Exploring Techniques for Effective Data Collection Methods
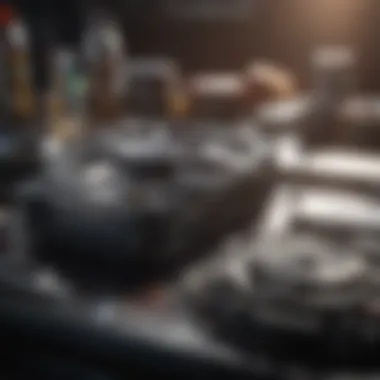
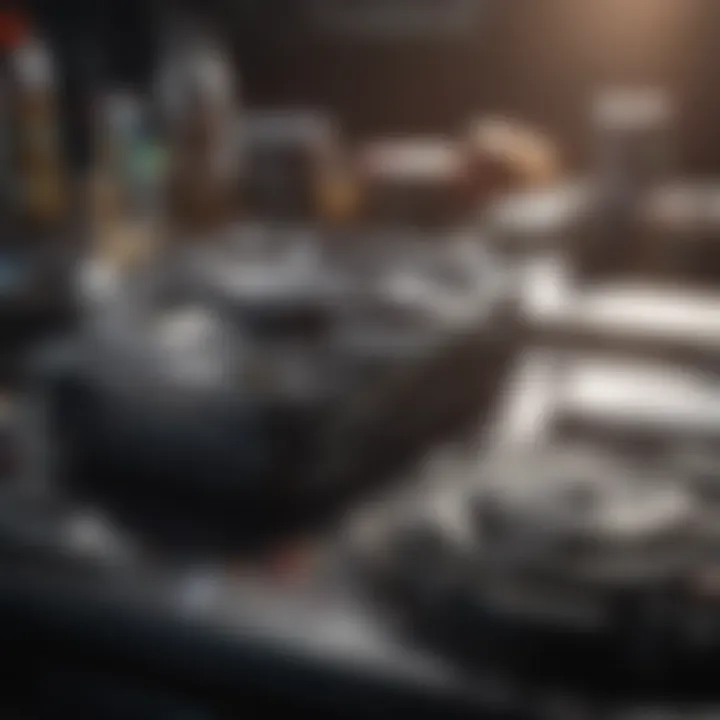
Intro
In the vast realm of research, data is considered the lifeblood that fuels inquiry and discovery. Yet, the means by which that data is gathered can greatly shape the outcomes and implications of a study. This article embarks on a journey through the diverse techniques employed for collecting data, an essential topic for researchers, educators, and anyone intrigued by the science behind understanding our world. Whether it's a survey conducted in a bustling urban area or an in-depth observation in a controlled lab setting, each method presents its own strengths and drawbacks.
"The methods of data collection form the backbone of empirical research, determining the quality and validity of the findings."
Research Background
Overview of the Scientific Problem Addressed
At the crux of any research endeavor lies a critical question or problem that needs clarity. For instance, when studying human behavior, researchers might grapple with understanding the influences of social media on mental health. Delving deep into such questions requires not just insightful theories but also robust data collection methods that could elucidate the intricacies involved.
Historical Context and Previous Studies
Historically, data collection techniques have evolved significantly. Techniques that were once heavily reliant on manual data entry and paper surveys have given way to digital tools and advanced methodologies driven by technology. Earlier studies often relied on anecdotal evidence or small sample sizes, making wide generalizations difficult. As disciplines grew more involved, so too did the need for rigorous approaches, leading to reinterpretations of established methods. Researchers began to favor triangulating their findings through mixed methods, ensuring greater reliability and validity.
This evolution continues today, as new technologies change the landscape of data gathering. Today’s scholars are increasingly exploring agile methods, responsive to the fast-paced societal changes, paving the way for innovative approaches in data collection.
Prelims to Data Collection
Data collection represents a pivotal phase in the research process, serving as the backbone for producing meaningful findings. The methods chosen for gathering data can significantly affect the quality and reliability of the overall outcomes. The heart of every study, whether it digs into the intricate nuances of social behavior or the complex pathways of biological systems, hinges on how well data is collected. This article delves into various techniques, understanding their strengths and weaknesses, and how they can be strategically employed to bolster research.
The Importance of Data Collection
In our increasingly data-driven world, the importance of data collection cannot be overstated. Not only does it provide a foundation for analysis, but it also informs decisions across multiple sectors, from policy-making to business strategy. Well-collected data ensures that the conclusions drawn are valid and replicable. For example, imagine a public health researcher examining the effects of a new vaccine. If the data collected on its efficacy is flawed, the implications could lead to misguided public health policies that may affect thousands, if not millions.
Additionally, data collection enhances our knowledge base. When researchers share their findings, they contribute to a larger pool of information that can be utilized by others in the field. This communal knowledge fosters collaboration and innovation, which in turn drives progress. Ultimately, a strong understanding of data collection contributes significantly to the integrity of research and its applicability in real-world scenarios.
Objectives of Data Collection
Establishing clear objectives for data collection is critical for a successful research endeavor. These objectives guide the selection of appropriate methodologies, help to clarify the purpose of the research, and ensure that the end results align with the initial goals. Here are some prominent objectives:
- Informative Purposes: The primary aim is often to gather information that will answer specific research questions. This means defining what information is required and ensuring that the methods chosen can yield reliable results.
- Comparative Analysis: Sometimes, researchers collect data with the intention of comparing different groups, variables, or outcomes. Here, it's crucial to define the parameters thoroughly to ensure an apples-to-apples comparison.
- Hypothesis Testing: In many scientific fields, the objective is to test a hypothesis. Collecting data in a systematic and structured way allows for statistical analyses that can confirm or refute these hypotheses.
- Trend Identification: For researchers studying changes over time, collecting longitudinal data becomes essential. Objectives here revolve around tracking these changes to draw meaningful conclusions.
Data collection isn't just a step in research; it's a structured approach that seeks to answer key questions and address concerns within various fields of study. Recognizing its significance and setting clear objectives lays the groundwork for successful research outcomes.
Qualitative Data Collection Methods
Qualitative data collection methods play a pivotal role in understanding human behavior, experiences, and motivations. Unlike quantitative techniques that often reduce complex phenomena to mere numbers, qualitative methods delve into the intricacies of social contexts, offering depth and richness in the data gathered. These approaches are especially valuable when researchers seek to explore phenomena that aren’t easily quantifiable, such as emotions, perceptions, and motivations. By employing methods like interviews and ethnographic studies, researchers can obtain nuanced insights that wouldn’t emerge from statistical analysis alone.
Interviews as a Data Collection Methodology
Interviews offer a versatile toolkit for qualitative data gathering. They can be tailored to different research objectives, informing and enhancing the research process significantly. They facilitate a direct dialogue between the researcher and participant, allowing for an adaptive exploration of topics. This adaptability comes in three key forms: structured, unstructured, and semi-structured interviews.
Structured Interviews
Structured interviews adhere to a strict format, where the same set of questions is asked to every participant. This uniformity is their biggest selling point. It allows researchers to collect data that is easier to compare across different respondents. The advantage here is clarity and simplicity, as every interview follows the same script, providing consistency in the data collected.
However, there are trade-offs. The rigidity of structured interviews might stifle spontaneity and limit the depth of responses. Participants may feel constrained and provide more superficial answers, which can lead to gaps in understanding. Despite this, structured interviews remain a popular choice, especially in large-scale studies where comparability is crucial.
Unstructured Interviews
Unstructured interviews, on the contrary, are much more fluid. There are no predefined questions; instead, the researcher steers the conversation based on participant responses. This organic approach fosters a natural dialogue, encouraging participants to express their thoughts freely.
The main benefit of unstructured interviews is depth. They allow researchers to capture complex views and richly textured descriptions of participants’ thoughts and feelings. But a key challenge with this method lies in its variability, making analysis complex and time-consuming. Also, the absence of a structured approach could lead to inconsistencies between interviews, complicating comparative analysis. Nevertheless, when the goal is to uncover latent insights, unstructured interviews stand tall.
Semi-Structured Interviews
Moving between the two extremes are semi-structured interviews. Here, researchers prepare a list of questions but retain the flexibility to explore topics that arise during the conversation. This method strikes a balance between the rigidity of structured interviews and the freedom of unstructured ones.
One significant advantage of semi-structured interviews is that they can adapt to the flow of conversation while ensuring that key topics are addressed. This flexibility helps researchers to explore new avenues that participants might open during dialogue, enhancing the richness of collected data. However, semi-structured interviews can also lead to variations in how questions are interpreted and answered, which might complicate analysis as well.
Focus Groups: Insights through Interaction
Focus groups bring individuals together in a moderated discussion, leveraging group dynamics to generate diverse perspectives on specific topics. By facilitating interaction among participants, researchers can gain insights that may not emerge in one-on-one interviews. This method allows for the exploration of collective opinions and attitudes, paving the way for deeper understanding through social interaction.
Focus groups can shine a spotlight on emerging themes or shared values. However, managing group dynamics can be tricky; dominant personalities may overshadow quieter members, potentially skewing data. It's essential for moderators to cultivate an inclusive environment where all voices can be heard.
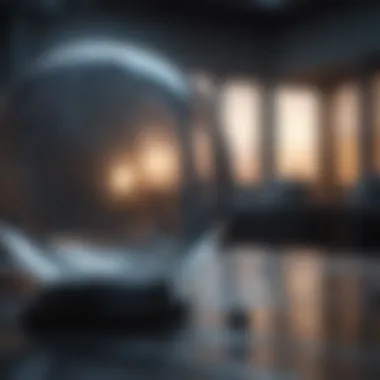
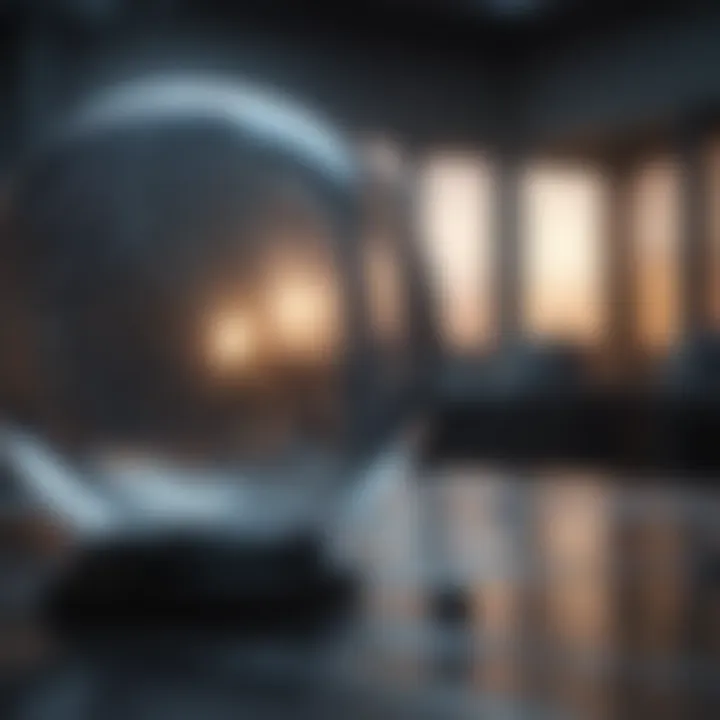
Ethnographic Studies for In-Depth Analysis
Ethnographic studies take qualitative data collection a step further by immersing researchers in the environments of their subjects. This method involves prolonged observation, allowing researchers to see and interpret behaviors in real context. Ethnography thrives on the intricate details of daily life and cultural practices, making it a valuable approach for understanding complex social phenomena.
In summary, qualitative data collection methods provide rich, textured insights that quantitative data often cannot capture. Each approach—whether structured interviews, unstructured interviews, semi-structured interviews, focus groups, or ethnographic studies—has its unique strengths and challenges, offering researchers various tools to suit their specific needs.
Quantitative Data Collection Methods
Quantitative data collection methods play a crucial role in research as they provide a robust means of gathering numerical data that can be analyzed statistically. These methods are essential for researchers seeking to measure variables and identify patterns, offering a more objective view of data compared to qualitative methods. In this section, we will delve into the various quantitative methods, emphasizing their benefits, specific elements, and important considerations.
Surveys and Questionnaires
Design Considerations
Design considerations are fundamental when crafting effective surveys and questionnaires. A well-thought-out design ensures that the questions are clear, relevant, and structured in a way that elicits accurate responses. The key characteristic of effective design lies in its ability to minimize bias and confusion, both of which can skew results. Consequently, thoughtful design becomes a beneficial choice, as it significantly impacts the data's reliability and validity.
Moreover, a unique feature of strong survey design is the use of pilot testing. Before rolling out a survey, testing it on a small group can unveil ambiguities or misleading phrases. This process increases the chance of gathering high-quality data, yet it also comes with the downside of needing additional time and resources.
Online Surveys
Online surveys have become a go-to method for collecting data due to their convenience and cost-effectiveness. The rise of technology makes them an appealing choice for gathering responses from diverse populations. One key characteristic is their accessibility across various devices, allowing participants to respond at their own convenience. Furthermore, a unique feature of online surveys is the ability to analyze data in real-time. Researchers can quickly assess response patterns and make immediate adjustments if necessary. However, the downside is the potential for lower response rates if not coupled with effective outreach strategies.
Telephone Surveys
Telephone surveys serve as an effective means of gathering data while providing a more personal touch compared to online methods. They enable researchers to clear up misunderstandings on the spot, ensuring clarity. The key characteristic of telephone surveys is their ability to reach populations that are potentially less tech-savvy or prefer direct interaction.
The unique trait of telephone surveys lies in the rapport that can be built during the call. When respondents feel comfortable, they may provide more detailed answers. However, this method can be time-consuming and costly, as it requires trained interviewers and scheduling.
Experiments in Scientific Research
Controlled Experiments
Controlled experiments are a cornerstone of scientific research, as they allow for clear causation studies between variables. One major aspect of controlled experiments is the ability to isolate the effect of one independent variable while holding others constant. This precision makes them invaluable for testing hypotheses. The foremost characteristic is the high level of control over variables, ensuring reliability in outcomes. They are a favored choice for researchers needing unequivocal answers. The structured approach also allows for repeatability, a key component in verifying findings. However, the downside is that controlled settings may not always accurately reflect real-world conditions, making external validity a concern.
Field Experiments
Field experiments offer the advantage of studying subjects in their natural settings, thereby enhancing the ecological validity of the findings. One specific aspect is how these experiments can capture behavior that is often missed in controlled environments. The key characteristic is the real-world applicability, providing insights that informed controlled setups might overlook. In field experiments, researchers can observe phenomena as they naturally occur, providing a more authentic picture. However, this approach often complicates the isolation of variables, leading to potential confounding factors. The logistical challenges of executing these experiments in unpredictable environments can also pose significant obstacles.
Observational Studies
Observational studies entail systematic observation and recording of behaviors or events without manipulating variables. This approach is particularly useful when experimentation is not feasible for ethical or practical reasons. One significant aspect is its versatility, allowing for the examination of phenomena as they occur in real time.
The key characteristic of observational studies is their non-intrusive design, giving a clearer picture of genuine behaviors. However, this method does have limitations, as observer bias can easily creep in, possibly skewing results. Additionally, the inability to control for external factors can challenge the interpretability of findings.
"The true value in quantitative methods lies not only in the numbers they yield but also in the systematic processes that guide research in understanding complex phenomena."
Mixed Methods Approach
The mixed methods approach has emerged as a vital strategy in data collection. By synthesizing both qualitative and quantitative data, researchers are able to gain a more comprehensive understanding of complex phenomena. This technique is important in this article as it highlights how diverse methodologies can be united to enhance the richness of the data collected.
In research, the need to dig deeper into the reasons behind numerical values—something that strictly quantitative methods often overlook—is what makes this approach significant. Thus, the mixed methods approach facilitates a fuller narrative, bridging the gap between numerical data and the human experience underlying that data.
Combining Qualitative and Quantitative Data
The Rationale Behind Mixed Methods
The rationale behind utilizing mixed methods is to leverage the strengths of both qualitative and quantitative approaches. Qualitative data can provide context, depth, and a nuanced understanding of social issues, while quantitative data can deliver broad insights through measurable and generalizable findings. This duality allows researchers to not only ask "what" is happening but also explore "why" it's happening.
A key characteristic of this rationale is its flexibility. Researchers can adapt their methods as needed and switch between qualitative and quantitative techniques depending on their research questions. This adaptability is beneficial because it allows for a more robust analysis, enabling findings to be corroborated and enhanced by using complementary data sources. One unique feature of employing mixed methods is triangulation, where multiple data sources validate the results. This can strengthen the credibility and reliability of the study, making it a popular choice in numerous fields.
Applications and Case Studies
Applications of mixed methods span various disciplines, particularly where complex social phenomena are being studied. For instance, a case study in education may combine standardized test scores (quantitative) with personal interviews of students and teachers (qualitative) to gauge the effectiveness of a new curriculum. This rich blend provides not just the outcome of the new curriculum but also insights into user experiences, potentially leading to data-driven improvements.
Another compelling application could be in health research, where the effectiveness of a health intervention may be assessed through patient recovery statistics while also incorporating qualitative interviews to understand patient perceptions and experiences. Such study designs make mixed methods appealing by enabling practitioners to tackle multifaceted issues.
However, challenges can arise as well. Mixing methodologies involves navigating differing paradigms, which can complicate analysis and interpretations. Yet, the advantages often outweigh these complexities, as the approach enriches the overall findings.
"Mixed methods research combines the strengths of both qualitative and quantitative approaches, allowing for a deeper understanding of research phenomena."
Emerging Technologies in Data Collection
In recent years, the landscape of data collection has undergone a significant transformation due to the rise of emerging technologies. These advancements not only broaden access to data but also enhance the efficiency and accuracy of the data collection process. Understanding these technologies is vital for researchers looking to optimize their methods and outcomes. With constant innovations, it is essential to stay informed about tools and approaches that can absolve traditional barriers and promote better, more informed research practices.
Mobile Data Collection Tools
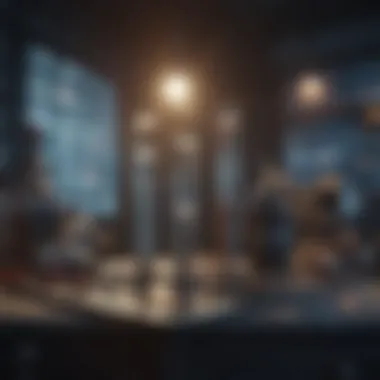
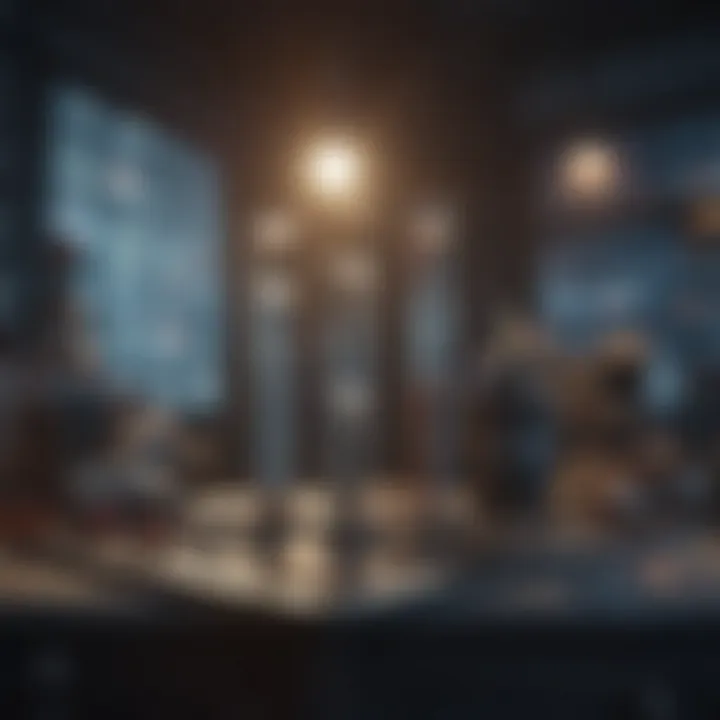
Mobile data collection tools offer a compelling alternative to conventional data gathering methods. These applications facilitate the collection of data via smartphones and tablets, merging convenience with efficiency. With just a few taps on a screen, researchers can design, distribute, and analyze surveys or forms.
The benefits of using mobile tools are manifold:
- Real-Time Data Entry: Respondents can input data instantly while on the go, reducing the lag time between data collection and analysis.
- Location Tracking: GPS features allow researchers to capture geospatial data, enriching the datasets with contextual location information.
- Offline Functionality: Many mobile apps permit data collection without an internet connection, syncing results once the connection is restored.
However, there are considerations to keep in mind, including the need for appropriate device compatibility and possible biases introduced by only capturing responses from users with smartphones.
Using Social Media as a Data Source
The social media landscape has burgeoned into a rich trove of user-generated content. Platforms like Facebook, Twitter, and Instagram not only serve as social spaces but also provide valuable data points for researchers. Harnessing social media data opens up avenues for understanding user behavior, trends, and sentiments.
Some advantages of tapping into social media include:
- Volume: With billions of users sharing content daily, there's an abundance of opinions, experiences, and behaviors to analyze.
- Diverse Demographics: The variety of users on social media allows researchers to target specific populations based on interests, locations, and demographics.
- Real-Time Insights: The dynamic nature of social media enables instant insights into emerging trends and public sentiments.
Yet, researchers must tread carefully. Ethical considerations surface around user consent and data privacy, as many users may not expect their publicly shared opinions to be used for research purposes.
Big Data Analytics
Big data analytics embodies the processing and analysis of vast datasets that traditional data processing software cannot handle effectively. This approach harnesses advanced algorithms to extract meaningful patterns and insights from a sea of complex data types. As the digital universe expands, the importance of big data analytics in data collection cannot be overstated.
Key benefits of utilizing big data analytics include:
- Predictive Analytics: By examining past trends, researchers can make forecasts about future occurrences.
- Enhanced Decision-Making: Data-driven insights empower organizations to make informed decisions and strategic moves.
- Automation of Routine Tasks: Automated data collection and processing reduces the workload and minimizes human error.
As helpful as it is, researchers must also contend with the challenges of data quality, security, and the ethical implications of using extensive datasets.
"Emerging technologies in data collection are not merely tools—they are transforming the foundation of research methodologies across disciplines."
Understanding and embracing these emerging technologies can enrich the data collection process, providing researchers with both the precision and scope needed for comprehensive analyses.
Ethical Considerations in Data Collection
When diving into the world of data collection, it’s pivotal to address the ethical aspects that come hand in hand with gathering information. Ethical considerations play a crucial role in ensuring that the integrity of the research process is maintained, protecting both the participants and the data collectors. This section will delve into the specific elements that define ethical data collection, the benefits it brings, and the key considerations that should always be top of mind.
Informed Consent and Transparency
Informed consent is not just a bureaucratic hurdle; it’s a fundamental principle that holds significant weight in ethical data collection. Simply put, informed consent means obtaining permission from participants after providing them with comprehensive information about the study. This includes the purpose of the research, how their data will be used, and any potential risks involved. Transparency is about being upfront with participants; it's like not pulling a fast one on them. For instance, if you're collecting data for a marketing study, it’s essential to let the participants know how their information will influence product development or advertising.
The benefits of informed consent extend beyond mere legality. It fosters trust between the researcher and the participant, enhancing the quality of the data collected. When participants feel valued and aware of what they're getting into, they're more likely to provide authentic and detailed responses.
Additionally, there’s a growing emphasis on ethical standards as scrutiny increases in the vast landscape of data collection. This is particularly relevant in light of recent debates surrounding data privacy and the misuse of information. Research institutions often implement strict guidelines, ensuring that informed consent becomes not just a signature on paper but a pivotal part of the process.
To sum up, obtaining informed consent is not just a checkbox to tick off. It’s the backbone of ethical data collection—ensuring participants are rightly informed and respected.
Maintaining Confidentiality
Confidentiality in data collection is another cornerstone of ethical practice. It involves safeguarding the privacy of participants and ensuring their personal information is not disclosed without permission. When individuals share sensitive data, they naturally expect their identities to be protected. Think of it this way: if someone shares a personal story, they wouldn’t want that out in the public domain, right?
Maintaining confidentiality comes with numerous considerations for researchers. It’s not merely about avoiding the public sharing of names or personal identifiers; it also involves how data is stored, processed, and ultimately shared. For example, using encryption methods and secure databases for storing participant information is essential. Researchers should also limit access to the data, sharing it only with those who have a legitimate need to know.
Furthermore, confidentiality extends to how results are reported. Aggregating data or anonymizing information are common practices used to protect individual identities. In this way, while one can draw conclusions from the data, the individuals behind those data points remain safeguarded.
"Ethical considerations in data collection are not just policies; they are commitments to respect and human dignity."
As we navigate the complexities of data collection, understanding and implementing ethical considerations is indispensable. By focusing on informed consent and maintaining confidentiality, researchers can create an environment of trust, which is essential for collecting quality data.
Challenges in Data Collection
In the landscape of research, the road to gathering data is paved with considerable hurdles. These challenges not only complicate the logistics of conducting studies but also directly influence the accuracy and integrity of the findings made. Understanding these challenges is critical for students, researchers, educators, and professionals aiming to refine their methodologies.
When embarking on a data collection journey, researchers must navigate a maze of potential pitfalls that can skew results, waste resources, or even lead to ethical dilemmas.
One key benefit of thoroughly examining these challenges is that it allows researchers to preemptively strategize appropriate solutions.
Common Obstacles Encountered
There are various obstacles that can hinder the data collection process. Here are notable examples:
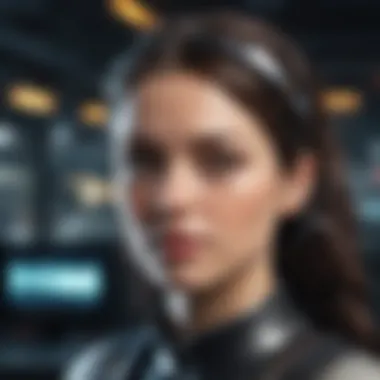
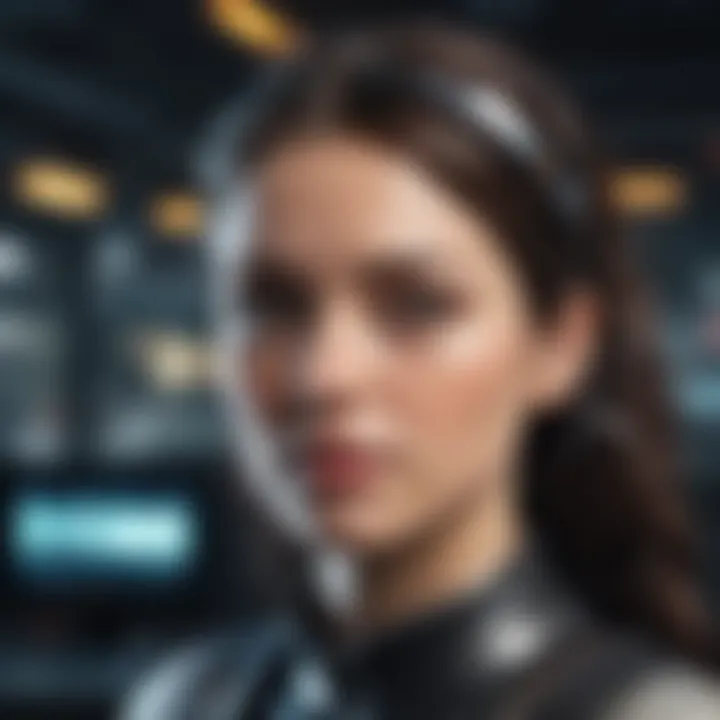
- Access to Participants: In certain fields, especially those involving sensitive topics, getting access to participants may prove difficult. Many individuals might be hesitant to share their experiences or opinions. This reluctance can severely limit the scope of data collected.
- Misalignment of Tools: Utilizing outdated or poorly designed tools can substantially compromise the quality of data. For instance, surveys may fail to collect useful information if the questions are vague or confusing.
- Resource Constraints: Time and financial limitations can severely affect data collection efforts. Researchers may find themselves having to conduct a smaller study than initially intended due to insufficient funds or time constraints.
- Exquisite Complications of Contextual Differences: Socio-cultural differences can create significant barriers, especially in ethnographic studies. What's acceptable in one culture might be a huge faux pas in another, which can lead to misunderstandings and bias in the data collected.
Addressing these obstacles requires careful planning and consideration of multiple factors, including ethical guidelines and methodological rigor.
Addressing Bias in Data Collection
Bias during data collection poses a considerable threat to the validity of research findings. Even the most well-intentioned research can fall prey to it, leading to skewed or misleading conclusions. Here’s how researchers can tackle this issue:
- Recognizing Bias Sources: The first step is identifying where bias might creep in. This could be in the design of surveys, the selection of participants, or even in the interpretation of collected data.
- Utilizing Random Sampling: One effective method to minimize selection bias is using random sampling. This method ensures every individual in the target population has an equal chance of being selected, leading to a more representative dataset.
- Employing Blinded Protocols: In experiments, blinding participants (and sometimes researchers) to certain aspects can reduce expectancy biases that may influence the results.
- Triangulation: Using multiple data sources or different methodologies can help counter potential biases inherent in any one method. For example, combining qualitative interviews with quantitative surveys allows for a more holistic understanding of research questions.
- Continuous Training: Regular training on recognizing and confronting biases can empower researchers to maintain objectivity, particularly in qualitative studies. This aspect is often overlooked, yet it’s essential to fostering a culture of ethical and responsible research.
"Recognizing and addressing biases is not merely an academic exercise; it's a vital component that can determine the fate of research."
Evaluating Data Collection Methods
In the realm of research, determining which data collection method to use can feel like trying to find a needle in a haystack. That’s where evaluating data collection methods becomes crucial. This stage allows researchers to sift through options and find the most suitable ones for their projects. Each method comes with its own set of pros and cons that can significantly impact the outcome of the research. Having a structured way to evaluate these techniques enables researchers to make informed choices that enhance the quality of their findings and contributes to the credibility of their work.
Criteria for Method Evaluation
When evaluating data collection methods, three core criteria often come into play: reliability, validity, and feasibility. Each of these components has its own nuances that warrant consideration.
Reliability
Reliability pertains to the consistency of a measurement. A reliable method will yield the same results under similar conditions, which is vital for ensuring that the data reflects true patterns and not mere fluctuations. For instance, in a survey that asks about consumer habits, if responses consistently align with observable behaviors, then the survey holds merit.
- Key Characteristic: The primary trait of reliability is repeatability. If a week later, the same survey yields the same results, the method can be deemed reliable.
- Benefit: This consistency makes reliability an attractive choice for researchers aiming to establish trust in their data. In this article, focusing on reliability assures readers that conclusions drawn from studies are firmly based on solid evidence.
- Unique Feature: A distinctive feature of reliability is its correlation to sample size; larger samples generally provide more reliable data. However, one must also be wary: a method can be reliable yet not valid. For instance, if a survey always outputs similar results but is based on faulty premises, it’s still unreliable in its essence.
Validity
Validity measures whether a method accurately captures the concept that it’s intended to assess. In other words, it’s about measuring what you mean to measure. For example, if a questionnaire designed to evaluate mental health reflects participants' true psychological state, it is seen as valid.
- Key Characteristic: The hallmark of validity is accuracy. If a measure is valid, it truly reflects the variable it is intended to quantify.
- Benefit: Validity is perhaps the most cherished attribute for researchers aiming for depth and authenticity in their findings. It assures the audience that results genuinely represent the inquiry's aim in this article.
- Unique Feature: A method can possess high validity even in smaller sample sizes, as what counts is the content being measured, not the number of participants. However, too much emphasis on validity can sometimes cakewalk one into overcomplicating methods unnecessarily.
Feasibility
Feasibility refers to the practicality of executing a given data collection method. This encompasses various aspects such as time, resources, and accessibility. A method may be reliable and valid, but if it ’s impractical to implement, it’s not worth pursuing.
- Key Characteristic: The essence of feasibility lies in practicality. A feasible study can feasibly be carried out with available resources, time frame, and scope.
- Benefit: This makes feasibility a pragmatic choice in the framework of this article, especially for researchers working within constraints. Understanding feasibility can save significant time, effort, and frustration down the line.
- Unique Feature: A unique aspect of feasibility is its adaptability; methods can often be tailored to better fit constraints. However, one should be cautious to not sacrifice essential qualities such as validity and reliability for mere convenience.
Best Practices for Selecting Methods
Selecting the right methods for data collection is no small feat. It’s essential to approach this with careful consideration of the previously mentioned criteria. Here are some insights for making this process smoother:
- Identify Research Goals: Different goals will dictate different methods. Start by clarifying what the research aims to achieve, whether it be exploratory, explanatory, or descriptive in nature.
- Consider Mixed Methods: Sometimes a combination of qualitative and quantitative techniques yields the best results. Evaluating methods through diverse lenses can result in a well-rounded viewpoint of the research subject.
- Pilot Testing: Before committing to a method, conduct a pilot test. This way, researchers can gauge not only reliability and validity but also the logistical side of feasibility.
- Analyze Existing Literature: Examining what has previously worked in similar studies can provide clues about which methods might be more effective.
Remember: Evaluating data collection methods is not just a step in the research process, but a critical foundation that can make or break scholarly inquiries. Upholding standards of reliability, validity, and feasibility ensures that the research is robust and insightful.
Ending and Future Directions
Data collection constitutes a vital aspect of research that informs our understanding across various fields. In the grand scheme of scientific inquiry, this article serves to highlight not just the existing methodologies, but also the necessity for their continued evolution. As we navigate through a world saturated with information, grasping the significance of reliable data collection methods becomes paramount. Effectively gathering data impacts the quality of research outcomes, which, in turn, influences policy decisions, educational strategies, and even social change.
The path forward reveals the importance of adaptability in data collection practices. Researchers must continuously assess the relevance and efficiency of methods used. With new technologies surfacing almost daily, juggling between traditional techniques and innovative approaches can seem like a daunting task. However, doing so ensures that the resultant insights are both valid and applicable in real-world scenarios.
Benefits of Refining Data Collection Techniques:
- Enhanced Validity: By staying current with methods, researchers reduce biases and inaccuracies.
- Broader Reach: The integration of new technologies allows for more diverse data sources, leading to richer datasets.
- Time Efficiency: Adapted processes save researchers’ time, allowing for quicker turnarounds on data analysis.
- Informed Decision-Making: Ongoing evaluation of data methods contributes to better-informed conclusions, fostering trust among research communities and stakeholders.
As considerations around ethics continue to shift, the importance of transparency and integrity in data collection cannot be overstated. The demand for data privacy, informed consent, and ethical diligence grows stronger, urging researchers to cultivate practices that reflect these values.
Moving forward, we also bear witness to several exciting innovations in data collection that promise to refine and enhance our understanding of varied phenomena.
Summary of Key Points
To sum it up, the discussion around data collection emphasizes many channels of thought:
- The diversity of methods available is a strength but comes with its own set of challenges.
- Continual assessment of effectiveness and relevance within various contexts is vital for reliable research.
- Ethical considerations are no longer an afterthought; they inform every angle of research design.
- The integration of innovative technologies can revolutionize traditional paradigms, enriching the field considerably.
Innovations on the Horizon
With technology ever advancing, researchers have an immediate horizon filled with promising new tools and methodologies. For instance, artificial intelligence is now being leveraged to analyze large datasets faster than ever. Think about machine learning models that predict outcomes based on complex patterns hidden in mounds of data.
Other notable innovations include:
- Wearable Technology: Devices such as smartwatches collect personal health data, delivering real-time insights that can be extremely valuable for both qualitative and quantitative assessments.
- Remote Sensing: In environmental studies, satellites and drones provide access to data that was once logistically impossible to obtain, expanding the scope for ecological research.
- Blockchain Technology: Perhaps more unconventional, the security and transparency offered by blockchain could revolutionize how data integrity is maintained, especially in fields reliant on rigorous standards.
As we push forward, it's crucial to remain mindful that these devices and methods aren't just tools, but gateways to deeper understanding. The future of data collection lies not only in leveraging innovations but also ensuring that as we gather data, we do so ethically, inclusively, and thoughtfully.