound AI systems can categorize sounds into groups without needing explicit labels, potentially leading to novel discoveries.
Neural Networks in Audio Processing
Neural networks play a pivotal role in processing audio data, mimicking the interconnected neuron structure of the human brain. This architecture is inherently effective in handling the complexities of sound signals. When it comes to audio processing, recurrent neural networks (RNNs) are frequently utilized. They excel at dealing with sequences, making them suitable for tasks such as speech recognition or music sequencing.
Another key player is the long short-term memory (LSTM) network, which overcomes the limitations of traditional RNNs by remembering long-range dependencies in sound data, thereby improving context awareness in tasks involving audio. For example, when recognizing speech, LSTMs can accurately retain information on prior words, which can change the interpretation of subsequent sounds.
By combining these neural architectures with robust algorithms, sound AI systems gain the capacity to perform complex tasks—like identifying nuances in a singer's voice or detecting environmental sounds with remarkable precision. As researchers explore the frontiers of neural network models, the potential for audio applications continues to blossom.
Applications of Sound AI
In the ever-evolving landscape of technology, sound artificial intelligence has carved out a significant niche. Applications of sound AI extend far beyond what one might first imagine. From the subtle art of music composition to the critical task of monitoring environmental sounds, sound AI has become a formidable force across various sectors. It unlocks a world of possibilities and challenges, reshaping industries and influencing daily life in ways previously thought to be sci-fi fantasies.
Music Generation and Composition
The realm of music generation has experienced a seismic shift due to sound AI. Algorithms designed to compose music can generate unique melodies, harmonies, and rhythm patterns that rival human creativity. Programs like OpenAI’s MuseNet or Google’s Magenta can compose a variety of music styles, from classical to pop, within seconds. This immediacy and versatility in music generation have been a game changer for musicians looking to overcome writer’s block or experiment with new sounds.
Additionally, these AI systems are trained on extensive libraries of existing music. They analyze patterns, chord progressions, and instrumentation, offering composers a helping hand that’s both insightful and innovative. This capability raises questions about originality and authorship. How much of a piece generated by an AI can legitimately be claimed by its human collaborator? This ambiguity invites deeper debate about creativity in the age of machines.
"The future of music creation lies at the intersection of artistry and technology, where sound AI plays a pivotal role in innovation."
Speech Recognition Technology
Another prominent application of sound AI is in speech recognition technology. Tools such as Apple's Siri and Amazon's Alexa have become household staples, showcasing how sound AI can enhance everyday tasks. These systems convert spoken language into texts, understand user commands, and even engage in natural dialogues. Such proficiency stems from machine learning techniques that enable continuous improvement in speech recognition.
However, the effectiveness of these technologies often hinges on accents, dialects, and background noise. Many users have found frustration when their speech patterns lead to misinterpretations by AI. Therefore, there is a growing focus on improving the adaptability of these systems to cater to diverse populations. Addressing such concerns not only helps mitigate user frustration but also builds trust in these technologies through increased accuracy and reliability.
Environmental Sound Monitoring
Environmental sound monitoring is perhaps one of the more understated yet vital applications of sound AI. By employing algorithms that recognize and classify sounds, it becomes possible to monitor ecosystems, detect wildlife, and identify urban noise pollution. Projects like EARTH (Ecosystem Acoustic Real-time Tracking Harmony) utilize sound AI to assess biodiversity in environments like forests and oceans.
In a world increasingly affected by climate change, understanding environmental soundscapes can provide critical insights into the health of ecosystems. Sounds can indicate the presence of specific species and their behavioral patterns. Moreover, local authorities can harness this technology to manage noise pollution in urban areas, fostering healthier living environments.
The implications for scientific research and urban planning are substantial. Utilizing sound AI for monitoring can lead to more informed decisions that ultimately promote sustainability and ecological preservation.
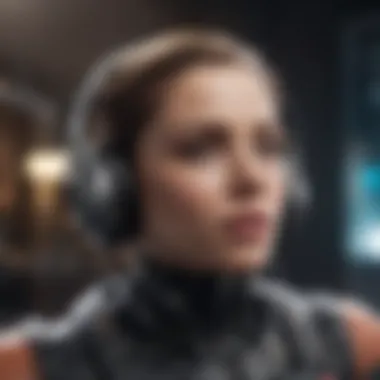
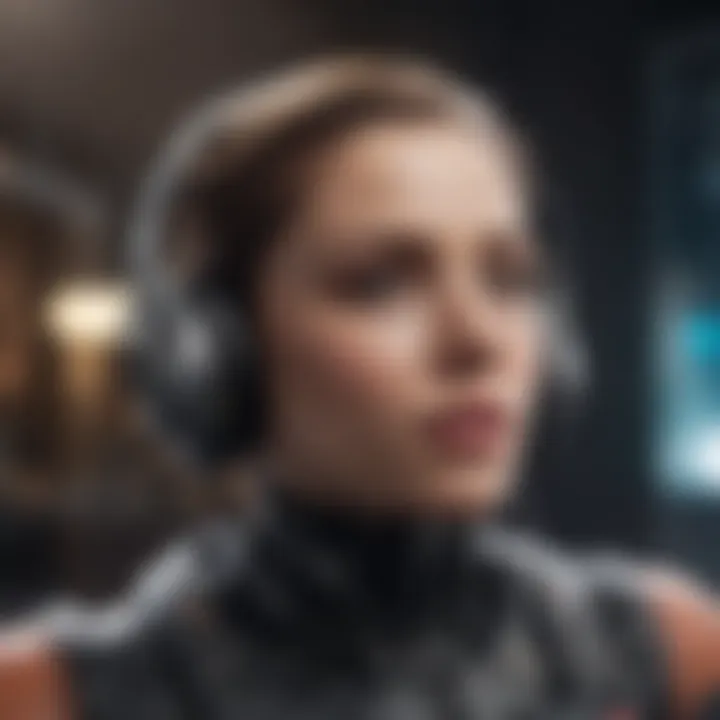
In summary, the applications of sound AI are not only expansive but also profoundly impactful. From the arts to environmental conservation, the ability to analyze, interpret, and generate sound opens new frontiers, challenging us to reconsider the role of technology in our lives.
The Role of Sound AI in Healthcare
In the healthcare sector, the intersection of sound technology and artificial intelligence raises the bar significantly. Sound AI plays a pivotal role in improving patient outcomes, streamlining processes, and enhancing diagnostic accuracy. It provides tools that can analyze sound in ways humans cannot – transforming echoes, murmurs, and other audio signals into vital health insights. The following sections delve into two critical applications of sound AI in healthcare: predictive analysis in patient care and auditory diagnostics and treatments.
Predictive Analysis in Patient Care
Predictive analytics using sound AI is becoming a game changer in managing patient health. By analyzing various sound data, healthcare professionals can harnass insights that preemptively address potential health issues. For instance, monitoring heartbeats through electronic stethoscopes can capture subtle changes that might signify risk factors for cardiovascular diseases long before traditional methods.
Sound AI systems can process vast quantities of audible data, enabling predictive algorithms to identify patterns. These patterns rely on machine learning to assess risk probabilities for various conditions based on previously recorded data. Consider how a patient with existing heart conditions could benefit from continuous heart sound monitoring, alerting medical staff to irregularities in real time. This proactive approach is crucial, especially in emergency situations, where every second counts.
"Sound is more than just waves in the air; it is a conduit for life-saving information in modern healthcare."
Auditory Diagnostics and Treatments
When it comes to diagnosing conditions, sound plays a vital role in several ways. The abilities of AI to dissect and interpret sounds make auditory diagnostics extraordinarily effective. For example, the sounds generated by certain medical devices, such as ultrasound machines or even the guttural noises from human bodies, can be analyzed by AI to identify abnormalities or confirm diagnoses.
A glaring example is the use of AI algorithms to evaluate cough sounds. Researchers have explored how sound analysis can detect conditions like pneumonia or even COVID-19. The technology listens to cough recordings and identifies specific features, correlating them with the patient's condition.
In treatments, sound AI can also enhance therapeutic methods. One such innovation is the use of AI in aural therapies. For instance, algorithms can create customized soundscapes that promote healing or relaxation based on patient feedback and physiological data.
Benefits of Sound AI in Healthcare:
- Increased accuracy in diagnosing conditions
- Improved patient monitoring and predictive capabilities
- Enhanced personalized treatment through sound analysis
- Greater efficiency in healthcare delivery processes
As these developments unfold, the implications for the healthcare field are profound. Sound AI is no longer just a technological curiosity; it is a crucial asset that shapes healthcare delivery and patient outcomes.
Ethical Considerations in Sound AI
The integration of artificial intelligence into sound technology presents not just opportunities but also a plethora of ethical challenges. As we navigate this dynamic intersection, it’s pertinent to reflect on how these issues can shape future developments. Specifically, as sound AI systems are increasingly used in various sectors, understanding ethical considerations is crucial for ensuring innovation aligns with societal values.
One significant aspect relates to the implications of collecting and processing audio data. In our tech-driven world, privacy concerns are at the forefront of such discussions. The data that sound AI systems harness can often be highly sensitive, encompassing personal conversations and ambient noises from private settings. With the advancement of technologies that can record and analyze sounds unobtrusively, maintaining the integrity of individual privacy becomes paramount.
Moreover, as AI systems grow smarter and more capable, there arises a responsibility to ensure that biases, whether in data input or algorithmic processing, do not lead to discriminatory outcomes. Analyzing the audio landscape reveals how bias can sneak into audio recognition systems, impacting not just accuracy, but shaping perceptions in broader societal contexts. Thus, an ethical framework guiding the application of sound AI will not only enhance trust but also foster equitable access to these evolving technologies.
"Ethics isn't just about following rules; it's about making choices that align with our fundamental values."
Privacy Concerns
In the ever-evolving realm of technology, privacy concerns are looming larger than life. Sound technologies, specifically those powered by AI, tap into a vast expanse of audio data, which may range from mundane background noise to highly personal conversations. This data collection can take various forms, including speech recognition in virtual assistants and constant monitoring in smart devices. While they may possess certain conveniences, they also raise significant fears about surveillance and the erosion of privacy.
Here are some areas of concern that demand attention:
- Informed Consent: Many users are unaware of how their audio data is being collected, analyzed, and utilized. Ensuring that users are informed and can give consent is crucial.
- Data Security: Storing sensitive audio data poses risks. If breached, personal information could fall into the wrong hands. This is particularly concerning for home devices that actively listen for commands.
- Potential Misuse: The capability to record and analyze sounds opens doors for misuse. From unauthorized surveillance to data exploitation, the ethical ramifications are staggering.
Navigating these challenges requires a robust regulatory framework and transparent practices that prioritize user rights without stifling innovation.
Bias in Audio Recognition Systems
The next pressing ethical issue in sound AI revolves around bias. Sound recognition systems, like all machine learning tools, are only as good as the data they are trained on. If this data lacks diversity, any resulting algorithms run the risk of perpetuating stereotypes or excluding particular groups altogether.
Consider how an audio recognition system might struggle with accents or dialects not adequately represented in its training dataset. The implications are vital:
- Discriminatory Models: If a system can’t accurately recognize speech from diverse backgrounds, certain demographics may find themselves systemically disadvantaged.
- Automatic Assumptions: Bias in audio processing can lead to flawed results, foster distrust, and reinforce societal biases, damaging relationships between technology and communities.
- Equitable Solutions: Addressing bias requires inclusive data practices and continuous assessment of algorithms to ensure fair outcomes.
As the layers of these issues unfold, what remains clear is that both developers and users have a collective responsibility to advocate for ethical practices within sound artificial intelligence. Ultimately, the goal should be a sound landscape that genuinely reflects and respects diversity, while also safeguarding privacy.
Challenges in Sound AI Development
As the field of sound artificial intelligence expands, it encounters a multitude of challenges that can hinder its progression and practical application. Navigating these challenges is crucial not just for developers, but also for industries that stand to reap the benefits of sound AI. Many of these hurdles often intersect and compound the difficulties faced in sound processing, making it essential to address them comprehensively.
Data Collection Issues
One of the major roadblocks in the development of sound AI is data collection. The algorithms that underpin sound AI systems rely heavily on vast amounts of audio data to function effectively. However, acquiring quality data is no easy feat.
- Quality vs. Quantity: It isn't enough to just have a mountain of data; the quality of this data is paramount. Many datasets contain noise or irrelevant information that can skew learning outcomes. Inaccuracies in the dataset can lead to algorithms that are either too sensitive to certain sounds or too dismissive of others.
- Diversity of Sound Samples: Sound AI needs to recognize a wide variety of sounds, ranging from spoken language to ambient environment noises. If the dataset is not diverse enough, it can create blind spots in the AI’s recognition capabilities. For instance, if most of the training data come from urban settings, the system might perform poorly in rural environments where sounds differ significantly.
- Ethical Concerns in Data Acquisition: The methods used to collect audio data often raise ethical questions. Privacy issues become paramount when utilizing recordings of human speech, especially in sensitive situations. Ensuring transparency in the data collection process is critical to maintaining the trust of stakeholders.
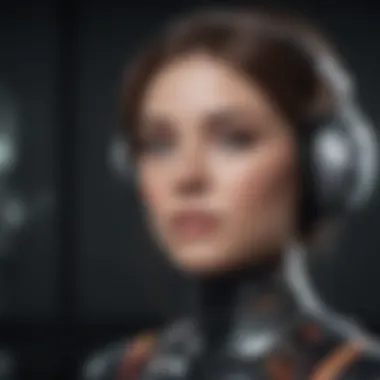
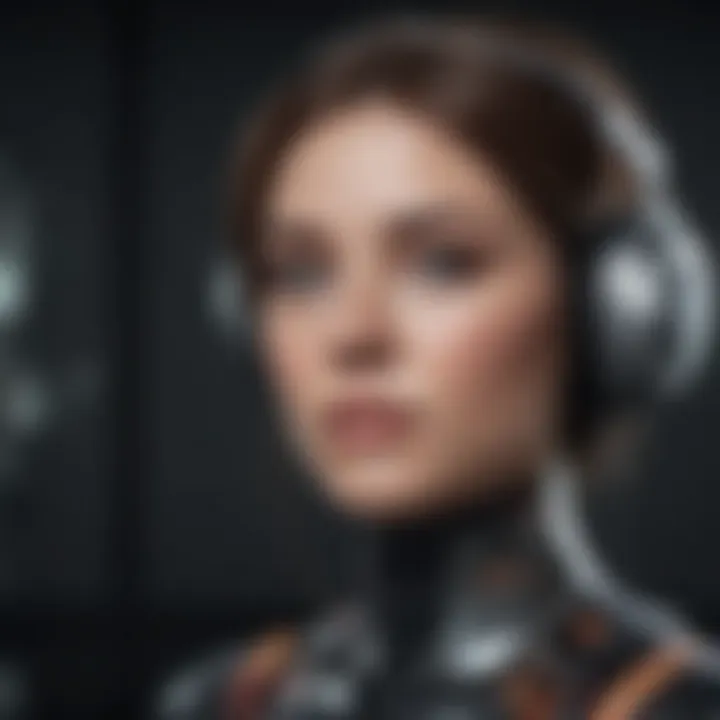
Technical Limitations of Current Systems
Apart from data collection woes, the technical limitations of existing sound AI frameworks pose significant challenges. Many systems today boast impressive capabilities, yet several constraints persist:
- Real-time Processing: Sound AI needs to analyze data instantly, which can be a tall order for current systems. Delays in processing can lead to a disjointed user experience, particularly in applications like speech recognition or live sound monitoring.
- Resource Intensive: The computational load required for sound analysis can be heavy. Many existing systems demand high-performance hardware, which can limit deployment in environments with budget constraints or lower computational capabilities.
- Limited Understanding of Context: Current sound AI lacks a robust understanding of context. For example, words that sound similar might lead to different meanings based on their usage. Without contextual understanding, the system can misinterpret critical information, affecting outcomes significantly.
"Addressing the technical limitations of sound AI isn't merely a task for engineers; it is fundamentally about creating systems that understand and respond to the complexities of human interaction."
Future Directions of Sound AI Research
As we stand at the intersection of sound technology and artificial intelligence, examining the future directions of sound AI research becomes crucial. This topic is not merely an academic exercise but a glimpse into the landscapes that will shape our interaction with technology in years to come. Exploring these frontiers enables us to anticipate how these innovations can be harnessed effectively across various domains.
Integrating Sound AI into Smart Environments
In today's world, the notion of smart environments isn’t just a fantasy anymore; it’s becoming a reality. The integration of sound AI into these spaces could significantly enhance the user experience. Consider a smart home where sound AI acts as the nervous system, responding to auditory cues.
- Ambient Sound Recognition: Imagine waking up to your home recognizing the sound of the coffee grinder, automatically adjusting the lighting to a cozy brightness, while playing soft morning tunes. Sound AI can help create a seamless environmental interaction.
- Dynamic Adjustments: Sound AI can adapt based on auditory inputs. For instance, in a busy café, the system could use algorithms to detect background noise levels and adjust music volume accordingly—keeping the vibe lively without overwhelming conversations.
- Accessibility Features: Integrating sound AI also paves the way for enhanced accessibility. People with disabilities can benefit from voice-activated systems that recognize commands amidst background noise, creating a more inclusive environment.
The vision for smart environments is increasingly intertwined with everyday experiences, making them more intuitive and responsive. This level of integration not only satisfies user expectation but also opens avenues for research into interaction dynamics, privacy, and user behavior.
The Role of Multimodal AI Systems
Looking ahead, the synthesis of different types of data through multimodal AI systems presents exciting opportunities in sound AI research. Rather than siloing sound analysis, incorporating visual, textual, and auditory data can create rich, contextual insights.
- Enhanced User Experience: By merging sound data with visual inputs, AI systems can better interpret situations. A video conferencing application, for instance, might analyze not just voice tones but also facial expressions alongside audio, leading to more nuanced interactions.
- Sophisticated Analytics: The combination of modalities allows for advanced analytics. In the healthcare sector, by fusing patient vocal tones and medical data, predictions about emotional states can be made, leading to proactive care strategies.
- Innovative Applications: In education and training, multimodal systems can offer tailored feedback. For instance, language learning apps could assess pronunciation through sound while also evaluating written skills, providing a holistic learning approach.
As we explore these advancements, it’s essential to consider ethical dimensions, data security, and potential biases that could emerge from integrating different types of AI systems.
"The sound of innovation often drowns out the warnings. As we push boundaries, we must remain vigilant about the ethical implications of our pursuits."
Overall, the future of sound AI research holds immense potential. By incorporating these advancements into everyday life, we not only redefine user interaction but also pave the way for a future where sound enhances our realities.
Industry Insights and Collaborations
In the fast-evolving landscape of sound artificial intelligence, collaboration between various sectors plays a pivotal role. Companies and research institutions are forming partnerships, ensuring knowledge transfer and facilitating innovations that can redefine sound technologies. These collaborations serve as a bridge, merging the latest advancements in AI with practical applications in sound, allowing us to better understand the nuances of auditory information processing.
One notable benefit of these collaborations is the pooling of resources and diverse expertise. When tech companies team up with academic institutions or healthcare organizations, they combine theoretical knowledge, technological tools, and real-world scenarios. This varies from developing sophisticated algorithms to deploying sound AI systems in practical environments, thereby accelerating the pace of innovation.
Additionally, industry insights gleaned through these partnerships are crucial for guiding future developments. For instance, understanding how sound recognition can improve user experiences shapes product design, leading to smarter audio interfaces in consumer gadgets. As these companies share findings and trends, they set the stage for more refined and user-friendly technologies.
"Partnerships in sound AI are not just about technology; they represent a commitment to crafting better auditory experiences for users everywhere."
Moreover, these collaborations encourage interdisciplinary approaches, blending insights from psychology, neurology, and sound engineering. The result is a more holistic understanding of how sound affects human interactions, ultimately leading to more effective healthcare applications and immersive media experiences.
Leading Companies in Sound AI
In the realm of sound AI, several key players stand out, each making significant contributions to the development and implementation of these technologies. Companies like Google and Amazon have integrated sound recognition capabilities into their smart assistants, allowing users to engage with their devices in more human-like ways. These tech giants invest heavily in research, advancing natural language processing and sound analysis.
Further innovations are seen in Apple, which has been utilizing sound AI for its noise cancellation features and spatial audio experiences. Their focus on enhancing user experience through sound technology has elevated the standard in personal audio.
Not to be overlooked, Spotify is leveraging sound AI to analyze listening habits, curating personalized playlists and improving recommendation algorithms. By tapping into user data, they ensure an enriched auditory experience.
Research Institutions Making Strides
On the academic front, research institutions play a vital role in pushing the boundaries of sound artificial intelligence. The MIT Media Lab, for example, is known for exploring new frontiers in sound technology, from machine learning models that interpret music to systems that enhance sound recognition in various environments. Their commitment to interdisciplinary research facilitates breakthroughs that industry players can later integrate into commercial products.
Moreover, universities like Stanford and Carnegie Mellon are conducting valuable research on neural networks applied to audio data, contributing to advancements in speech recognition and environmental sound classification. These institutions often partner with industry leaders to ensure that their findings translate into practical applications that can be tested and further refined in real-world settings.
Thus, the interplay of industry and academia is instrumental in sculpting the future of sound AI. The insights drawn from these collaborations help to navigate the complex challenges of sound recognition and audio processing while unleashing the potential for novel technologies that can significantly enhance the way we experience and interact with sound.
Culmination
The intersection of sound technology and artificial intelligence marks a significant frontier in innovation, bringing numerous advantages and considerations to the forefront. This article has sought to elucidate the multifaceted roles that sound AI plays across various sectors, exploring how it transforms our daily lives, facilitates advancements in healthcare, enhances artistic expression, and creates greater efficiencies in environmental monitoring. The findings reflect a landscape where sound AI is not just a tool but a ubiquitous presence that shapes interactions and perceptions in profound ways.
Summary of Key Findings
The exploration led to several key insights:
- Integration of Sound and AI: The seamless marriage between sound technologies and artificial intelligence has birtheed applications ranging from speech recognition to complex auditory diagnostics. These innovations exemplify how AI can be harnessed to interpret sounds, delivering precision that exceeds human capabilities in specific contexts.
- Impact on Healthcare: Sound AI's role in healthcare emerges as particularly significant, showcasing predictive analytics that can enhance patient care. The adoption of auditory diagnostic tools has the potential to revolutionize how conditions are assessed and treated, suggesting a future where early detection of illnesses becomes routine.
- Ethical and Technical Challenges: While the promise of sound AI is vast, this synthesis does not overlook the ethical pitfalls. Privacy issues and the inherent biases in audio recognition systems highlight areas needing careful governance and oversight. Addressing these challenges will be vital for the sustainable growth of this technology.
- Future Applications: The potential for further integration of sound AI into smart environments presents an exciting horizon. The rise of multimodal AI systems signifies a shift that could redefine standard productivity and interaction paradigms across numerous industries.
The Future of Sound Artificial Intelligence
Looking ahead, the trajectory of sound AI is promising. As technology evolves, the capability to merge sound intelligence with other modalities—like visual or tactile algorithms—will likely reach new heights. This symbiotic growth may lead to advancements such as:
- Enhanced smart city solutions where ambient sounds contribute data for urban planning and safety.
- More immersive experiences in virtual reality, where sound AI plays a key role in simulating lifelike auditory environments.
- Innovations in music therapy, utilizing AI-driven compositions tailored to individual emotional needs, potentially augmenting therapeutic practices.