In-Depth Exploration of Facial Emotion Datasets
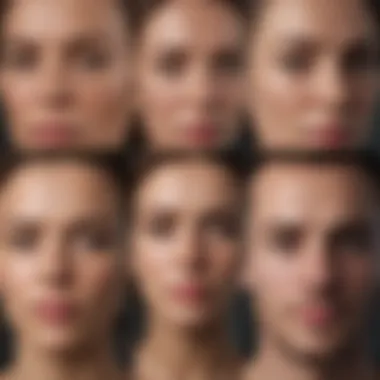
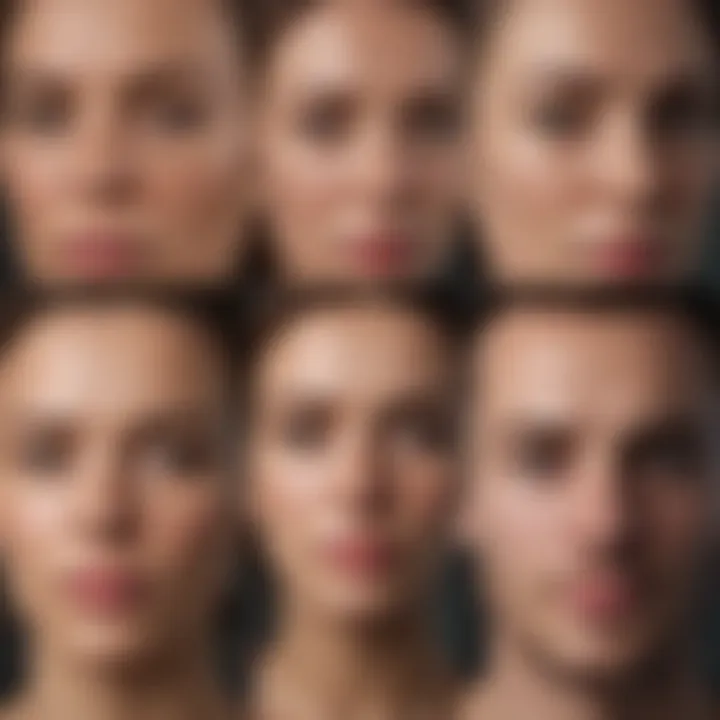
Intro
Understanding human emotions is a complex task, often riddled with nuances that challenge even the keenest observers. Emotions shape our interactions, decisions, and overall experiences. In recent years, the advent of facial emotion datasets has opened a new frontier in understanding these human expressions. These datasets serve as a backbone for various fields, from psychology to artificial intelligence, paving the way for significant breakthroughs.
In this article, we will explore the development, significance, and various applications of facial emotion datasets. We'll shine a light on the methodologies used for data collection, discuss common challenges encountered in maintaining data integrity, and examine the ethical implications that arise when working with such sensitive information. The goal is to provide a comprehensive overview that not only showcases the current landscape but also offers insights into future trends. Through the intertwining of research and application, we aim to underscore why these datasets are cornerstone resources for researchers, educators, and industry professionals alike.
Prolusion to Facial Emotion Datasets
The landscape of facial emotion datasets has seen significant transformation over the years, proving to be indispensable to the fields of psychology, artificial intelligence, and beyond. These datasets essentially act as the bedrock for our understanding of human emotions, capturing their complexity through various types of facial expressions. Their importance lies in the ability to bridge the gap between raw emotion and quantitative analysis, allowing researchers to decode how feelings manifest through facial features.
Definition and Importance
Facial emotion datasets are collections of images or videos that depict various human emotions conveyed through facial expressions. These can include happiness, sadness, anger, surprise, fear, and disgust, among others. Understanding these emotions is critical not only for academic research but also for practical applications. For instance, in psychological settings, these datasets enable the accurate assessment of patients’ emotional states, thus aiding in diagnosing conditions like depression or anxiety.
In the realm of artificial intelligence, particularly in the development of machine learning algorithms, these datasets serve as training material. By equipping AIs to recognize and interpret human emotions, we open doors to innovations in areas like human-computer interaction, marketing strategies, and security systems. The role of facial emotion datasets extends beyond mere academic interest; they hold the potential to reshape industries fundamentally.
Key Benefits:
- Enables emotion recognition studies in psychology.
- Fuels advancements in AI and machine learning.
- Enhances user experience in technology applications.
- Supports mental health monitoring and assessment.
Historical Context
The origins of facial emotion datasets can be traced back to the early studies of emotional expression, notably those conducted by psychologists like Paul Ekman. In the 1970s, Ekman's groundbreaking research on facial expressions led to the development of the Facial Action Coding System (FACS). It was one of the first structured ways to categorize facial movements by the muscles involved. As our understanding of emotions evolved, so did the methodologies used to collect and analyze these expressions.
In the following decades, advancements in technology, including the rise of digital imaging and video recording, allowed for more comprehensive data collection. The introduction of online platforms and social media further expanded the reach and accessibility of these datasets. Researchers could now gather diverse emotional expressions from a wide range of demographics, enriching the datasets and making them more valuable for cross-cultural analyses.
The evolution of facial emotion datasets has not been without its challenges. Issues related to bias, representation, and ethical implications surfaced as these datasets grew. However, acknowledging these obstacles has led to ongoing discussions and efforts aimed at improving dataset quality and ensuring that they reflect real-world diversity.
"The value of facial emotion datasets lies in their capacity to illuminate the often unseen facets of human interaction and behavior."
Understanding the intricacies of facial emotion datasets sets the stage for deeper dives into their methodologies, key datasets, applications, challenges, and future perspectives. Hence, as we explore this topic further, we delve into the specifics of how these datasets are compiled, their historical developments, and their pivotal role in enhancing our grasp of human emotions.
Methodologies for Data Collection
The methodologies applied in collecting facial emotion datasets are paramount to the effectiveness of emotion recognition systems. These techniques are vital in establishing reliable avenues for data acquisition, ensuring that the information captured reflects accurate emotional states. Without robust data collection methods, the subsequent analyses lose credibility, impacting both research outcomes and the practical applications of these datasets across various fields. In this section, we will delve into different data acquisition techniques and the standardization of data, key components that contribute to the integrity and usefulness of emotion datasets.
Data Acquisition Techniques
Manual Annotation
Manual annotation involves the painstaking process where human annotators review and label images or videos, indicating various emotional expressions. This approach allows for a nuanced understanding of emotions since annotators can consider context and subtleties in facial gestures that automated systems might overlook. A key characteristic of manual annotation is its depth of perception, which is often regarded as essential for accurate emotion classification.
One compelling advantage of manual annotation is the opportunity to provide fine-grained details. For instance, when a subtle smirk indicates amusement or sarcasm, human judgments can differentiate these emotions with a level of sophistication that machines currently struggle with. However, this method isn't without drawbacks. It's time-consuming and can introduce variability based on the individual annotator's biases or interpretations. Often, inconsistencies in labeling arise, which can muddle dataset reliability.
Automated Systems
Automated systems utilize advanced algorithms and machine learning models to detect and classify emotions from facial expressions. This methodology often includes facial recognition technologies that analyze spatial patterns and movement dynamics. An essential characteristic of automated systems is their efficiency; they can process vast amounts of data in a fraction of the time it would take human annotators.
The unique feature of these systems is their ability to learn iteratively through deep learning techniques, improving their accuracy over time. However, they also bring notable disadvantages. One major concern is that they might misinterpret nuanced human expressions. For instance, they tend to struggle with emotions not clearly defined in classic categories, such as mixed feelings or culturally specific expressions. Furthermore, data quality can vary significantly based on the input images’ diversity.
Crowdsourcing Approaches
Crowdsourcing approaches harness the power of the online community to gather data from a broader audience. This method encourages contributors to upload images or videos where they express various emotions, which are then aggregated into a dataset. A significant characteristic of this approach is its scalability; it can rapidly increase the volume of data collected, providing diverse emotional representations across different demographics.
A key unique feature here is the ability to capture real-time emotional responses from various contexts. This can lead to richer datasets that represent a myriad of experiences. However, the downsides include potential inconsistencies in quality since not all submissions meet the same standards. Moreover, relying on non-experts means that annotations can vary widely, which can diminish the dataset's reliability.
Standardization of Data
Ensuring that data is standardized is crucial for the integrity of facial emotion datasets. Standardization helps maintain uniformity across datasets, paving the way for effective analyses and comparisons. Here we discuss two key aspects: quality of images and annotation consistency.
Image Quality Considerations
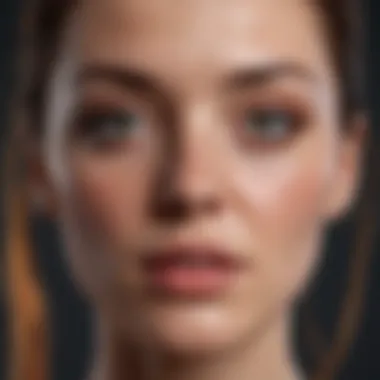
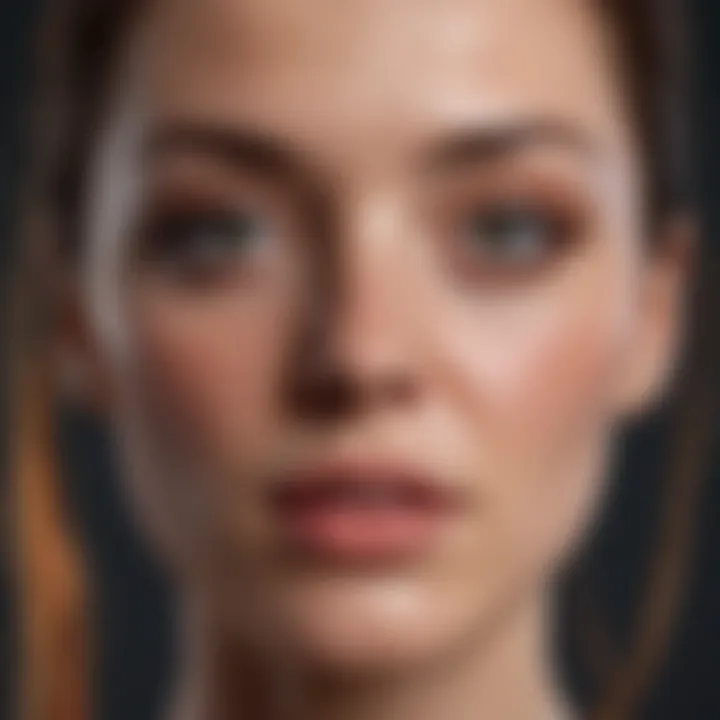
Image quality considerations pertain to the clarity and resolution of the captured images within a dataset. High-quality images are crucial for enabling facial recognition systems to accurately detect subtle emotional cues. A defining characteristic of this aspect is ensuring that images have adequate lighting, focus, and resolution to avoid misinterpretations of expressions.
When datasets are properly curated for image quality, they enhance the reliability of the recognition algorithms. However, there’s a balance to strike; higher quality images might demand more storage and processing power, which can complicate large-scale analyses. Notably, poorer quality images can lead to false positives or negatives, compromising the dataset's overall effectiveness.
Annotation Consistency
Annotation consistency is about maintaining similar standards across different annotations. It involves establishing clear guidelines for what constitutes certain emotions and ensuring all annotators follow these standards closely. A fundamental characteristic of annotation consistency is its role in reducing variability across labels, which is vital for data integrity and comparability across analyses.
Having a consistent annotation process makes it easier to replicate studies and validate research findings. However, achieving this consistency can be challenging. Different cultural backgrounds and subjective interpretations can lead to differing opinions on what certain expressions signify. This can introduce further complications, especially in datasets aiming for broad applicability or those intended for global applications.
Effective data collection methodologies not only ensure the quality of facial emotion datasets but also enhance their applicability in real-world scenarios.
Key Datasets in Facial Emotion Recognition
Facial emotion recognition is an intricate and pivotal area within emotional analysis, and the key datasets driving this research are foundational to understanding human emotions. These datasets serve as the backbone for various applications in psychology, artificial intelligence, and even healthcare, providing rich sources of information. A comprehensive grasp of these datasets allows researchers and practitioners to build better models, analyze emotional cues more accurately, and contribute to advancements in both theoretical frameworks and technological innovations.
The AffectNet Dataset
Overview
The AffectNet dataset is a substantial resource boasting over a million facial images labeled for seven different emotions. This dataset holds a significant position due to its depth and scalability. What sets AffectNet apart is its large variety of images sourced from different ethnic backgrounds, which facilitates a more inclusive study of emotions. Moreover, the dataset supports both automated and manual annotations, making it a versatile choice for various research implementations.
Features
One of the distinct features of the AffectNet dataset is its expansive image annotations that include not only basic emotions but also nuanced ones like contempt and pride. This comprehensive categorization supports more granular analyses which are crucial in areas like social behavior studies. This granularity can produce richer insights, although it might also lead to complexity in training machine learning models effectively.
Applications
AffectNet is widely recognized in academic circles for its ability to enhance emotion recognition algorithms. The dataset is increasingly utilized in emotion-aware applications, including customer feedback systems and affective computing. However, researchers should tread carefully, as the dataset's size and complexity may result in longer processing times or necessitate sophisticated preprocessing methods.
FER-2013
Dataset Composition
FER-2013, another notable dataset, comprises about 35,000 facial images, with a focus on providing a benchmark for emotion recognition technology. This dataset mainly encapsulates basic emotions such as happiness, sadness, fear, anger, surprise, and disgust. What stands out about this dataset is its balance between simplicity and usability. Its compact size relative to the amount of data often analyzed makes it an attractive option, especially in initial research phases. Nevertheless, with its straightforward composition, it might not capture the full spectrum of human emotions well enough for more advanced analyses.
Challenges and Limitations
While FER-2013 is widely used, it isn't without challenges. The dataset's somewhat limited range can lead to difficulties in creating models robust against real-world emotional expressions. Furthermore, the images often lack diversity concerning ethnicity and background scenarios, providing a less comprehensive view of emotions across different populations. This limitation underscores the importance of supplementing this dataset with others like AffectNet for a more rounded perspective.
CK+ Dataset
Details on the Dataset
The CK+ Dataset, or the Extended Cohn-Kanade Dataset, has contributed significantly to facial expression recognition research since its inception. It contains 593 sequences from 123 subjects, capturing spontaneous facial expressions in a controlled environment. One key feature is the detailed action unit annotation, which allows for a deep dive into facial dynamics. This has proven advantageous for researchers seeking to analyze subtle emotional expressions.
Impact on Research
The CK+ dataset has set a high standard in emotional recognition studies. Its rich annotation scheme and controlled environment have made it a benchmark for evaluating new emotion recognition algorithms. However, researchers may find that its artificial setting could limit the applicability of findings to real-world scenarios, as naturalistic perceptions of emotions can differ significantly from those portrayed in such controlled experimental settings. Therefore, blending datasets is often proposed to balance these limitations.
Applications of Facial Emotion Datasets
The application of facial emotion datasets is vast and multifaceted, ranging from psychological research to groundbreaking technological advances. Understanding the versatility and significance of these datasets is crucial in contextualizing their value. When researchers and developers look at these datasets, they see tools that can decode the intricate language of human emotions, thereby unlocking potential in various fields. Each application, whether it be in psychology, artificial intelligence, or healthcare, carries its unique set of benefits, considerations, and challenges.
Psychological Research
Emotion Recognition Studies
Emotion recognition studies harness the power of facial emotion datasets to decode what an individual's expressions signify. This is a core aspect, especially for understanding human emotions at various levels. These studies not only help in identifying baseline emotions but also contribute toward recognizing subtle emotional variations that occur in different contexts. A significant characteristic of emotion recognition studies is their reliance on machine learning techniques to classify and interpret the emotions displayed. This methodology is favored in this article because it brings a scientific edge to otherwise subjective interpretations, establishing a bridge between emotion and data.
One unique feature of these studies is the adaptability of different algorithms, which allow them to refine their recognition capabilities based on previous experiences. However, one notable limitation is the context dependency of facial expressions; what appears as joy in one culture could signify something entirely different in another. This variability means researchers must tread carefully, considering cultural implications to avoid misunderstandings in emotion analysis.
Behavior Analysis
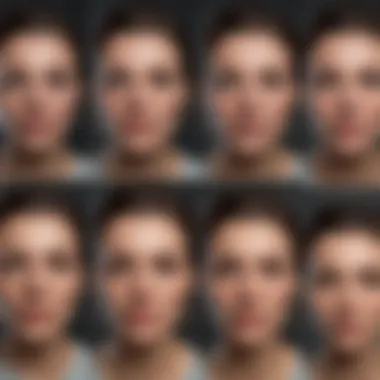
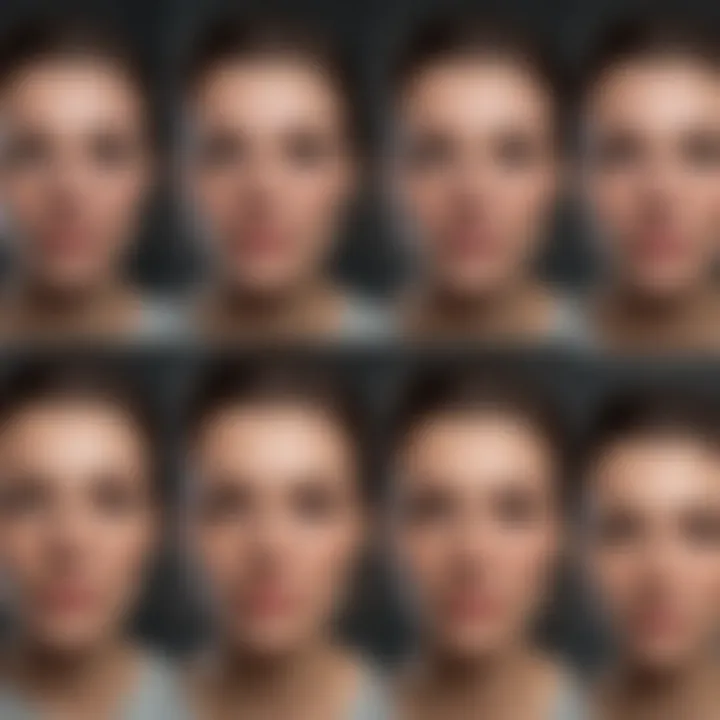
Behavior analysis takes a more expansive view by integrating facial emotion datasets to observe and interpret patterns in behaviors derived from emotional responses. This field plays a vital role in shedding light on how emotions influence actions and decisions. The significance of behavior analysis lies in its ability to inform psychological theories and practices. It provides concrete data that correlates facial expressions with behavioral outcomes. This makes it stand out as a valuable avenue in our discussion.
A particularly advantageous quality of behavior analysis is its holistic approach, which examines not just isolated facial expressions, but also the broader implications of emotions on behavior in varied situations. The downside, however, is its complexity. It requires not only sophisticated datasets but also nuanced understanding and interpretation abilities, demanding careful consideration during research.
Artificial Intelligence Development
Machine Learning Algorithms
Machine learning algorithms are at the heart of interpreting facial emotion datasets. These algorithms can extract features and patterns from raw image data, translating visual information into actionable insights. Their importance in this article cannot be overstated, as they represent the backbone of emotion recognition systems and facilitate advancements in AI. The key characteristic that makes these algorithms prominent is their ability to learn from data, improving their functions over time yes, it's a continuously evolving field!
One unique feature of machine learning algorithms is their capacity for real-time processing. This means they can analyze emotions as they occur, making them highly relevant in various applications from customer service chatbots to mental health assessments. However, challenges like the need for substantial training data to reduce biases and enhance accuracy can hinder their effectiveness.
Real-world Implementations
The real-world implementations of facial emotion datasets are where theoretical learning takes on practical significance. From enhancing user experiences in technology to aiding mental health assessments, these implementations showcase the practical side of research. The critical aspect of these applications is that they translate academic theories into tangible solutions that address real-world problems.
What sets these implementations apart is their diverse range, from academic institutions employing emotional analytics to better cater to student needs, to corporations using data to enhance customer interactions. While the benefits are apparent, such as increased efficiency and personalization, there are also downsides, including the risk of misinterpretation or oversimplification of emotional data, which necessitates careful monitoring and continuous improvement.
Healthcare Applications
Mental Health Assessment
In the realm of healthcare, mental health assessment has become an essential area where facial emotion datasets can shine. These datasets allow professionals to analyze emotional expressions and correlate them with psychological states. The great advantage of focusing on mental health assessment lies in its potential to provide early indications of emotional disturbances, leading to timely interventions. This article rightly emphasizes this aspect, considering its implications for public health.
A unique feature of mental health assessments using facial emotion data is the prospect of remote analysis. This can be a game-changer in terms of accessibility for patients who may not always be able to attend traditional therapy sessions due to various barriers. However, a challenge lies in ensuring data privacy and ethical usage, as mismanagement of sensitive emotional data could prove detrimental.
Patient Monitoring Systems
Patient monitoring systems leverage facial emotion datasets for more efficient tracking of emotional responses in patients. This is particularly beneficial in managing cases of chronic illnesses or severe mental health conditions, where emotional state can fluctuate significantly. The uniqueness of these systems lies in their ability to provide real-time updates to healthcare providers, prompting timely responses when patients exhibit distress.
Despite their benefits, significant considerations regarding implementation exist, particularly surrounding the balance between monitoring and patient autonomy. The goal is to enhance care without crossing boundaries or infringing on privacy rights. Acknowledging these nuanced dynamics is key to successfully integrating emotive data into patient monitoring frameworks.
Overall, the applications of facial emotion datasets present a wealth of opportunities for research and practical use. Understanding the complexities and subtleties involved is crucial for maximizing the benefits of these datasets while safeguarding ethical practices in all fields.
Challenges in Dataset Utilization
When it comes to facial emotion datasets, the challenges faced in ensuring their effective use cannot be overstated. Understanding these challenges is crucial for researchers and practitioners alike. As facial emotion datasets proliferate, ensuring that they are utilized appropriately becomes more imperative. This section explores the intricate web of issues that can arise, particularly focusing on bias and representation, as well as the ethical considerations surrounding data privacy.
Bias and Representation Issues
Demographic Representation
Demographic representation is at the heart of the issue when we talk about bias in facial emotion datasets. A key characteristic of this aspect is that datasets often fail to capture a diverse range of human faces. This shortcoming can lead to algorithms that are ill-equipped to recognize the emotions of underrepresented groups. To highlight the necessity, take for instance a study that relied on predominantly young, Caucasian subjects. Such a lack of variety in faces translates into algorithms that might misinterpret or even overlook emotions expressed by other demographics.
Moreover, biased datasets can yield outcomes that perpetuate stereotypes. This can be especially detrimental in sensitive fields such as mental health and law enforcement, where misinterpretations can have severe consequences. Therefore, ensuring diverse demographic representation is not just beneficial; it is fundamentally necessary for the reliability and accuracy of emotion detection technologies.
Scaling Datasets
Scaling datasets presents its own set of challenges. As facial emotion datasets grow in size, the complexity involved in managing and processing this data significantly heightens. A primary characteristic of scaling is the need for robust infrastructure, which can become quite costly.
For example, larger datasets typically require more sophisticated machine learning setups and processing power. This might lead smaller research teams to be left in the dust, unable to keep pace with advancements due to resource constraints. On the flip side, expansive datasets can lead to better training for models, resulting in improved recognition abilities. However, without careful handling, scaling can also amplify any existing biases and discrepancies found in a smaller dataset. Thus, while there are clear advantages to scaling, one must tread carefully to navigate the associated risks and ethical considerations.
Data Privacy and Ethics
Informed Consent
Delving into data privacy, informed consent stands out as a significant aspect. It essentially refers to participants being fully aware of how their emotional data will be used. This element is crucial for ethical compliance and has implications for data reliability. A key characteristic is the transparency that informed consent necessitates. Participants should know the potential risks involved in sharing their emotional data.
However, obtaining informed consent is largely dependent on the context of data collection. In research environments, it is a standard procedure, yet, in more informal settings, it can fall by the wayside. This can lead to situations where participants are unaware of how their data is being utilized, raising ethical red flags. Fostering a culture of informed consent not only secures ethical standing but enhances the credibility of research findings, making it an essential practice in dataset utilization.
Data Handling Practices
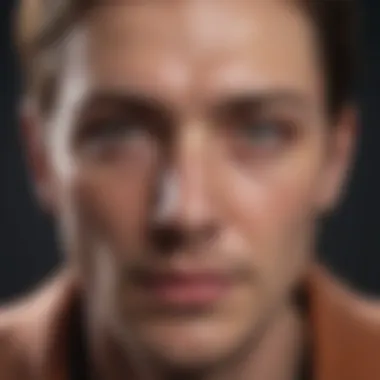
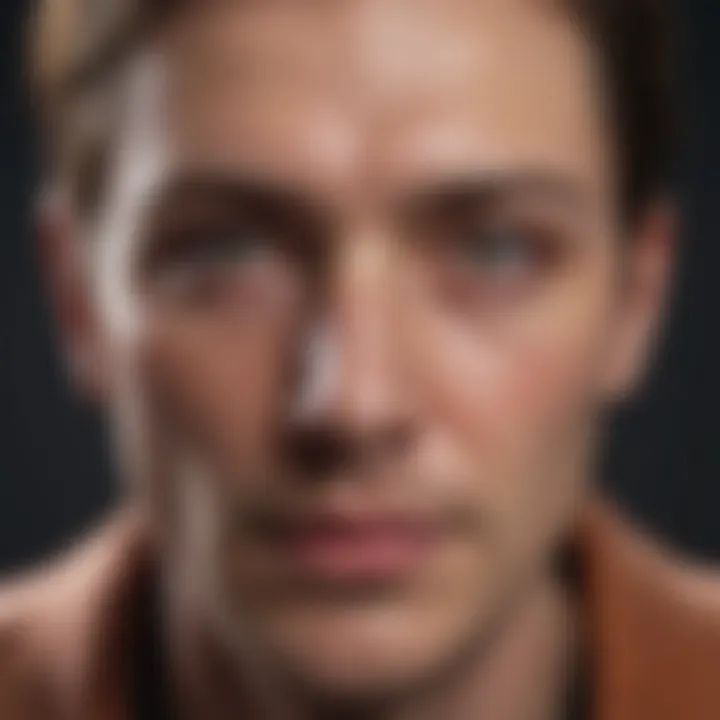
In tandem with informed consent, data handling practices play a pivotal role in maintaining ethical standards. This component focuses on how data is stored, processed, and shared once collected. A big part of good data handling practices is ensuring data anonymization to protect participants' identities.
Proper data handling can contribute significantly to the overall goal of ethical compliance in emotion research. Notably, when datasets are handled poorly, the risk of misuse increases. Hackers could access sensitive emotional data, leading to dire consequences. Plus, if data sharing is not adequately managed, it can inadvertently lead to bias re-introduced during future analyses. While ensuring robust data handling practices can be meticulous work, they are vital for maintaining ethical integrity and trust in the research community.
"The essence of ethical research lies in the proper handling and respect for participant data, establishing a bedrock of trust in facial emotion studies."
In summary, navigating the challenges of dataset utilization in facial emotion recognition engages with a maze of complexities. From ensuring diverse demographic representation to addressing data privacy and the obtaining of informed consent, every step outlines the need for careful and considerate approaches in this evolving field.
Future Trends in Facial Emotion Dataset Research
The landscape of facial emotion datasets is evolving quickly, driven by significant technological enhancements and interdisciplinary collaboration. This section delves into the oncoming trends that are shaping the research environment, examining how advancements in AI and analytical tools are being utilized and the implications of cross-field studies for the future of emotional recognition.
Technological Advancements
AI and Deep Learning
AI and deep learning technologies are leaving a significant mark on the field of facial emotion recognition. These tools offer sophisticated algorithms that analyze vast datasets, enabling the extraction of hidden patterns in emotional expression. A hallmark of deep learning is its capacity to process unstructured data with remarkable efficiency, which is particularly beneficial when handling the complexities of human emotions that often elude traditional analysis methods.
One key characteristic of deep learning is its hierarchical model architecture. This structure allows the system to learn features at multiple abstraction levels. The ability to automatically learn from raw data, rather than relying on manual feature extraction, stands out as a game-changer in this field. However, it’s important to weigh these advantages against the challenges inherent in deep learning. Data-intensive requirements and the risk of overfitting are two common hurdles that researchers must navigate. Despite these issues, the allure of improved performance almost ensures that AI and deep learning will remain central to future developments in facial emotion datasets.
Emerging Analytical Tools
Emerging analytical tools are also transforming how researchers approach facial emotion datasets. These tools span from novel data visualization methods to advanced sentiment analysis software. A defining feature of these new tools is their capacity to provide insights in real-time, allowing for dynamic interaction with data.
What makes these tools particularly attractive is their versatility. They can often be integrated with existing datasets, enhancing the research framework without requiring an overhaul. For instance, tools that offer predictive analytics can forecast emotional trends based on historical data, providing critical insights for various applications from healthcare to market research. The challenge, however, lies in ensuring these tools maintain ethical standards and promote transparency with data use. Addressing these concerns is vital to the integrity of the research output, motivating developers to prioritize ethical considerations alongside technological progress.
Interdisciplinary Collaborations
Cross-Field Studies
Cross-field studies represent a promising avenue in the exploration of facial emotion datasets. By merging insights from psychology, neuroscience, and computer science, these studies strive for a holistic understanding of emotional expression. A standout feature of such interdisciplinary research is its capacity to yield richer datasets that capture the breadth of human emotional experience.
Furthermore, this collaboration paves the way for innovative methodologies that may not emerge in isolated research environments. For instance, a psychological perspective can inform better algorithmic designs, ensuring that the computational models are more grounded in human emotional cognition. On the downside, maintaining effective collaboration across diverse fields can be challenging due to differing terminologies and objectives, but the effort often results in breakthroughs that advance the field significantly.
Integrative Approaches
Integrative approaches take the concept of collaboration a step further, advocating for a more seamless integration of theories and methods from various disciplines. Such integration can lead to the development of comprehensive frameworks that address both technical and socio-emotional aspects of facial emotion datasets. A key characteristic of integrative approaches is that they often foster innovation and creativity, allowing researchers to tackle complex questions from multiple angles.
The unique advantage here lies in the establishment of nuanced perspectives that consider the social, cultural, and psychological contexts of emotions. However, while these approaches offer a more thorough exploration of emotions, they may also lead to complexity in analysis and interpretation. As researchers juggle multiple theoretical frameworks, ensuring clarity and focus can become a challenging feat.
The future of facial emotion datasets rests on the convergence of technological advancements and interdisciplinary insights, creating a fertile ground for innovative research and applications.
Culmination
Ending any comprehensive exploration often sheds light on the nuances that may easily slip through the cracks. In discussing facial emotion datasets, it becomes clear that their significance transcends mere data collection. They are the backbone for numerous advancements in fields such as psychology, artificial intelligence, and even healthcare. One of the most vital aspects featured in this article is the intricate intersection between technology and human emotion detection. As we unravel this connection, it highlights how these datasets serve as a bridge that links theoretical knowledge with practical applications.
Understanding this topic provides numerous benefits. For instance, researchers developing technologies that interpret facial emotions can refine their designs based on rich datasets, ultimately leading to tools that better understand complex emotional states. Thus, the datasets not only enhance academic research but also inform real-world applications through machine learning and social robotics.
Moreover, ethical considerations surrounding these datasets merit serious thought. As highlighted in earlier sections, data privacy, informed consent, and representation challenges cannot be overlooked. Each dataset carries with it a promise that researchers must uphold. Engaging with facial emotion datasets goes beyond technical applications; it opens up discussions on morality and human rights, compelling scientists and practitioners to consider the potential impacts of their work on society at large.
"By recognizing the profound implications of facial expression data, we allow ourselves to shape a future where technology is empathetic and adapts to human nuances."
By focusing on the essential elements discussed throughout this article, we can appreciate the multifaceted nature of facial emotion datasets. It's evident that they will continue to play an ever-growing role in shaping both academic landscapes and practical tools, ensuring their relevance for years to come.
Summary of Key Insights
As explored in the depths of this article, several key insights emerge regarding facial emotion datasets:
- Catalysts for Innovation: These datasets act as crucial elements for the development of more sophisticated algorithms in emotion recognition, which can lead to breakthroughs in various industries.
- Research Enhancements: Academic investigations in psychology gain depth and breadth by utilizing data that captures nuanced emotional expressions.
- Ethical Dilemmas: The challenges of bias, representation, and privacy highlight the necessity for stringent ethical standards when handling such sensitive information.
By summarizing these prominent themes, professionals, students, and educators can take actionable steps forward, integrating these insights into their work or studies.
Future Research Directions
Looking ahead, the realm of facial emotion dataset research is filled with potential. Some promising directions for future exploration include:
- Technological Breakthroughs: Continued advancements in AI and deep learning models are likely to yield improved emotion detection accuracy. Technologies such as reinforcement learning might play a role in enhancing the adaptability of these systems.
- Interdisciplinary Collaboration: Merging fields like psychology, neuroscience, and computer science opens new avenues for understanding complex emotional states. Such collaborations can enhance the validity and applicability of research findings.
- Real-world Applications: Integrating these datasets into real-world applications, like therapeutic tools in mental health, can provide practical benefits and enrich patient interactions.
Through these focused research directions, the synergy between emotional recognition and technology may lead to solutions that not only advance academic understanding but also foster societal growth.