Exploring the Role of Observational Trials in Research
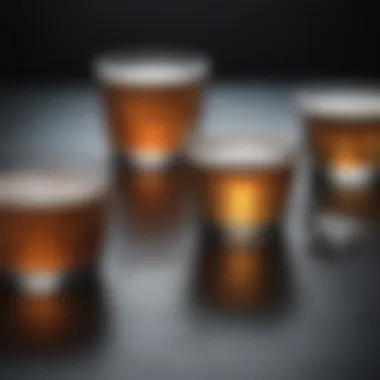
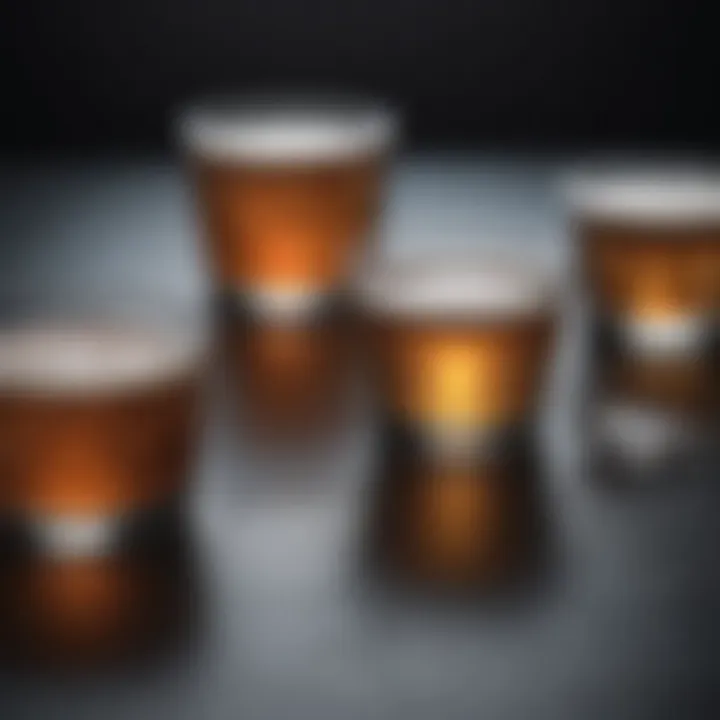
Intro
The world of research is vast, with various methodologies providing different lenses through which we can examine scientific questions. One of the essential methods in this realm is observational trials. Unlike their interventional counterparts, which actively impose treatments or manipulations, observational studies take a step back. They look at normal behaviors, conditions, or outcomes in real-world settings without interfering.
Understanding observational trials is crucial for anyone involved in research, whether they are students, seasoned researchers, or educators. These trials shine a light on various health, social, and environmental factors while raising significant questions about correlation versus causation.
Through this article, we will explore the different types of observational trials, the methodologies that come into play, the ethical considerations involved, and the challenges they present. This discourse will reflect not just an examination of the process but also an appreciation of how these trials contribute to public health, policy-making, and evidence-based medicine.
Research Background
Overview of the Scientific Problem Addressed
Observational trials serve as a response to many scientific queries that remain unanswered through traditional experimental designs. They address the fundamental problem of understanding how different variables impact health outcomes without the constraints of a controlled experiment.
For instance, consider the link between lifestyle choices and chronic diseases. Experimental setups may not always be feasible or ethical when dealing with human subjects. This limitation makes observational studies vital as they allow researchers to gather real-world data on how these choices affect health without dictating the lives of participants.
Historical Context and Previous Studies
The roots of observational research can be traced back to early epidemiological studies. One notable example is the Framingham Heart Study, launched in 1948. This long-term study has significantly influenced the understanding of cardiovascular disease by systematically observing a cohort of individuals over decades.
Historically, observational methods were often underestimated, overshadowed by the perceived rigor of randomized controlled trials. However, as more evidence emerged about the importance of context and real-world implications, the status of observational studies has risen. Today, they are seen not just as secondary data sources, but as crucial players in hypothesis generation and policy development.
Findings and Discussion
Key Results of the Research
To fully appreciate observational trials, one must understand the vast array of methodologies employed. Typically, these are categorized into cohort studies, case-control studies, and cross-sectional studies. Each type serves a unique purpose in research:
- Cohort Studies: These studies follow groups over time to observe outcomes based on exposure to certain variables.
- Case-Control Studies: Here, researchers start with an outcome and look back to see how certain factors may have influenced that result.
- Cross-Sectional Studies: These provide a snapshot in time, analyzing data from a population at one given moment.
Interpretation of the Findings
The richness of data gathered from observational trials highlights the importance of statistical analyses. Techniques such as multivariate regression and propensity score matching have become instrumental in mitigating biases and enhancing the validity of findings.
"Understanding the nuances within observational studies can translate into better clinical practices and targeted interventions."
However, observational trials are not without flaws. Issues like selection bias, recall bias, and confounding variables can distort findings if not appropriately accounted for. Yet, being aware of these limitations does not diminish their importance; rather, it sharpens the focus on improving methodologies.
This ever-evolving landscape of observational research continues to shape our understanding of numerous fields, making it essential for professionals to stay informed and engaged with the latest findings and methodologies.
Preface to Observational Trials
Observational trials play a pivotal role in the realm of research, providing insights that interventional studies sometimes overlook. These trials focus on watching real-world scenarios without manipulating any variables, which reflects how various factors in natural settings can impact outcomes. This is particularly important in fields like public health and social sciences, where understanding behavior and conditions in their natural context yields valuable information.
The advantage of observational trials lies in their ability to gather data from large populations over extended periods, allowing researchers to identify patterns and long-term effects that might not be evident in shorter studies. They are essential for generating hypotheses that can later be tested through experimental methods. Despite their many benefits, observational trials also come with their set of challenges, such as confounding variables and selection bias, which researchers must carefully navigate to draw valid conclusions.
Definition and Purpose
At its core, an observational trial is designed to study outcomes without intervention. Defining these trials keeps us grounded; without a clear explanation, ambiguity could cloud a lot of discussions. The primary purpose of observational trials is to explore correlations between variables. Researchers may want to understand how lifestyle choices, environmental factors, or genetic predisposition influence health conditions or behaviors. For instance, a study might observe the dietary habits of individuals over time and see how these habits relate to obesity rates.
The observational nature allows for flexibility in design and implementation, giving researchers the chance to adapt their focus based on emerging data and trends. In some ways, this makes observational studies invaluable for monitoring public health or studying diseases that develop in a gradual manner, as they reflect the complexities of real life rather than a controlled lab environment.
Historical Context
The lineage of observational research stretches back through centuries of scientific inquiry. Initially, these studies were more anecdotal than evidence-based, as researchers relied on their observations to hypothesize about phenomena. The groundwork for modern observational trials can be traced back to figures like John Snow, a 19th-century physician revered for his pioneering work in tracing the source of a cholera outbreak in London. By meticulously observing the spread of the disease and its correlation with a certain water pump, Snow laid the foundational principles that guide observational studies today.
Over the years, as science has evolved, so have the methodologies surrounding these trials. The introduction of statistical methods in the early 20th century further enriched observational research by allowing for more rigorous analysis of collected data. This transitional phase marked a shift from mere observation to a structured approach, culminating in the diversified types of observational studies we recognize today. The rise of technology in the latter part of the 20th century has also informed how data is collected and analyzed, enhancing the overall quality and reach of observational trials.
"Observational studies can provide a wealth of information that can be crucial for understanding complexities that controlled studies might miss."
This rich history not only showcases the evolution of observational trials but also highlights their importance in shaping public health policies and guiding future research directions. Understanding this context is crucial for grasping the full weight of observational trials in modern research.
Types of Observational Trials
Understanding the various types of observational trials is crucial in the realm of research. These trials serve as the backbone for a range of studies, shedding light on real-world outcomes without direct intervention. Each type has its own set of strengths and applications. Recognizing where and how they fit can often be the difference between fruitful insights and misleading conclusions. The distinctions are not merely academic; they shape data interpretation, inform practices, and even influence healthcare policy.
Cohort Studies
Cohort studies are often like watching a series unfold over time in a well-written drama. Researchers observe a group of participants—say, people exposed to a certain medication—and track them longitudinally. The aim here is to see how their health outcomes develop compared to another group not exposed to the medication. What makes these studies stand out is their ability to establish temporal relationships. You see who developed what and when, helping to point out potential risk factors or outcomes that may be linked. Advantages include the ability to gather a wealth of data regarding lifestyle factors and other variables that might not be apparent in a shorter study. These studies are especially valued in epidemiology, where understanding the impact of health behaviors over a long period can uncover significant trends.
Case-Control Studies
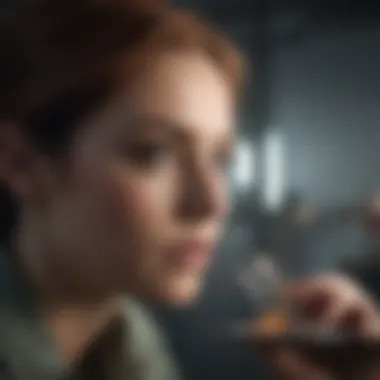
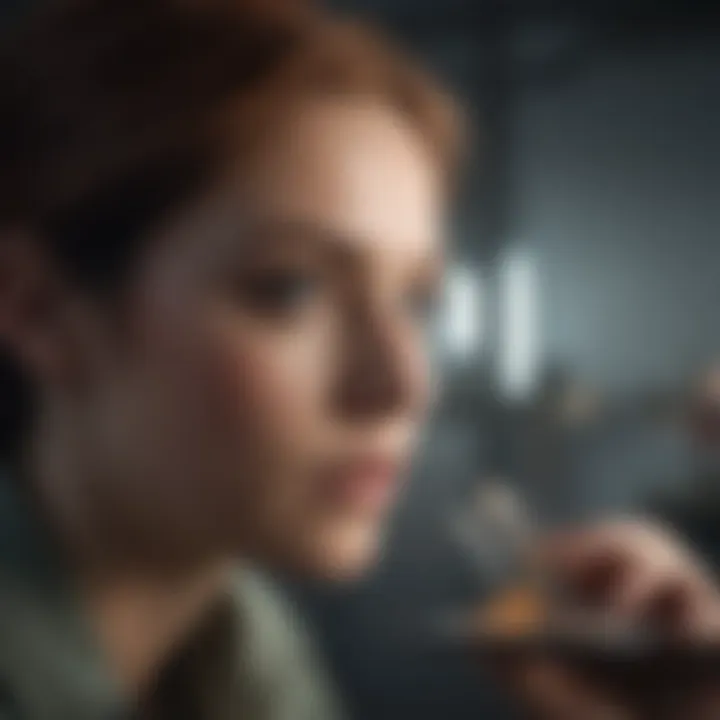
If cohort studies are the long-running series, case-control studies are more akin to a detective story—solving the mystery after the fact. In these studies, researchers start with an outcome, such as a disease, and look back at exposure to potential risk factors. For example, if an outbreak of a rare condition is observed, the researchers might compare people who have that condition with those who don't, trying to determine what distinguishes them. Case-control studies are efficient and often quicker to conduct. They lend themselves well to studying rare diseases, where finding a large enough sample for cohort studies could be impractical. Shortcomings do exist, particularly regarding recall bias, as participants may not accurately remember past exposures.
Cross-Sectional Studies
Cross-sectional studies are like capturing a moment in time—a snapshot of reality, if you will. Researchers collect data from a population at a specific point and analyze correlations between various factors. This type is particularly useful for assessing the prevalence of health outcomes or behaviors. You often hear them used in public health to gauge the spread of diseases or identify health disparities across demographics. However, the catch is that while these studies provide valuable information about associations, they can’t draw conclusions about cause and effect. Just because two variables appear related doesn’t mean one causes the other. These studies are essential for formulating hypotheses that can later be explored through more rigorous long-term studies.
Longitudinal Observational Studies
Longitudinal observational studies combine elements from both cohort and cross-sectional studies. Picture a journey where researchers look at the same subjects repeatedly over time, gathering data at multiple intervals. This design helps track changes and developments in health status or other variables. They can provide a deeper understanding of how certain factors might influence outcomes over periods, revealing trends that might otherwise be overlooked. However, they require a bigger commitment, both in time and resources, which can lead to significant challenges in retaining participant enrollment. This factor makes adherence even more critical as dropouts can skew results and interpretations.
"In every observational trial, understanding the type being used is key to interpreting results correctly. Each type brings its own flavor to the research landscape, offering unique insights where experiments may not."
For anyone delving into the realm of observational research, grasping these various types is vital. Each has its own strengths and weaknesses that can influence research conclusions significantly. Understanding how they operate ensures that anyone engaging with the data can appreciate the nuances involved—leading to better interpretations and advancements in the field.
Methodological Approaches
Understanding the various methodological approaches in observational trials is paramount for fostering robust research conclusions. These elements not only dictate how researchers gather data but also shape the overall integrity of their findings. By meticulously considering the study design and data collection methods, researchers can uncover significant insights that reflect real-world outcomes. Moreover, the careful selection of these methodologies greatly influences the credibility of the research and its applicability in clinical practice.
Study Design Considerations
When delving into study design, various factors must be considered to lay a solid foundation for any observational trial. The choice of whether to adopt a cohort study or a cross-sectional design, for instance, hinges on the research question posed. For example, cohort studies follow participants over time to see how different exposures affect outcomes, while cross-sectional studies provide a snapshot of a population at a certain moment. This fundamental difference impacts the type of data collected and the conclusions drawn.
In essence, a well-thought-out design approach minimizes biases and maximizes the reliability of the results. Balancing these aspects with practical considerations like resource availability, time constraints, and participant recruitment also plays a critical role in the ultimate success of the trial.
Data Collection Methods
The methods employed for data collection in observational trials can either make or break the integrity of the research. Here's a closer look at several key methods:
Surveys
Surveys are a popular choice in observational research due to their ability to gather a considerable amount of data from a diverse population relatively quickly. Typically characterized by structured questionnaires, surveys can address a wide range of topics and capture participants' attitudes, beliefs, and behaviors. A unique feature of surveys is their adaptability; they can be distributed online, mailed, or conducted face-to-face, depending on what fits the study best.
However, while surveys are generally efficient, they can introduce challenges such as response bias, where individuals may not answer truthfully. Nevertheless, when designed thoughtfully, surveys serve as a powerful tool for gathering insights that can inform hypotheses and drive future research.
Interviews
Interviews provide a more in-depth perspective on participant experiences and perceptions. This qualitative method can reveal complexities that surveys might overlook, offering richness to the data collection process. Usually, interviews are semi-structured, allowing the interviewer to probe deeper based on responses, which can lead to unexpected insights.
Nonetheless, interviews can be time-consuming and may lead to interviewer bias if not properly managed. Despite these downsides, the unique ability of interviews to provide nuanced perspectives makes them a valuable asset in observational research.
Existing Databases
Utilizing existing databases refers to leveraging pre-collected data from previous studies or public records. This method offers a wealth of historical data that would be impractical or impossible to gather anew. A significant advantage of this approach is that researchers can analyze large datasets, enhancing the statistical power of their findings.
However, relying on existing databases can come with its set of limitations, like restricted access to detailed data or inconsistencies in the information collected. Thus, careful consideration of the database's origins and the potential for confounding variables is crucial in this method’s application.
Ethical Considerations
Ethical considerations hold a pivotal role in observational trials, serving as a compass to guide researchers through complex moral landscapes. Unlike randomized controlled trials, where participants are assigned to interventions, observational studies rely on real-world data and natural behavior. This uniqueness raises various ethical questions, primarily focusing on patient rights, data integrity, and societal implications.
Informed Consent
Informed consent is one of the cornerstones when conducting observational research. It ensures that participants have a clear understanding of the study, its objectives, and any potential risks involved. Transparency in this process helps foster trust between researchers and participants, a crucial factor considering that individuals often share sensitive information during these trials.
Moreover, the process of obtaining informed consent must be adaptable. Situations may arise where it’s impractical or even infeasible to get consent from all participants—especially in studies using pre-existing data. Researchers often grapple with how to ethically navigate these instances. One approach could be to use anonymization methods while clearly explaining the procedures to participants. This can mitigate risks while maintaining the study's integrity.
The benefits of informed consent are manifold:
- Respect for Autonomy: Participants make their own choices, fully aware of the implications.
- Safeguarding Rights: Protecting participant rights leads to ethical research practices.
- Enhancing Credibility: Studies that prioritize informed consent often yield results viewed as more reliable and valid.
As such, the recruitment process should emphasize the voluntary nature of participation and provide comprehensive information to prospective participants. Each step should be designed not just for compliance, but to encourage an informed choice.
Privacy and Confidentiality
Privacy and confidentiality are fundamental ethical principles in observational trials, especially when handling personal data. Participants need assurance that their information will be kept safe and used solely for research purposes. Protecting this delicate information goes beyond mere regulations; it speaks to the ethical obligation of researchers to honor the trust bestowed upon them.
In today's world, where data breaches and misuse are all too common, the importance of maintaining privacy can't be overstated. Here are key considerations:
- Data Encryption: Employ robust encryption protocols to secure sensitive information.
- Limited Access: Ensure that only authorized personnel can access personal data, minimizing exposure risk.
- Anonymization Techniques: Use methods to strip identifiable elements from datasets, thus enhancing participant anonymity.
"Trust is built with consistency. Upholding privacy and confidentiality in research builds confidence among participants, allowing for richer data collection."
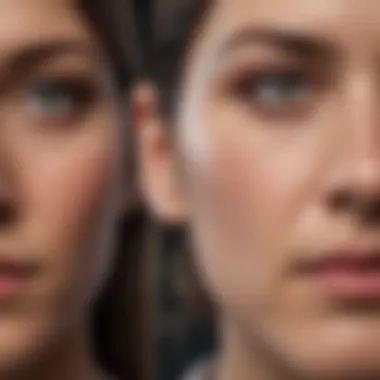
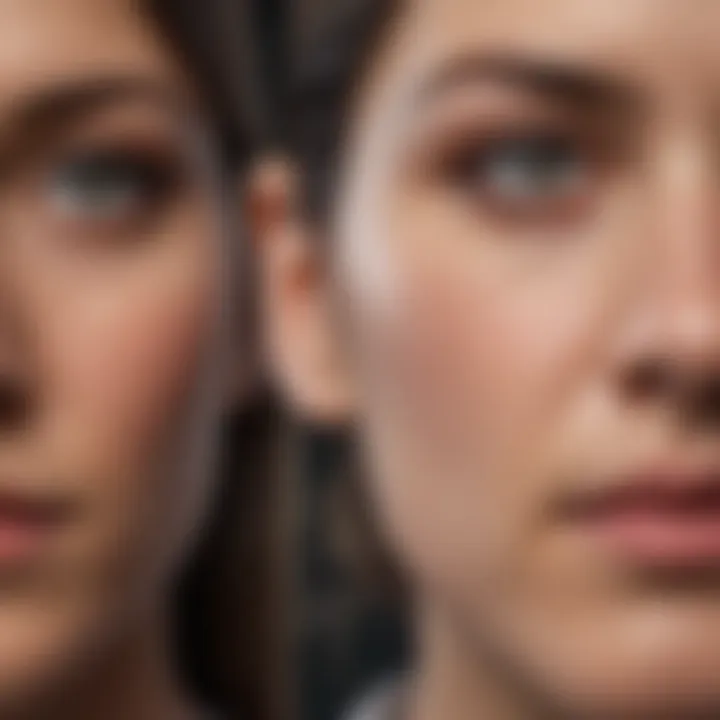
Maintaining confidentiality is not merely a regulatory obligation; it cultivates a collaborative environment. When participants feel secure in sharing their narratives, the resulting data tends to be richer and more comprehensive, thus strengthening the quality of findings.
In summary, ethical considerations in observational trials are paramount. They create a framework within which researchers operate. By diligently ensuring informed consent and safeguarding privacy, the integrity of observational research is enhanced, paving the path for significant findings that respect participant rights.
Challenges in Observational Trials
Observational trials offer a unique lens through which researchers can study the real world, allowing for insights that interventional trials sometimes miss. However, they are not without their hurdles. Understanding the challenges involved in these studies is crucial for drawing accurate conclusions and ensuring the integrity of the research. Let's explore three major challenges that frequently arise in observational trials: selection bias, confounding variables, and data quality issues.
Selection Bias
Selection bias occurs when the participants included in a study are not representative of the broader population they are meant to reflect. This can lead to skewed results and conclusions, as the outcomes observed may not be applicable to the general public. For instance, if a cohort study investigating a new medication only recruits participants from a single hospital, the findings might not reflect how the medication performs in different demographics or settings.
The challenge lies in constructing a study that ensures a truly representative sample. Methods to handle selection bias include using random sampling techniques, ensuring diverse inclusion criteria, and utilizing weighting strategies in data analysis.
As a rule of thumb, researchers should always question their sample's applicability. Without a representative sample, the results risk becoming more of a reflection of those selected rather than an insight into the wider population.
Confounding Variables
Confounding variables are those pesky factors that can muddy the waters of research. They may be correlated with both the exposure being studied and the outcome, leading to incorrect assumptions about cause and effect. For instance, if a researcher is studying the relationship between physical activity and heart health, failing to account for factors like age or existing health conditions can lead to misleading conclusions.
Addressing confounding requires a mix of careful study design and thorough statistical analysis. Researchers should be proactive in identifying potential confounders and either control for them in the design phase or adjust for them during the analysis. Techniques like stratification or multivariable regression can help parse out the true relationships between variables.
"Identifying confounding factors is akin to piecing together a puzzle; you need to ensure every piece fits to reveal the complete picture."
Data Quality Issues
Finally, the overarching concern of data quality can’t be ignored. Poor data leads to unreliable results and, ultimately, flawed conclusions. Issues can range from missing data entries to inaccuracies in self-reported data. In observational studies, data is often collected through surveys or interviews, making it susceptible to biases like recall bias—where participants may not remember past events accurately.
To mitigate these issues, it's important to implement rigorous data collection methods. Researchers should establish clear protocols for data entry, and where possible, triangulate data sources to corroborate findings. Regular audits and pilot studies can also help identify potential pitfalls before the actual data collection begins.
Statistical Methods in Observational Research
In the world of research, statistical methods serve as the backbone that supports the findings and conclusions drawn from observational trials. They are vital in interpreting data accurately, ensuring that the results are not just mere numbers but rather meaningful insights that can influence theories and practices. When addressing observational trials, it’s crucial to apply the right statistical techniques to make sense of complex datasets, tailor interventions, or inform policy decisions. As the variety of methods grows, so does the importance of selecting the appropriate techniques for effective analysis.
Descriptive Statistics
Descriptive statistics are the starting point for any data analysis in observational research. They provide a simple summary of the sample and the measures. These statistics offer a snapshot by presenting data in a manageable form. The key characteristics of descriptive statistics include:
- Measures of Central Tendency: These include the mean, median, and mode, which provide insights into the typical value of the data gathered. They help researchers understand what is common within the dataset.
- Measures of Dispersion: These characteristics, such as variance and standard deviation, give perspective on how much the data varies or spreads around the mean. A large standard deviation, for example, indicates that the data points are spread out over a wider range of values.
Utilizing descriptive statistics is like casting a wide net to gather comprehensive data points, which eventually informs further analysis. This foundational layer is essential before reaching into more complex inferential statistics, ensuring that researchers have a clear grasp of their data.
Inferential Statistics
Building on the groundwork laid by descriptive statistics, inferential statistics allow researchers to make predictions or inferences about a larger population based on a sample set. This branch of statistics is geared toward answering questions that go beyond immediate observations. Some primary elements include:
- Hypothesis Testing: This analyzes whether the results observed in a sample can be generalized to a broader population. Through tests like t-tests or chi-square tests, researchers assess if there are statistically significant differences or effects.
- Confidence Intervals: These intervals provide a range of values that capture the true population parameter with a specified level of certainty, often articulated as 95%. It illustrates the precision of the estimate in context.
Using inferential statistics is a bit like trying to find a needle in a haystack but with a better understanding of the layout of the haystack. It narrows down possibilities, guiding researchers to understand when findings signify more than just randomness in data.
Regression Analysis Techniques
Regression analysis techniques form an important toolkit within inferential statistics. This method helps to identify relationships between variables, explaining how the addition or change of one variable affects another. The techniques often employed include:
- Linear Regression: This approach examines the linear relationships and is effective in predicting values based on trends found within the data.
- Logistic Regression: Used especially for binary outcomes, this technique helps understand the impact of predictor variables on the possibility of a phenomenon happening or not.
- Multivariate Regression: A step beyond, this method can analyze multiple variables simultaneously, offering a broader perspective on the data set.
"Regression analysis serves as a critical lens, allowing scientists to peer into complex interdependencies that exist among variables."
In summary, statistical methods in observational research are fundamental not only for understanding data but also for deriving inferences that shape the scientific narrative. They facilitate deeper insights, encouraging an evidence-based approach to research that is both rigorous and impactful.
Implications of Observational Trials
Observational trials carry significant weight in the realm of research, making them a cornerstone for generating knowledge across various scientific fields. Primarily, they shine a light on real-world implications and offer insights that are often overlooked in controlled experimental settings. This section delves into the dual nature of observational trials—how they shape hypotheses and inform clinical practices.
Hypothesis Generation
One major implication of observational trials is their role in generating hypotheses. By scrutinizing the relationships between variables in large population samples, researchers can identify patterns or associations that might warrant deeper investigation. For instance, a study observing dietary habits and heart disease incidences might reveal surprising correlations; if informally noted, these could lead to more rigorous scientific inquiries.
- Exploratory Nature: Observational research is inherently exploratory. It allows researchers to investigate unknown associations, leading to hypothesis generation regarding causation.
- Realistic Context: Such studies reflect the complexities of real-world scenarios, providing pragmatic insights that randomized trials might miss.
- Diverse Population Sampling: In observational studies, the diverse participants often represent various backgrounds, which can introduce factors affecting health outcomes previously unconsidered.
This ability to generate complex hypotheses is indispensable in advancing scientific understanding, as it makes way for further, more nuanced explorations.
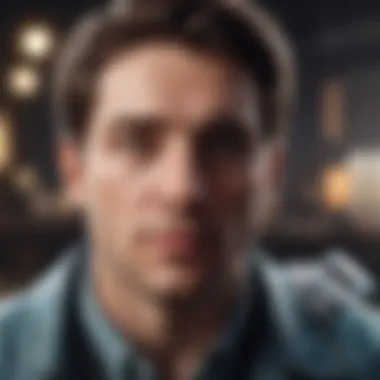
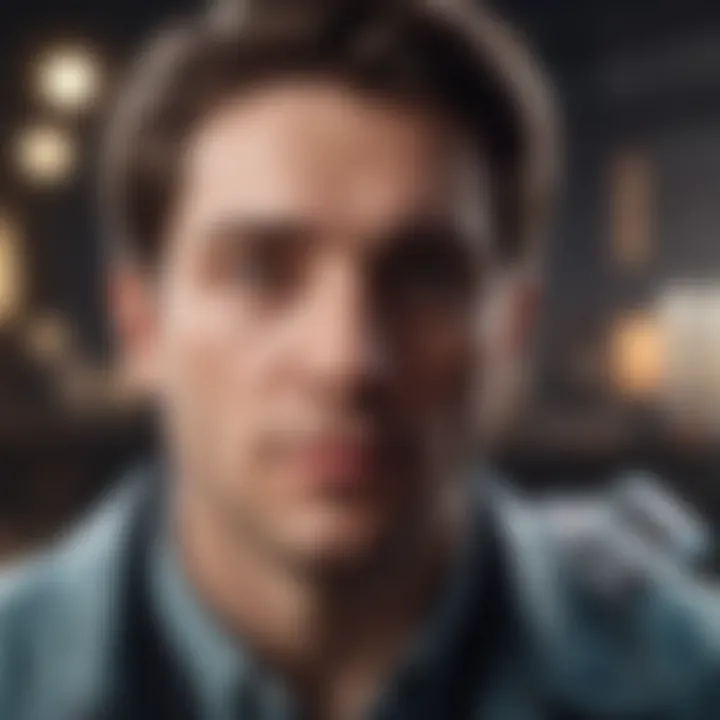
Informed Clinical Practice
Observational trials also have profound implications for clinical practice. The findings from these trials can directly guide healthcare decisions by shedding light on effectiveness and applicability of certain interventions in everyday settings. Unlike randomized controlled trials that might idealize the efficacy of a treatment, observational research shows how treatments perform in the hustle and bustle of real life.
- Evidence for Best Practices: Clinicians can base their decisions on real-world evidence concerning patient outcomes. For example, a study might show that a particular medication has higher success rates among patients with specific comorbidities, thus steering healthcare providers towards personalized treatment plans.
- Understanding Population Health Trends: Observational research adds layers of understanding concerning disease prevalence and the impact of lifestyle factors, thereby helping healthcare systems allocate resources more effectively.
- Risk Assessment and Management: The patterns revealed in observational studies can assist practitioners in assessing risks, giving them tools to inform patients accurately regarding potential outcomes of various treatment options.
"Observational trials serve as the compass for navigating the clinical landscape, guiding practitioners through the myriad complexities of patient care."
By connecting the dots between hypothesis generation and clinical application, observational trials ultimately enhance evidence-based medicine's foundations. They usher in a landscape where informed decisions are the norm, allowing clinicians and researchers alike to build on existing knowledge for future improvements.
Case Studies and Examples
When it comes to observational trials, the practical application through case studies and examples showcases real-world scenarios that highlight strengths and weaknesses in research methodologies. The importance of this section lies in its potential to transcend theoretical discussions and ground them in tangible outcomes. For students, researchers, educators, and professionals, these cases represent not just academic milestones but crucial learning experiences that shape future research endeavors.
Successful Observational Trials
Successful observational trials serve as beacons in the landscape of research, reflecting the potential of this methodology to yield impactful insights. One notable example is the Framingham Heart Study, which began in 1948 and continues to this day. Originally aimed at identifying the common risk factors for cardiovascular diseases, this long-term study has uncovered invaluable data on things like blood pressure, cholesterol levels, and lifestyle choices. Over generations of participants, researchers have gained profound understanding concerning heart disease, helping shape public health guidelines. The wealth of data generated has led to predictive models that are still in use today.
Additionally, the Nurses' Health Study provides another compelling example. Launched in 1976 with more than 120,000 registered nurses, this study has produced critical findings related to women's health, including associations between dietary habits and chronic diseases. This multi-decade observational research encouraged shifts in dietary recommendations, proving instrumental in promoting healthier lifestyles across the population.
These success stories underscore the capacity of observational trials to inform public health initiatives and drive policy changes while enhancing scientific knowledge across disciplines.
Lessons Learned from Failures
While successful trials contribute positively to the body of knowledge, failures provide equally important lessons. A pertinent instance is the Women’s Health Initiative, which faced considerable criticism upon concluding that hormone replacement therapy could have harmful effects. Initially, this trial aimed to examine the long-term health effects of hormone therapy among postmenopausal women. However, unexpected adverse outcomes steered public perception and clinical practices dramatically. The fallout led to a rift between researchers and the medical community, illuminating the roles of monitoring and reassessing protocols.
Another case involved the START (Strategic Timing of Antiretroviral Treatment) study. Here, the intention was to evaluate when to start treatment. Though the study aimed at benefiting patients, varying participant demographics and healthcare access led to inconclusive results. The implications are clear: without aligning trial designs with diverse populations, gaps in understanding can widen, leading to misinformed strategies.
These lessons exhibit that failure is not merely an end but a formative experience. They compel researchers to rethink their approaches, ensuring that methodologies are robust and inclusive enough to account for unforeseen variables.
In synthesizing examples of successes and challenges, a deeper respect for observational trials emerges. The potential for these studies to evolve and adapt can switch the narrative from mere data collection to genuine advancements in evidence-based medicine.
"Mistakes are proof that you are trying."
This notion resonates deeply within the scope of observational research, suggesting that embracing both successful and failed trials paves the way for future innovation and progress.
Future Directions in Observational Trials
As we look to the horizon of observational trials, it’s clear that we're standing at the edge of significant transformation. With growing demands for insightful, robust data that informs healthcare decisions, it’s vital to explore how future directions in observational studies can shape research methodologies and outcomes.
Integration of Technology
Big Data
Big Data plays a crucial role in observational trials by leveraging vast amounts of information to uncover patterns, trends, and insights. The key characteristic of Big Data is its ability to process large volumes of multifaceted data in real-time, which is particularly beneficial when addressing complex health issues.
- Unique Feature: One unique aspect is its capacity for longitudinal tracking, where researchers can follow patient data over time, analyzing changes and developments in health conditions. This aspect enables a richer contextual understanding of how treatment effects unfold.
- Advantages and Disadvantages: While Big Data can enhance the thoroughness of observations, researchers must also consider challenges like data quality and the risk of incorporating biases from unstructured data. Therefore, setting robust criteria for how data is collected and analyzed becomes paramount to ensure relevance and accuracy.
Artificial Intelligence
Artificial Intelligence adds another layer of sophistication to observational trials. By using algorithms to analyze data patterns, AI serves as a facilitator for better predictive analytics. The unique feature of AI is its ability to learn from datasets and improve over time, which can lead to more precise findings and hypotheses generation.
- Advantages and Disadvantages: However, while AI can refine data interpretation, the reliance on these algorithms introduces the critical need for transparency and explainability in their applications. Misinterpretation or misuse might lead to erroneous conclusions, affecting clinical practices and policy decisions. Hence, balancing innovation with critical ethical considerations is necessary.
Evolving Regulatory Frameworks
Navigating the complexities of regulatory frameworks is essential in the realm of observational trials. As technology evolves, so too must regulations which govern data use. The importance here lies in fostering an environment where research innovation can thrive without compromising participant safety or data integrity.
- Responding to Change: As observational research increasingly involves data sourced from various digital platforms, regulators must adapt to address novel challenges such as data ownership, patient consent, and the ethical use of AI. Creating flexible yet protective frameworks can enhance trust in research outcomes and encourage broader participation from diverse populations.
- Global Perspective: Furthermore, the global character of observational studies necessitates collaborative efforts to harmonize regulatory standards across borders. This alignment can facilitate the exchange of knowledge and promote the development of more comprehensive datasets, ultimately enriching the scientific community.
The future of observational trials is not just about integrating cutting-edge tools; it’s about understanding how these advancements can optimize research while keeping ethical practices in the foreground. By placing attention on technology and evolving frameworks, we can pave the way for observational studies that not only produce valuable insights but also uphold the highest standards of research integrity.
End
Observational trials represent a cornerstone in the landscape of scientific research. The essence of these studies lies in their ability to provide rich, real-world data that can illuminate trends and associations that might otherwise remain hidden in controlled environments. The significance of concluding this article with a thorough exploration of observational studies underscores their pivotal role in formulating medical guidelines and policy-making.
Summary of Key Points
In summing up the key points discussed throughout this article, observatinal trials, unlike their interventional counterparts, do not seek to test hypotheses under strict conditions. Instead, they allow researchers to gather information based on actual circumstances and behaviors. Here are some fundamental takeaways:
- Observational trials can take various forms such as cohort studies, case-control studies, and cross-sectional studies, each with its own strengths and weaknesses.
- Methodological rigor is crucial to mitigate challenges like selection bias and confounding variables, which can impede the validity of findings.
- Ethical considerations, especially ensuring informed consent and protecting participant privacy, remain at the forefront of observational research.
- Advanced statistical methods are employed to analyze data, providing insights that shape clinical practices and enhance evidence-based medicine.
- The evolution of technology, particularly big data and AI, presents new frontiers for observational research, expanding its capabilities and reach.
Significance in the Scientific Community
The contributions of observational trials extend beyond mere data collection. These studies address a gap that randomized controlled trials often overlook. They reflect the dynamics of everyday life and bring forth new hypotheses that can later be tested in more controlled settings. This intrinsic value becomes vital as the healthcare landscape faces rapidly evolving challenges.
Furthermore, observational trials have underscored their importance in public health. For example, they have been essential in tracking disease patterns, outcomes of medical interventions, and the effectiveness of health policies in diverse populations. This not only empowers researchers but also informs practitioners who rely on solid evidence to guide their decisions.
As the scientific community continues to embrace these observational methodologies, the dialogue surrounding their application will likely expand. This will lead to a richer understanding of complex health phenomena, ultimately advancing the fields of medicine and public health.