Understanding Population Health Risk Stratification
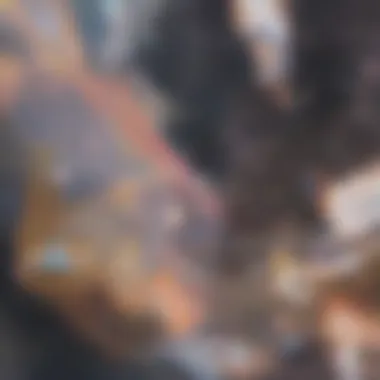
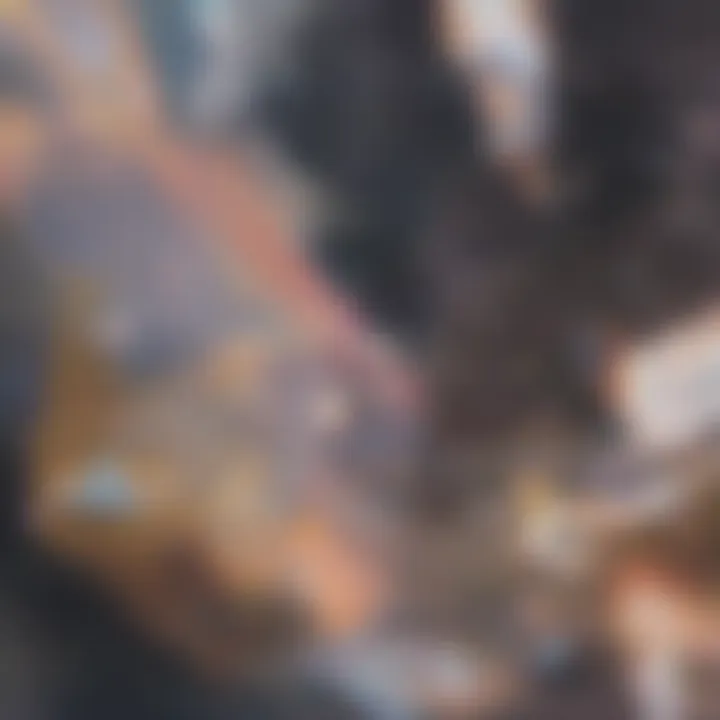
Intro
As healthcare systems face mounting pressures including rising costs, aging populations, and a growing burden of chronic diseases, the need for effective risk stratification becomes increasingly critical. Understanding the risk profiles of different groups can lead to more tailored interventions, ultimately contributing to improved health outcomes.
Definition and Importance
The importance of this approach extends beyond mere categorization. It promotes proactive healthcare management, shifting the emphasis from reactive treatments to preventive measures. For instance, patients identified as high risk for chronic diseases like diabetes can receive targeted education, lifestyle counseling, and monitoring, aiming to improve their health trajectory and reduce overall healthcare costs. Importantly, this focus on prevention can also lead to better population health outcomes, illustrating how risk stratification fundamentally reshapes health delivery.
Historical Context
In the 1980s and 1990s, managed care organizations began to develop systems that identified at-risk populations to provide more comprehensive care. As more healthcare providers recognized the potential benefits, risk stratification methodologies became widely adopted. The advent of technology allowed for the integration of electronic health records, enabling finer-grained data analysis and a sophisticated understanding of population health dynamics. Over time, this evolution set the stage for the current landscape, where risk stratification has become a cornerstone of public health initiatives and healthcare policy.
Fundamental Concepts
Key Theories in Population Health
Key theories in population health form the backbone of risk stratification practices. One prominent theory is the population health model, which emphasizes health outcomes across groups rather than individual patients. This model encourages healthcare providers to consider social and environmental factors affecting health. It balances between clinical risk factors and broader determinants of health. Moreover, concepts like the epidemiologic triad, focusing on agent, host, and environment interactions, help in understanding disease dynamics.
An understanding of these theories aids in the identification of at-risk populations. This knowledge informs targeted interventions which can lead to improved health outcomes. Presenting healthcare in this comprehensive manner considers various influences and provides a robust framework for planning and policy-making. It is imperative to note that these theories are not static. They evolve based on new research, requiring practitioners to continuously update their knowledge base.
Social Determinants of Health
Research indicates that nearly 80% of health outcomes are determined by social and environmental factors rather than clinical care. This underscores the importance of integrating social determinant data into health risk assessments. Providers can prioritize resources effectively when they understand the context of the populations they serve.
In practical terms, healthcare systems must consider how these social factors intersect with traditional medical data. For example, understanding a patient's living situation can provide valuable insights into their health risks. By doing so, healthcare can shift towards a more holistic and equitable approach.
"Recognizing social determinants is essential for effective risk stratification and improving health outcomes."
Methodologies for Risk Stratification
Understanding the methodologies for risk stratification is crucial for improving population health outcomes. These methodologies help in identifying high-risk individuals or groups within populations, guiding healthcare providers to allocate resources more efficiently. By strategically targeting interventions, healthcare systems can enhance care quality and reduce costs associated with unmanaged health risks. This structured approach supports evidence-based public health policies and fosters better health outcomes across diverse populations.
Data Collection Techniques
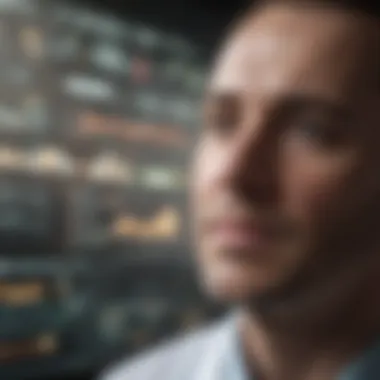
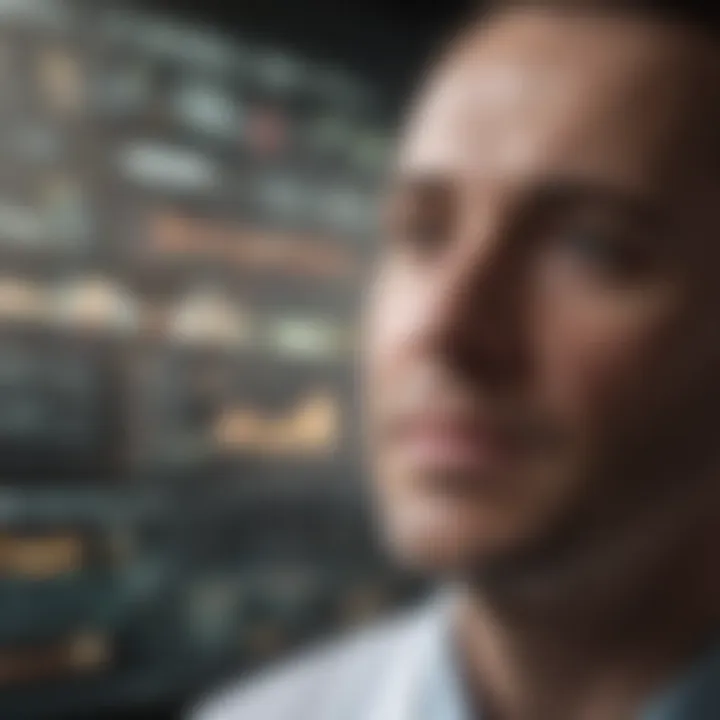
Data collection is the foundation of effective risk stratification. Various techniques can be employed to gather relevant information. Primary data collection methods include surveys, interviews, and focus groups, while secondary methods rely on existing datasets from hospitals or health organizations. For example, electronic health records (EHRs) serve as a rich source of health information, enabling researchers to analyze patient demographics, medical histories, and treatment responses.
- Surveys: Directly engaging with the community can reveal valuable insights. Questions should be designed to capture healthcare access, behavioral patterns, and social factors.
- Electronic Health Records: These digital tools streamline access to patient information and clinical data. They help in identifying at-risk populations based on health history.
- Claims Data: Insurance claims can highlight patterns in patient care usage, helping to identify frequent users of healthcare services who may require targeted interventions.
Implementing robust data collection methods ensures that healthcare providers base their strategies on accurate and comprehensive information, ultimately leading to better risk stratification results.
Analytical Frameworks
After data collection, analytical frameworks come into play to process and interpret the information. These frameworks facilitate the transformation of raw data into actionable insights. Some effective analytical approaches include cohort studies, regression analysis, and risk scoring systems. Cohort studies, for instance, can help track health outcomes over time in specific population segments, while regression analysis connects various risk factors to health outcomes.
- Cohort Studies: These observational studies can help identify relationships between risk factors and health outcomes. Longitudinal data enhances our understanding of changes in health status over time.
- Regression Analysis: By examining the effect of independent variables on dependent health outcomes, this method helps quantify individual risk levels.
- Risk Scoring Systems: Algorithms can be created to assign scores based on various risk factors. These scores categorize individuals into different risk levels, guiding interventions more effectively.
These analytical approaches allow healthcare professionals to extract meaningful patterns and correlations, creating a more nuanced view of population health risks.
Machine Learning Applications
The application of machine learning in health risk stratification has gained significant traction. By employing algorithms, machine learning can analyze vast datasets to identify patterns that may not be readily apparent through traditional methods. These applications optimize predictive modeling and automate risk assessments.
- Predictive Modeling: By training models on historical data, machine learning algorithms can forecast potential health outcomes for individuals, enabling proactive interventions.
- Automated Decision Support: Machine learning systems can assist healthcare providers in decision-making by delivering insights on potential risk factors relevant to patient care.
- Natural Language Processing (NLP): This subset of machine learning can analyze unstructured data, such as clinical notes, to uncover hidden risks based on physician documentation.
Machine learning is not just a tool; it becomes an integral part of how we rethink risk assessment in healthcare.
Incorporating machine learning in risk stratification can improve accuracy and streamline workflows, ultimately enhancing patient care.
Understanding these methodologies is critical for healthcare professionals who aim to implement effective population health strategies. Each technique's strengths contribute uniquely to the greater goal of optimizing healthcare delivery and improving health outcomes.
Implications for Public Health Policy
One major benefit of incorporating risk stratification into policy is the prioritization of resources. Policymakers can allocate funds and services where they are most needed, which systematically improves the overall health of communities. For example, vulnerable groups—those identified as having a higher probability of adverse health outcomes—can receive tailored, proactive interventions. This ultimately lowers healthcare costs and improves patient outcomes in the long run.
Another key consideration is the emphasis on preventive health measures. By identifying at-risk populations, policymakers can focus on preventive strategies that address potential health issues before they escalate. This proactive approach allows for better management of chronic illnesses and reduces the burden on healthcare systems.
"Effective public health policy employs risk stratification as a foundation for informed decision-making that fosters community health resilience."
Resource Allocation Strategies
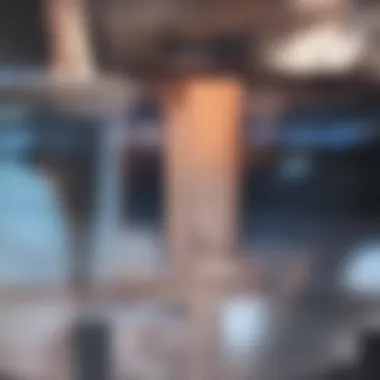
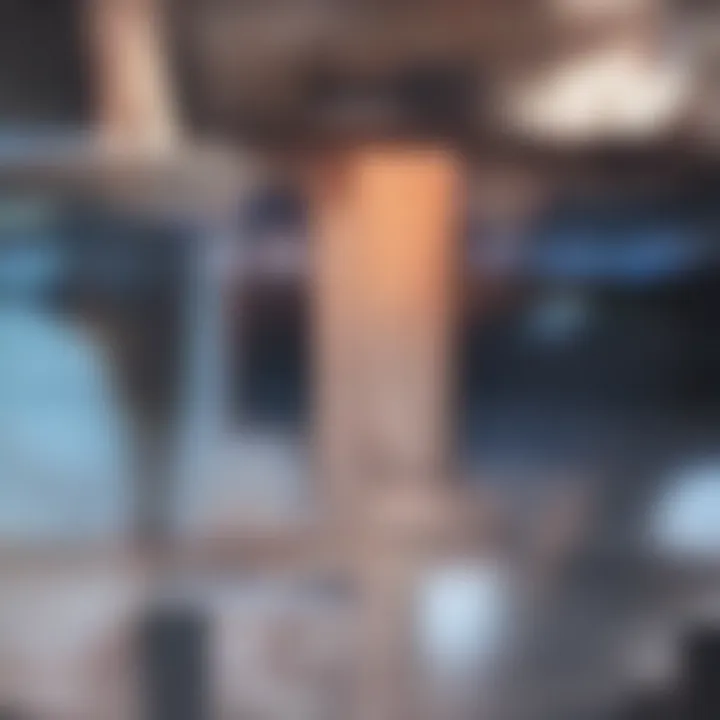
Healthcare funding can be directed to programs and services that address the specific needs of high-risk groups. This targeted approach avoids wastage of resources and ensures that interventions effectively meet community needs. Implementing public health campaigns that raise awareness about specific risk factors among identified populations can also maximize the usability of resources.
Furthermore, the allocation of human resources, such as healthcare professionals, can be optimized. For instance, placing more nurses or social workers in communities identified as high-risk allows for closer monitoring and quicker responses to emerging health issues.
Preventive Health Measures
Preventive health measures are essential outcomes of effective risk stratification. By identifying individuals who are at higher risk for specific conditions, public health officials can implement interventions that prevent those conditions from developing.
These measures can take various forms, such as vaccination programs, routine screenings, and health education initiatives. For example, a community identified as having a high prevalence of diabetes can benefit from educational programs focused on nutrition, exercise, and regular health check-ups. Likewise, mental health initiatives targeting at-risk populations can significantly improve community resilience.
Moreover, preventive measures aligned with risk stratification are also cost-effective. By reducing the incidence of diseases through prevention, healthcare systems can experience lower hospitalization rates and reduced strain on emergency services—all leading to healthier populations that require fewer resources over time.
Case Studies in Risk Stratification
Case studies in risk stratification play a crucial role in understanding practical applications and real-world outcomes of population health strategies. They serve as evidential support for theories and methodologies discussed in the broader context of health risk stratification. By delving into specific examples, we can identify both the successes and challenges inherent in implementing these strategies.
The insights gleaned from case studies help in refining future implementations and shaping public health policy. Evaluating these examples can uncover effective tactics that lead to improved health outcomes, increased patient care, and better resource management.
Successful Implementations
One notable example of successful risk stratification is found in the deployment of chronic disease management programs. The Chronic Care Model implemented at Kaiser Permanente showcases how effective stratification can enhance patient engagement and health outcomes. In this model, patients with chronic diseases such as diabetes or heart conditions are systematically identified and monitored.
This system uses data analytics to evaluate patient information, thereby categorizing individuals based on their risk levels. The organization then allocates resources to those at high risk, offering them personalized support, education, and intervention plans. Results have shown reduced emergency room visits and better control of chronic conditions, underscoring the model's efficacy.
Another compelling case can be seen in the Accountable Care Organizations (ACOs). ACOs function on the principle of coordinated care. They analyze patient data to stratify health risks among populations, allowing for preventive measures tailored to specific groups. In an ACO initiative in Massachusetts, stratification led to significant decreases in hospital admissions. By proactively addressing the unique health needs of patients, ACOs not only enhance care quality but also decrease overall healthcare costs.
Lessons Learned from Failures
Despite the successes, several implementations of risk stratification have faced substantial challenges, leading to less than favorable outcomes. One key area is the integration of data systems. In an instance involving a large hospital network in California, different departments utilized incompatible data systems. The failure to aggregate and analyze unified data led to fragmented care delivery and inaccurate risk assessments. This project exemplified how essential interoperability is for effective implementation.
Misinterpretation of risk factors is another common pitfall. In one regrettable case, a healthcare organization in Texas over-reliant on algorithmic models failed to recognize social determinants of health in their stratification process. This oversight resulted in misallocation of resources. Individuals with high social needs but lower clinical risk were overlooked, highlighting that machine learning tools must be complemented by clinical judgment and contextual understanding.
Challenges in Implementation
Data Privacy and Security Concerns
One of the foremost challenges in implementing risk stratification is ensuring data privacy and security. With health data collection growing rapidly, safeguarding patient information has become paramount. Organizations must comply with strict regulations such as the Health Insurance Portability and Accountability Act (HIPAA) in the United States. Any misstep in data handling can lead to severe legal repercussions and loss of public trust.
Healthcare providers must implement robust data management systems. This includes adopting encryption techniques, conducting regular audits, and training personnel on data handling protocols. Additionally, involving patients in the process can enhance transparency and trust. When patients understand how their data will be used and stored, it alleviates some concerns regarding privacy.
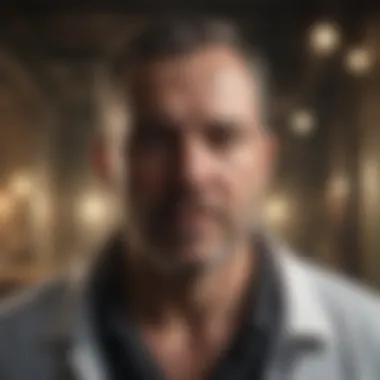
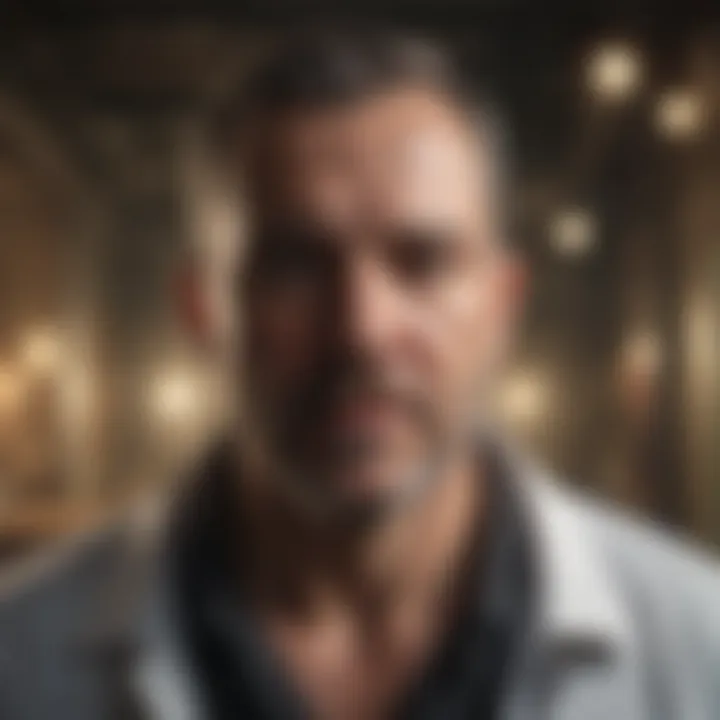
"Data privacy is not just a requirement; it embodies the trust that individuals place in healthcare systems."
Interoperability Issues
Another significant challenge relates to interoperability. In a fragmented healthcare landscape, different organizations often use varied systems and data formats. This lack of standardization impedes the exchange of health information. Consequently, it affects the ability to stratify populations effectively. If data cannot be accessed or understood across platforms, the analysis becomes inefficient and ultimately less effective.
To address this, stakeholders must advocate for standardized data formats. This can improve compatibility among systems. Governments and organizations can play an instrumental role in promoting interoperability through policies that foster collaboration between technology vendors. The goal should be to create a seamless flow of information among all relevant stakeholders.
Stakeholder Engagement
Effective implementation of risk stratification frameworks requires active engagement from all stakeholders involved. These include healthcare providers, patients, policymakers, and IT professionals. Failing to include diverse voices can lead to gaps in understanding and misalignment of goals.
Engaging stakeholders at the outset strengthens the implementation process. Gathering input from those affected by the stratification allows for a better understanding of community needs. It is essential to facilitate open discussions to identify expectations and potential hurdles.
Emerging Technologies
- Telemedicine allows for remote consultations and monitoring, expanding access to care especially in underserved areas.
- Predictive analytics uses historical data to forecast future health risks, enabling healthcare providers to implement timely interventions.
- Blockchain technology can ensure data security and interoperability, fostering trust among consumers regarding their health information.
- Natural language processing can analyze unstructured data from medical records, enhancing diagnostic precision.
Overall, these technologies not only enhance risk stratification processes but also pave the way for personalized healthcare solutions. Such transformation is vital for addressing chronic diseases and improving preventive care.
Evolving Healthcare Models
- Accountable Care Organizations (ACOs) are one example, where providers are collectively responsible for patient outcomes and costs. This collaboration encourages proactive patient engagement and care coordination.
- Patient-Centered Medical Homes (PCMHs) focus on providing comprehensive primary care, ensuring patients receive all necessary services in a coordinated manner.
- The integration of behavioral and physical health services allows for a holistic approach, where mental health is considered alongside physical health, recognizing their interconnectedness.
These models underline the necessity of careful risk stratification as they require comprehensive data to tailor interventions that are effective and equitable. Furthermore, educating stakeholders on these models will foster a better understanding of their significance in improving community health outcomes and reducing health disparities.
"Investing in emerging technologies and evolving models can shift the focus from reactive to proactive healthcare, which is critical for achieving better health outcomes."
End
The article highlights key benefits derived from effective risk stratification such as improved patient outcomes, cost reduction in healthcare systems, and enhanced focus on preventive health strategies. It considers factors such as accuracy in data gathering and the role of analytical tools in shaping policies that directly address health disparities.
As we synthesize key insights from earlier sections, it becomes clear that addressing challenges like data privacy, stakeholder engagement, and interoperability is essential for effective implementation.
Summary of Key Insights
- Importance of Data: Accurate data collection is critical for effective risk stratification. It informs the development of analytical models that predict health risks and outcomes.
- Intervention Targeting: Stratified populations allow healthcare workers to design targeted interventions, addressing unique needs rather than applying one-size-fits-all solutions.
- Cost Efficiency: By focusing on high-risk populations, healthcare systems can optimize resource allocation and reduce overall costs associated with preventable diseases.
- Policy Impact: Insights from stratification can directly influence public health policies, enhancing their effectiveness in addressing social determinants of health.
Final Thoughts
In closing, those involved in healthcare—from administrators to practitioners—must remain informed about advancements in risk stratification. Such knowledge will aid not just in improving individual patient care but also in uplifting entire communities by addressing systemic issues that affect health.