Understanding Meta-Analysis: Definition and Applications
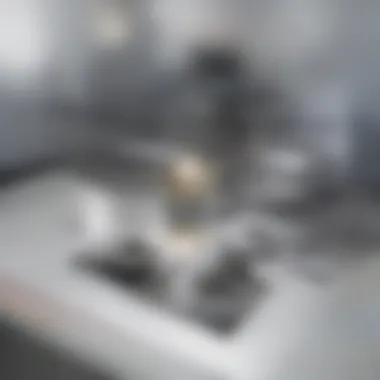
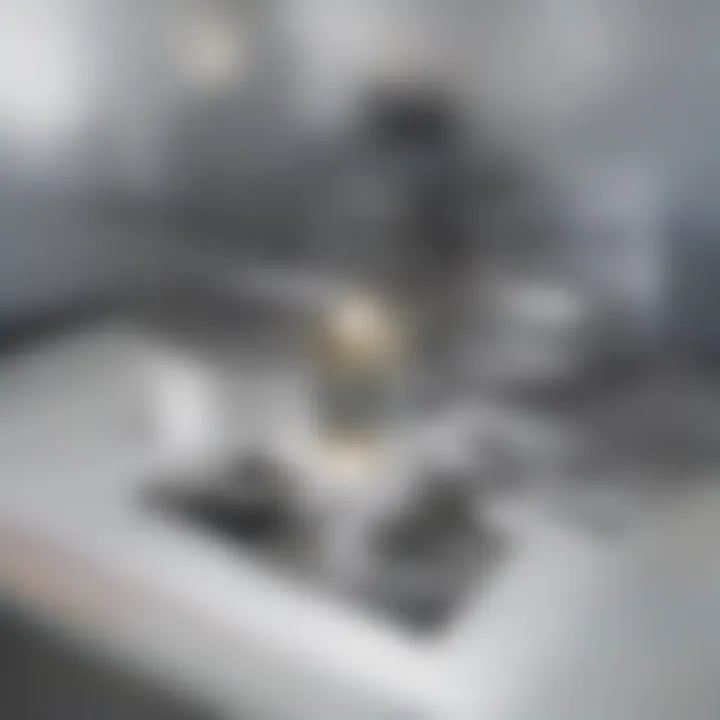
Intro
Meta-analysis acts as a powerful tool in research, allowing for the integration and synthesis of findings from multiple studies. It offers researchers the ability to create a cohesive body of evidence that can inform practice and guide future research. This approach is not merely a statistical exercise; it is a means of enhancing understanding across various scientific fields. However, it is vital to grasp the complexities involved in conducting a meta-analysis, as well as the implications of its findings. A deeper comprehension of this process can significantly influence evidence-based decision-making, shaping policies and practices in diverse domains.
Research Background
Overview of the scientific problem addressed
The primary scientific challenge that meta-analysis addresses is the variability in research findings. Often, individual studies yield conflicting results, complicating the ability to draw meaningful conclusions. This inconsistency makes it difficult for practitioners and policymakers to rely on singular research outcomes.
Meta-analysis seeks to resolve these issues by systematically combining data from various studies. This is particularly useful in fields such as medicine, psychology, and education. Researchers can thus establish clearer patterns and gain insights that individual studies may not reveal.
Historical context and previous studies
The concept of meta-analysis has evolved significantly since its emergence in the 1970s, when the term was first articulated by Gene V. Glass. Early applications were concentrated in social sciences, but over time, its use expanded into healthcare, behavioral studies, and even economics. Researchers like Harris Cooper further refined the methods, leading to more structured approaches that address both the synthesis of data and the underlying methodological rigor.
Studies such as the one by Borenstein et al. laid foundational work highlighting statistical techniques that facilitate meta-analyses. They provided clear guidance on effect sizes, confidence intervals, and heterogeneity assessment. This historical development underscores the significance of meta-analysis in contributing to robust and reliable scientific knowledge across disciplines.
"Meta-analysis is an essential tool for breaking the silence of countless lone voices of research – synthesizing them into a coherent understanding of complex phenomena."
Findings and Discussion
Key results of the research
Research using meta-analysis has demonstrated its effectiveness in various contexts. For instance, in medicine, it has been instrumental in confirming the efficacy of treatments or interventions. An analysis might combine the results of several randomized controlled trials to evaluate whether a specific drug shows statistically significant benefits over placebos. This synthesis strengthens the overall evidence base.
Interpretation of the findings
The interpretation of meta-analysis findings requires careful consideration of the data and methodologies involved. Researchers must evaluate not only the aggregated results but also the quality of the individual studies included in the analysis. Bias, sample sizes, and study designs can all impact the synthesis. A thorough meta-analysis takes these factors into account, ensuring that the findings reflect a more nuanced understanding of the effects being studied.
Defining Meta-Analysis
Meta-analysis is an essential component of research synthesis. It serves as a powerful tool that combines findings from various studies, allowing for a more comprehensive understanding of a specific phenomenon. This section will discuss the significance of defining meta-analysis in detail, including its key elements, benefits, and considerations.
Conceptual Overview
Meta-analysis can be described as a statistical methodology that integrates results from numerous independent studies focusing on the same research question. By quantitatively assessing these studies, researchers can derive a weighted average of effect sizes, providing insights that a single study might not capture.
Some critical elements of meta-analysis include:
- Study Selection: Carefully choosing which studies to include based on predefined criteria to ensure relevance and reduce bias.
- Data Extraction: Systematically collecting and consolidating data from selected studies for analysis.
- Statistical Analysis: Employing various statistical methods to summarize the data and draw conclusions.
The benefits of conducting a meta-analysis are significant. Researchers can enhance the statistical power of their findings by aggregating results from multiple sources. This approach reveals consistent patterns across studies that may not be evident in isolated research. Further, it informs evidence-based practices by providing a more robust foundation for decision-making.
Historical Context
The concept of meta-analysis began gaining traction in the late 20th century, particularly in the fields of medicine and psychology. In 1976, the pioneering work by Gene V. Glass introduced the term 'meta-analysis' to describe the methodological process of synthesizing research findings quantitatively. This period marked a shift towards a more systematic approach in evaluating research, which was essential given the growing volume of published studies.
Over time, meta-analysis has evolved considerably, with improvements in statistical techniques and software, such as RevMan and Comprehensive Meta-Analysis, making it more accessible. The advent of the internet also allowed for easier access to a broader range of studies, which further enriched the practice of meta-analysis.
As the importance of systematic reviews and meta-analyses became recognized, funding agencies began to encourage their use in research proposals. This led to a rise in the number of meta-analyses published across various disciplines, fostering greater collaboration among researchers and enhancing the quality of evidence in fields like public health, environmental studies, and social sciences.
In summary, defining meta-analysis is critical for understanding its role in research synthesis. By looking at its conceptual foundations and historical context, readers can appreciate its impact on evidence-based practices and the advancement of scientific inquiry.
The Methodology of Meta-Analysis
The methodology of meta-analysis represents a foundational pillar in this powerful research tool. It encompasses various systematic approaches that any rigorous meta-analysis must adopt. Understanding these methodologies is crucial for data integration and validity in findings. Each step within the methodology provides structure and ensures each analysis is comprehensive and robust. This section dissects the key components of this methodology - selection of studies, data extraction techniques, and statistical analyses - thereby illuminating their importance to the overall process.
Selection of Studies
The selection of studies is a critical component within the meta-analysis framework. This step determines which research will contribute to the overall conclusion and outcomes of the meta-analysis.
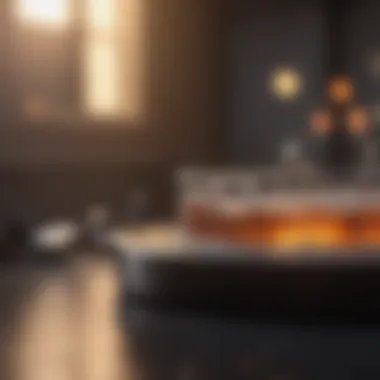
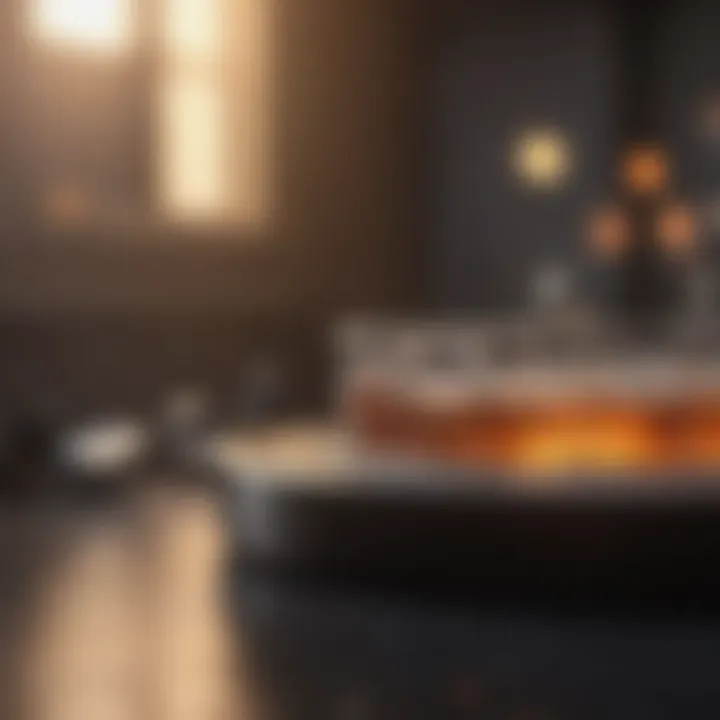
To effectively conduct this selection, a few factors need to be taken into account:
- Inclusion and Exclusion Criteria: Establishing strict guidelines helps in filtering relevant studies. These criteria should align with the research questions posed by the meta-analysis. Studies must meet certain thresholds for quality and applicability.
- Database Searches: Utilizing databases such as PubMed, Cochrane Library, and Scopus is crucial. Collecting literature from multiple reputable sources reinforces the integrity of the selection process.
- Systematic Review Protocol: Following a systematic review protocol enhances reproducibility and transparency. This protocol should detail the methods for study selection, data extraction, and analysis.
Overall, selecting studies with rigor shapes the quality of the meta-analysis and can significantly impact the conclusions drawn.
Data Extraction Techniques
After identifying the relevant studies, data extraction techniques come into play. This process focuses on gathering information from each study included in the meta-analysis.
Key elements of data extraction include:
- Standardized Forms: Utilizing templates or forms for data extraction ensures consistency across studies. This standardization is fundamental in minimizing errors and biases during the data collection phase.
- Extraction Variables: Predetermined variables such as sample size, study design, and outcomes are critical. Documenting these details ensures that important data is systematically captured.
- Double Data Entry: In some cases, double data entry by independent reviewers is recommended. This practice enhances reliability, as it allows for discrepancies to be identified and resolved before analysis.
Through careful data extraction, researchers can lay the groundwork for statistically sound analyses that follow.
Statistical Analyses in Meta-Analysis
Statistical analyses are the final step in the methodology of meta-analysis. This aspect interprets and summarizes the collected data, allowing for an overall understanding of the research landscape.
Important considerations in this stage include:
- Choice of Model: Selecting between fixed-effect and random-effects models is crucial. The fixed-effect model assumes that all studies estimate the same underlying effect, while the random-effects model accounts for variability across studies.
- Heterogeneity Assessment: This evaluation determines whether variations in results across studies are due to actual differences or random chance. Tools such as I² statistic help quantify this heterogeneity.
- Sensitivity Analysis: Conducting sensitivity analyses allows the researcher to investigate how results may change with the inclusion or exclusion of certain studies. This adds robustness to the overall findings.
The integration and interpretation of statistical analyses not only enhance the findings of a meta-analysis but also contribute significant insights to the respective research fields.
Types of Meta-Analysis
Understanding the different types of meta-analysis is crucial for researchers and practitioners. Each type serves distinct purposes and employs various methodologies that influence outcomes. When conducting a meta-analysis, selecting the appropriate type ensures that the synthesized results accurately reflect the body of evidence in a given field. This understanding guides researchers in interpreting findings and informs decision-making in practice.
Fixed-Effect Meta-Analysis
Fixed-effect meta-analysis is based on the assumption that all the studies share a common effect size. This method is most applicable when the studies being analyzed are similar in nature, such as those examining the same intervention or exposure across similar populations. It calculates an overall effect size by averaging the results from individual studies, weighting them based on their precision, typically represented by the inverse variance.
The primary benefit of fixed-effect meta-analysis is its simplicity and clarity. When the assumption of homogeneity holds true, it offers a precise estimate of the overall effect. However, this approach becomes problematic when there is significant variability or heterogeneity among studies, as it may lead to inaccurate conclusions.
Random-Effects Meta-Analysis
In contrast to fixed-effect meta-analysis, random-effects meta-analysis acknowledges the inherent variability across studies. This method not only accounts for the sampling error within each study but also includes the variation between different studies. The underlying rationale is that the true effect may differ from one study to another due to factors like population differences, study design, and intervention types.
Random-effects meta-analysis provides a more generalizable conclusion, especially when studies are heterogeneous. The results produced are often less precise but more reflective of the real-world diversity in research findings. It allows researchers to capture the wider range of possible effects that could exist across diverse contexts and methodologies.
Network Meta-Analysis
Network meta-analysis expands upon traditional meta-analysis techniques by allowing the synthesis of multiple treatments simultaneously, even if some treatments have not been compared directly in head-to-head trials. This method creates a network of studies that compare various interventions, aligning them in a manner that provides a comparative effectiveness analysis across different treatment options.
The advantage of network meta-analysis lies in its ability to inform treatment guidelines, particularly in clinical contexts where direct comparisons may be lacking. It enables a broader understanding of how different interventions stack up against each other, which is invaluable for practitioners making informed decisions. However, the complexity of setting up and interpreting network meta-analyses requires careful consideration and advanced statistical expertise.
"Choosing the right type of meta-analysis is pivotal for achieving reliable results and has a profound impact on conclusions drawn from the data."
In summary, understanding these types of meta-analysis—fixed-effect, random-effects, and network—is vital for any researcher or professional looking to synthesize evidence effectively. Each approach has its unique strengths and considerations, guiding the interpretation and application of findings across various disciplines.
Importance of Meta-Analysis in Research
Meta-analysis plays a crucial role in synthesizing information from multiple studies. Its significance extends beyond mere data aggregation; it enhances the overall validity and robustness of research conclusions. By combining results from diverse studies, meta-analysis helps to reveal broader trends and patterns that a single study may not capture. This process is essential for ensuring that findings are not only statistically significant but also reflective of real-world outcomes.
Enhancing Statistical Power
One of the primary benefits of meta-analysis is its ability to enhance statistical power. Individual studies often have limited sample sizes, which can affect the accuracy and reliability of their conclusions. When results from smaller studies are pooled together, the overall sample size increases. A larger dataset improves the likelihood of detecting true effects and reduces the chance of Type II errors—failing to identify an effect that is present. This amplification of statistical power makes it easier to establish meaningful relationships within the data.
In addition to increasing statistical power, meta-analysis facilitates the examination of subgroups and interactions, offering greater insight into specific conditions or populations. Researchers can generate more nuanced conclusions that can guide further studies or application in practice.
Identifying Consistent Patterns
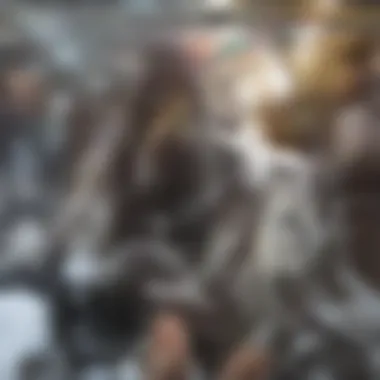
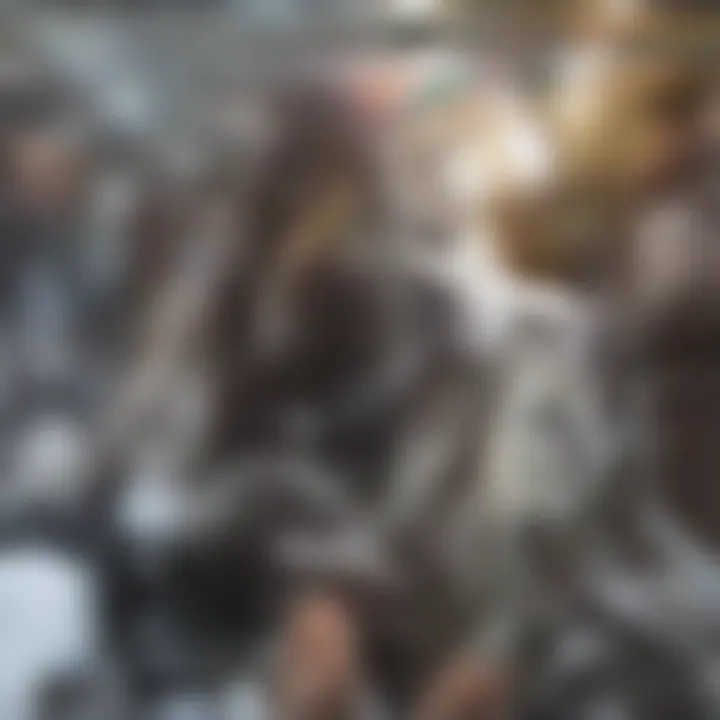
Meta-analysis is instrumental in identifying consistent patterns across various studies. By systematically analyzing results, it helps to discern commonalities and divergences in findings. Recognizing these patterns is fundamental for several reasons. First, it ensures that research outcomes are coherent and can contribute to a unified understanding of a phenomenon. Second, it enables researchers to challenge or confirm prevailing theories and models in their respective fields.
The identification of consistent patterns can also guide future research. By understanding where results align, researchers can pinpoint gaps in the literature that need further exploration. Can this be particularly powerful when conflicting results arise in individual studies? Meta-analysis sheds light on underlying factors contributing to such discrepancies, therefore refining the focus for future investigations.
Informing Evidence-Based Practices
The application of meta-analysis has profound implications for evidence-based practices across various disciplines, including healthcare, education, and social policy. By integrating findings from multiple studies, practitioners gain access to comprehensive evidence that can inform decision-making processes. For instance, in clinical research, meta-analyses can evaluate the effectiveness of treatments or interventions more rigorously than individual trials. This synthesis allows practitioners to adopt strategies that are backed by a compilation of empirical evidence, ultimately leading to better outcomes for patients.
Furthermore, meta-analysis encourages transparency in research by revealing inconsistencies and highlighting areas that require more in-depth study. This openness fosters a culture of continuous improvement based on evidence.
"Meta-analysis not only increases the credibility of findings but also provides a more reliable basis for formulating guidelines and recommendations in practice."
Applications of Meta-Analysis
Meta-analysis serves as a pivotal tool in synthesizing data across a multitude of fields, offering a framework that promotes robust understanding of broad trends and patterns. The unique capacity of meta-analysis to collate results from various studies allows researchers to derive insights that may not be evident from single studies alone. This capacity is vital in enhancing the strength of conclusions drawn from research efforts, ultimately fostering evidence-based practices in diverse contexts.
Clinical Research
In clinical research, meta-analysis plays a crucial role by aggregating findings from numerous studies, aiding in the evaluation of treatment efficacy and safety across populations. For instance, when assessing a new medication, individual studies may yield varied results due to sample differences or methodology. By employing meta-analysis, researchers can reconcile these discrepancies, thereby providing stronger evidence for treatment guidelines and clinical decisions. It also enhances statistical power, enabling the detection of significant effects that might be overlooked in smaller studies.
"Clinical decisions are often grounded on the synthesis of diverse evidence, and meta-analysis provides the tools to achieve that."
Environmental Studies
In the realm of environmental studies, meta-analysis is instrumental in understanding the impact of human activities on ecosystems. Studies examining pollution levels, climate change effects, or biodiversity loss may vary in scope and scale. By collating these studies, meta-analysis identifies overarching trends and causal relationships. This comprehensive understanding is critical for informing policy decisions and conservation efforts. Furthermore, it helps in evaluating the effectiveness of interventions by analyzing their outcomes across different environments and contexts.
Social Sciences
Meta-analysis is equally significant in social sciences, where it helps decipher complex social phenomena by integrating findings from various research. For example, in studying behavioral interventions aimed at improving educational outcomes, differing methodologies and contexts can complicate interpretations. A meta-analysis in this field not only clarifies the effectiveness of such interventions but also explores the factors that moderate their success. This synthesis of evidence supports the development of comprehensive theories and models, reinforcing the importance of evidence in shaping social policy and practice.
In summary, the applications of meta-analysis across clinical research, environmental studies, and social sciences underscore its significance in synthesizing diverse study findings. These applications not only enhance rigor in research but also reinforce the foundation for evidence-based practices.
Challenges in Conducting Meta-Analyses
Conducting meta-analyses is an intricate process laden with various challenges. Understanding these obstacles is crucial for researchers who aim to synthesize findings from multiple studies effectively. The significance of addressing such challenges cannot be overstated, as they can influence the outcome and reliability of the analysis. Each challenge requires careful consideration and methodology adjustments. This section elaborates on three primary challenges in conducting meta-analyses: publication bias, heterogeneity among studies, and the quality of included studies.
Publication Bias
Publication bias represents a critical challenge in meta-analyses, as it occurs when the likelihood of a study being published is influenced by its results. Primarily, studies with positive or significant outcomes are more likely to be published than those with negative or inconclusive results. This skewed publication landscape can lead to an overestimation of the effect size in meta-analyses, ultimately distorting the true relationship being investigated.
Researchers need to actively seek unpublished studies or grey literature to mitigate publication bias. Tools such as funnel plots can help visually assess potential bias in the included studies. Moreover, sensitivity analyses may provide insight into how the exclusion of certain studies affects overall findings. A comprehensive approach ensures a more accurate and trustworthy synthesis of data.
Heterogeneity Among Studies
Heterogeneity refers to the variation in study outcomes attributable to differences in study populations, interventions, methodologies, and other factors. High levels of heterogeneity complicate the interpretation of meta-analytic findings. It can lead to inconsistent estimates of effects, reducing the confidence researchers can place in the combined results.
To address heterogeneity, researchers can use statistical tests like the I² statistic and Cochran's Q test to assess the extent of variability among studies. When significant heterogeneity is detected, the use of random-effects models can provide a more appropriate framework for analysis, accommodating the diversity inherent in the datasets. Additionally, conducting subgroup analyses is valuable for identifying factors contributing to variability and refining the overall conclusions drawn from the meta-analysis.
Quality of Included Studies
The quality of studies included in a meta-analysis is paramount to its credibility. Studies that are poorly designed or have methodological flaws can skew the results and undermine the synthesis's validity. Therefore, assessing the quality of each study is essential before inclusion in the meta-analytic process.
Several tools exist for quality assessment, such as the Cochrane Risk of Bias Tool and the Newcastle-Ottawa Scale. Implementing these tools allows researchers to evaluate critical aspects of study design, reporting, and execution. Ensuring the inclusion of methodologically sound studies enhances the robustness of the meta-analysis, making it more reliable for guiding future research and evidence-based practices.
"A well-conducted meta-analysis is only as reliable as the studies it encompasses. Thus, rigorous scrutiny is essential at every step."
By understanding and addressing these challenges, researchers can improve the quality of their meta-analyses. Such attention to detail not only strengthens individual studies but also contributes to the broader landscape of scientific inquiry.
Quality Assessment in Meta-Analysis
Quality assessment in meta-analysis is crucial for ensuring the reliability of the results synthesized from various studies. It involves evaluating the methodological rigor of the included studies and helps to understand the robustness of the overall findings. This section will discuss specific elements, benefits, and considerations regarding quality assessment in meta-analysis.
The primary goal of conducting a quality assessment is to differentiate between high-quality studies and those that might introduce bias or unreliable data. This assessment sheds light on the strength of the evidence and can impact the conclusions drawn from the meta-analysis significantly. Therefore, without proper quality evaluation, the credibility of the synthesis may be jeopardized, leading to potentially misleading interpretations.
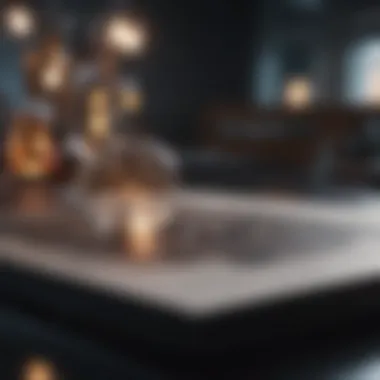
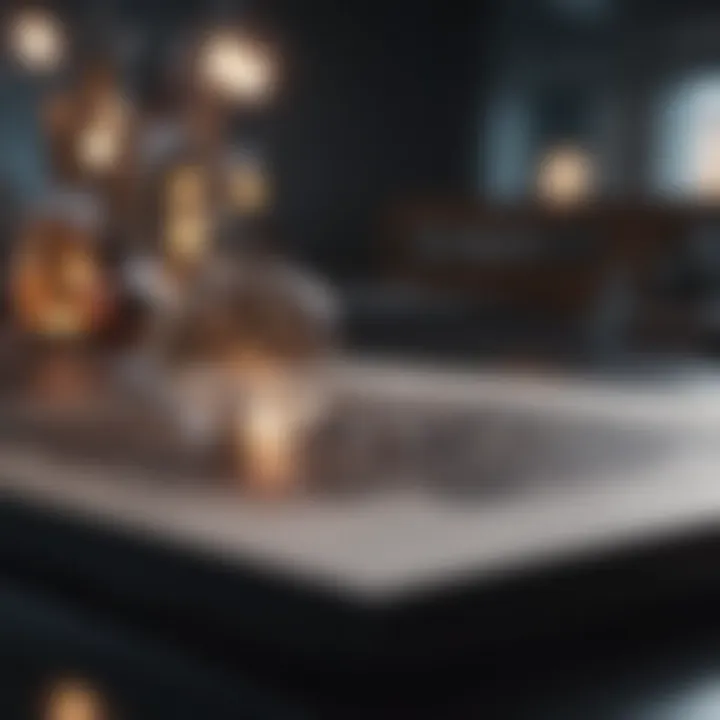
Tools for Quality Assessment
Several tools exist to aid researchers in evaluating the quality of studies included in a meta-analysis. These tools can vary depending on the type of research under scrutiny. Common tools include:
- Cochrane Risk of Bias Tool: Primarily for randomized controlled trials, this tool assesses potential biases related to randomization, blinding, and reporting.
- Newcastle-Ottawa Scale: Used for assessing the quality of non-randomized studies, it focuses on selection, comparability, and exposure/outcomes.
- AMSTAR: This tool specifically evaluates the methodological quality of systematic reviews.
These tools provide a structured approach, guiding researchers through critical aspects of study design that can influence the results.
Grading the Evidence
Grading the evidence is another essential aspect of quality assessment in meta-analysis. It helps categorize the level of confidence one can have in the synthesized findings. Various frameworks are utilized for grading evidence, with two prominent systems being GRADE (Grading of Recommendations Assessment, Development and Evaluation) and the Evidence-Based Medicine (EBM) pyramid.
- GRADE: This framework assesses quality based on factors such as study design, risk of bias, inconsistency, indirectness, imprecision, and publication bias. The outcomes can either be rated as high, moderate, low, or very low.
- EBM Pyramid: This method ranks the strength of evidence from various types of research, with systematic reviews and meta-analyses at the top, followed by randomized controlled trials, cohort studies, and expert opinions.
By grading the evidence, researchers can communicate the reliability of their findings to practitioners effectively. This aids in making informed decisions based on robust data synthesis.
Proper quality assessment and grading prevent the interpretation of erroneous conclusions, enhancing the overall integrity of the research.
Future Directions in Meta-Analysis
Meta-analysis is a dynamic field and its future directions are crucial for continued advancements in research synthesis. As more studies become available across various disciplines, the methodologies and applications of meta-analysis must evolve. This evolution is shaped by emerging technologies, new methodologies, and an increasing need for interdisciplinary collaboration. Understanding these future directions can enhance the credibility and utility of meta-analyses in informing both theory and practice.
Integration of Machine Learning
Machine learning is rapidly transforming how researchers handle data across fields. The integration of machine learning into meta-analysis introduces advanced algorithms that can better manage large datasets and uncover hidden patterns. Traditional meta-analysis typically relies on established statistical methods, which can sometimes miss complex interdependencies among variables. With machine learning, researchers can apply predictive modeling, cluster analysis, and natural language processing to extract richer insights from existing studies.
Several machine learning techniques can augment traditional meta-analysis:
- Predictive Analytics: Helps in forecasting outcomes based on historical data, thus aiding in hypothesis generation.
- Feature Selection Algorithms: Identify the most relevant variables from vast datasets, improving the focus of meta-analyses.
- Neural Networks: Can model intricate relationships within the data that traditional methods may overlook.
However, it is vital for researchers to ensure transparency in these models. As machine learning techniques can sometimes be seen as "black boxes," clear documentation and reporting of methods are necessary to foster trust and reproducibility.
Increasing Interdisciplinary Collaboration
As research problems become more complex, the need for interdisciplinary collaboration in meta-analysis is becoming evident. No single discipline holds all the answers. Collaborations between fields such as healthcare, technology, social sciences, and environmental studies can lead to more comprehensive understandings of intricate issues, allowing for more nuanced interpretations of data.
The benefits of interdisciplinary work in meta-analysis include:
- Broader Perspectives: Input from diverse fields can reshape research questions and methodologies, leading to innovative approaches.
- Enhanced Rigor: Combining different methodological frameworks can strengthen the validity of findings, reducing biases inherent to one discipline.
- Resource Sharing: Collaborative efforts can optimize resource use, accessing shared data repositories and expert knowledge across fields.
Effective collaboration requires communication strategies that bridge the gap between varied terminologies and theoretical frameworks. Training and workshops can be valuable in aligning interdisciplinary teams, ensuring that knowledge is exchanged efficiently.
In summary, the future of meta-analysis lies at the intersection of technology and interdisciplinary collaborations. Embracing these directions can not only enhance research efficacy but also expand the impact of meta-analyses in forming evidence-based policy and practice.
Ending
In the context of this article, the conclusion serves as a crucial component, encapsulating the essence of meta-analysis and its broader implications in research. It synthesizes the information provided, reaffirming the importance of meta-analytic methodologies in enhancing the scientific landscape. With a comprehensive understanding of the topic, readers can appreciate the substantial benefits of meta-analysis in deriving insights from diverse studies.
Concluding this article, the following key elements stand out:
- Importance of Evidence Synthesis: Meta-analysis aggregates findings from multiple studies, offering a more robust conclusion than singular studies can provide. This synthesis is essential in fields where research results can vary widely.
- Statistical Power: By combining data, meta-analysis increases the ability to detect effects and relationships, even in scenarios where individual studies may lack sufficient power.
- Guidance for Practice: The insights drawn from meta-analytic studies inform evidence-based practices, aiding researchers and practitioners in making data-driven decisions.
- Identifying Knowledge Gaps: The summary of a meta-analysis can reveal areas where research is lacking, guiding future inquiries.
Critically, the conclusion encourages further inquiry into these methodologies. It raises awareness about the challenges faced in conducting meta-analyses, such as publication bias and study quality, which researchers must navigate constantly.
Summary of Key Points
In this article, several key themes emerged regarding meta-analysis:
- Definition and overview of meta-analysis, highlighting its methodology and historical context.
- The various types of meta-analyses, including fixed-effect, random-effects, and network meta-analysis.
- Importance in enhancing statistical power and identifying patterns across studies.
- Applications spanning clinical research, environmental studies, and social sciences.
- Challenges associated with conducting thorough and reliable meta-analyses.
- Importance of quality assessment tools and grading evidence.
- Future directions focusing on machine learning and interdisciplinary collaboration.
"Meta-analysis is not merely a tool; it is an essential framework for understanding complex questions across disciplines."
The Future of Evidence Synthesis
The trajectory of meta-analysis points towards an increasingly integral role in scientific inquiry. As data availability continues to expand, the need for sophisticated synthesis methods becomes paramount. Future advancements will likely include:
- Integration of Machine Learning: Leveraging algorithms can enhance data processing and lead to more robust findings.
- Interdisciplinary Collaboration: Teaming up across various fields will promote richer analyses and broader applicability of meta-analytic results.
As the research environment evolves, it is crucial for scholars, educators, and practitioners to stay informed about the developments within meta-analysis. Embracing these changes will not only refine their methods but also contribute to the integrity and richness of scientific knowledge.